Multi-view subspace enhanced representation of manifold regularization and low-rank tensor constraint
INTERNATIONAL JOURNAL OF MACHINE LEARNING AND CYBERNETICS(2022)
摘要
In this paper, to extract the manifold information from multi-view data and enhance the clustering performance of a multi-view learning method, the multi-view subspace enhanced representation of manifold regularization and low-rank tensor constraint (MSERMLRT) method is introduced. Our model uses a tensor to explore the correlation between views. The tensor is constrained with a low-rank, and the purpose of such processing is to reduce the redundant information of the learned subspace representation. This model also uses the manifold information from multi-view data and imposes a sparse constraint on the product of itself and the transpose of the subspace representation matrix to enhance the diagonal block structure of the subspace representation, thereby improving its clustering effect to a certain extent. We also designed a helpful method for solving the MSERMLRT model and analyzed the convergence of our approach both theoretically and experimentally. The clustering performance on certain challenging datasets indicate that the MSERMLRT model is superior to many other advanced multi-view clustering methods.
更多查看译文
关键词
Manifold regularization,Low-rank,Tensor,Subspace enhanced representation,Sparse constraint
AI 理解论文
溯源树
样例
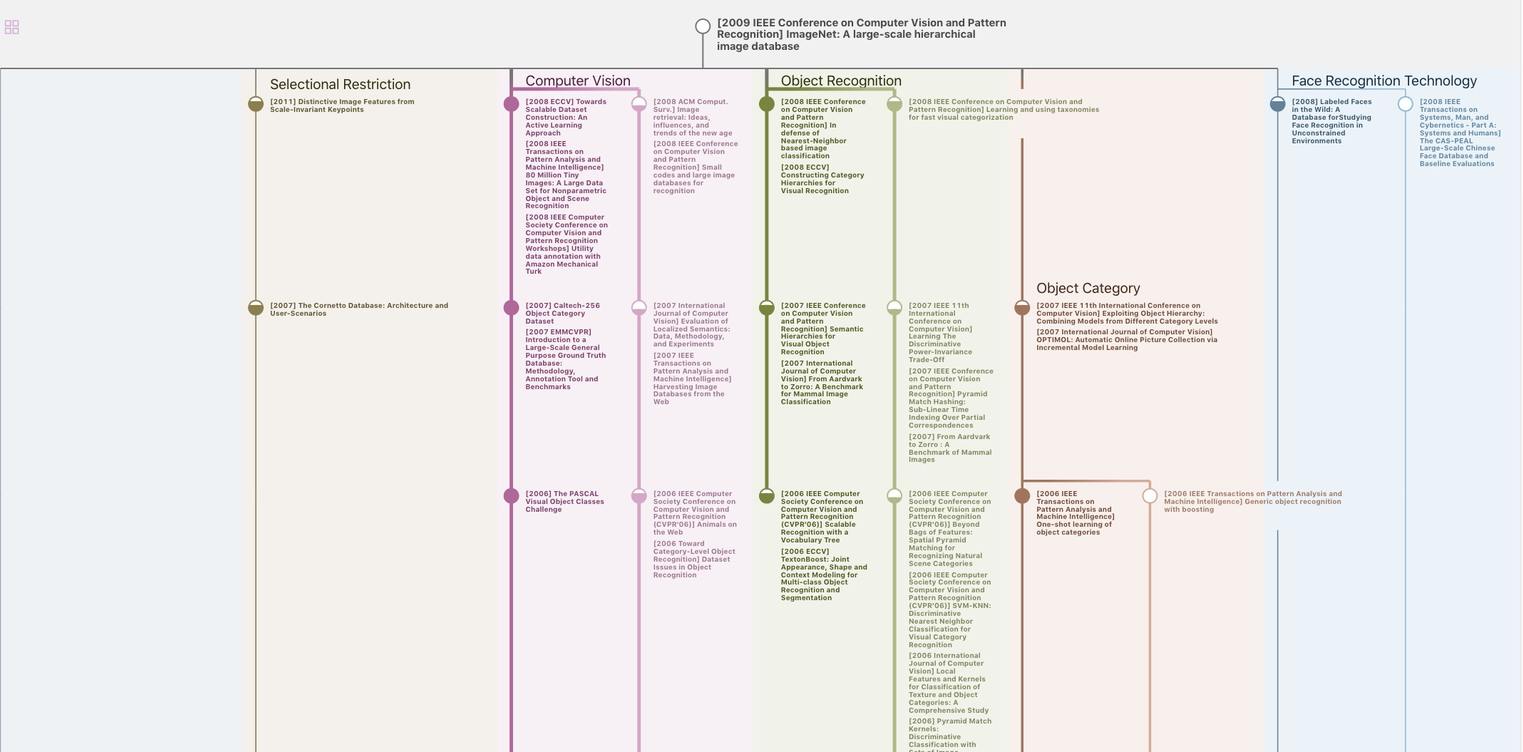
生成溯源树,研究论文发展脉络
Chat Paper
正在生成论文摘要