Joint Optimization of Jamming Link and Power Control in Communication Countermeasures: A Multiagent Deep Reinforcement Learning Approach
WIRELESS COMMUNICATIONS & MOBILE COMPUTING(2022)
摘要
Due to the nonconvexity feature of optimal controlling such as jamming link selection and jamming power allocation issues, obtaining the optimal resource allocation strategy in communication countermeasures scenarios is challenging. Thus, we propose a novel decentralized jamming resource allocation algorithm based on multiagent deep reinforcement learning (MADRL) to improve the efficiency of jamming resource allocation in battlefield communication countermeasures. We first model the communication jamming resource allocation problem as a fully cooperative multiagent task, considering the cooperative interrelationship of jamming equipment (JE). Then, to alleviate the nonstationarity feature and high decision dimensions in the multiagent system, we introduce a centralized training with decentralized execution framework (CTDE), which means all JEs are trained with global information and rely on their local observations only while making decisions. Each JE obtains a decentralized policy after the training process. Subsequently, we develop the multiagent soft actor-critic (MASAC) algorithm to enhance the exploration capability of agents and accelerate the learning of cooperative policies among agents by leveraging the maximum policy entropy criterion. Finally, the simulation results are presented to demonstrate that the proposed MASAC algorithm outperforms the existing centralized allocation benchmark algorithms.
更多查看译文
关键词
deep reinforcement learning,reinforcement learning,jamming link,communication countermeasures
AI 理解论文
溯源树
样例
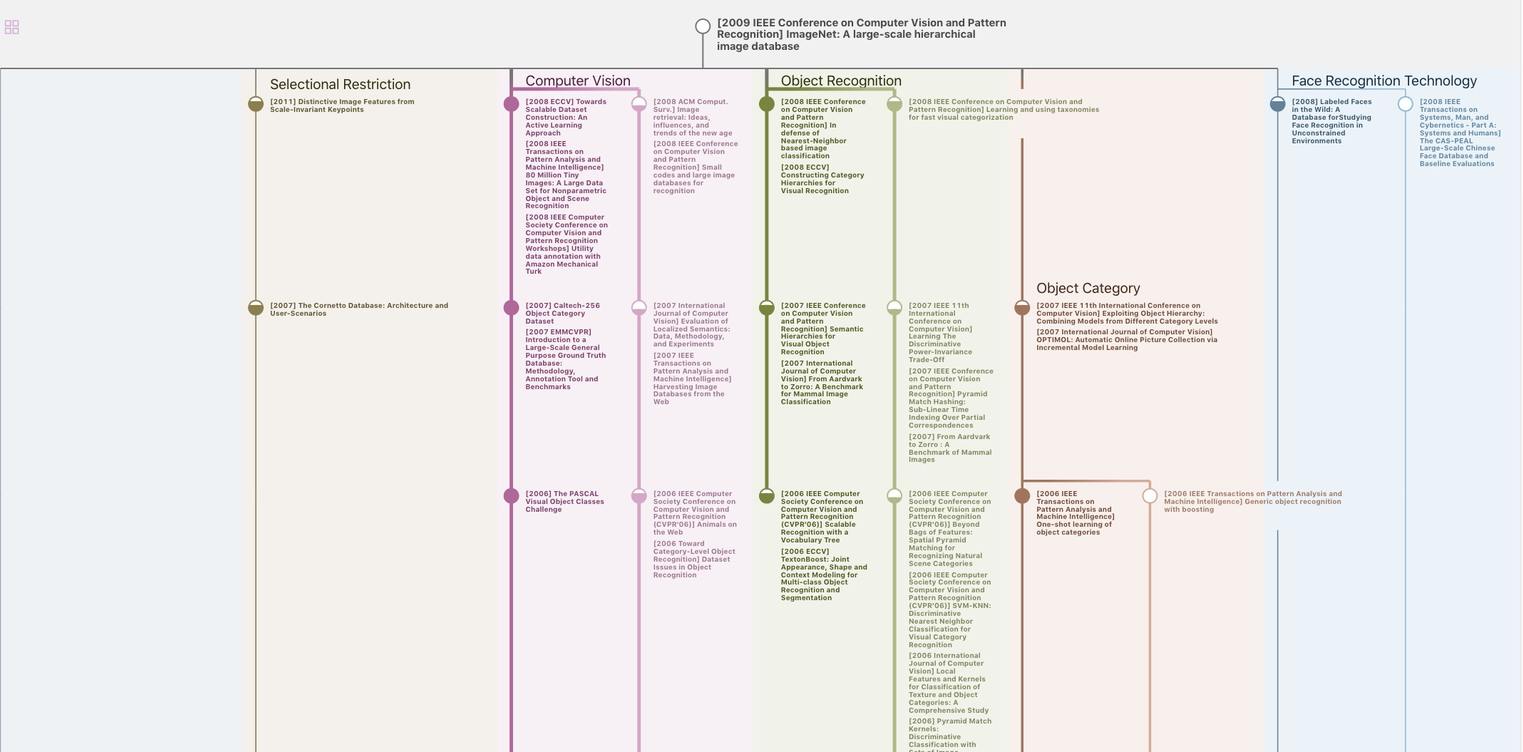
生成溯源树,研究论文发展脉络
Chat Paper
正在生成论文摘要