Image Fundus Classification System for Diabetic Retinopathy Stage Detection Using Hybrid CNN-DELM
BIG DATA AND COGNITIVE COMPUTING(2022)
摘要
Diabetic retinopathy is the leading cause of blindness suffered by working-age adults. The increase in the population diagnosed with DR can be prevented by screening and early treatment of eye damage. This screening process can be conducted by utilizing deep learning techniques. In this study, the detection of DR severity was carried out using the hybrid CNN-DELM method (CDELM). The CNN architectures used were ResNet-18, ResNet-50, ResNet-101, GoogleNet, and DenseNet. The learning outcome features were further classified using the DELM algorithm. The comparison of CNN architecture aimed to find the best CNN architecture for fundus image features extraction. This research also compared the effect of using the kernel function on the performance of DELM in fundus image classification. All experiments using CDELM showed maximum results, with an accuracy of 100% in the DRIVE data and the two-class MESSIDOR data. Meanwhile, the best results obtained in the MESSIDOR 4 class data reached 98.20%. The advantage of the DELM method compared to the conventional CNN method is that the training time duration is much shorter. CNN takes an average of 30 min for training, while the CDELM method takes only an average of 2.5 min. Based on the value of accuracy and duration of training time, the CDELM method had better performance than the conventional CNN method.
更多查看译文
关键词
diabetic retinopathy,CNN architecture,feature learning,DELM classification
AI 理解论文
溯源树
样例
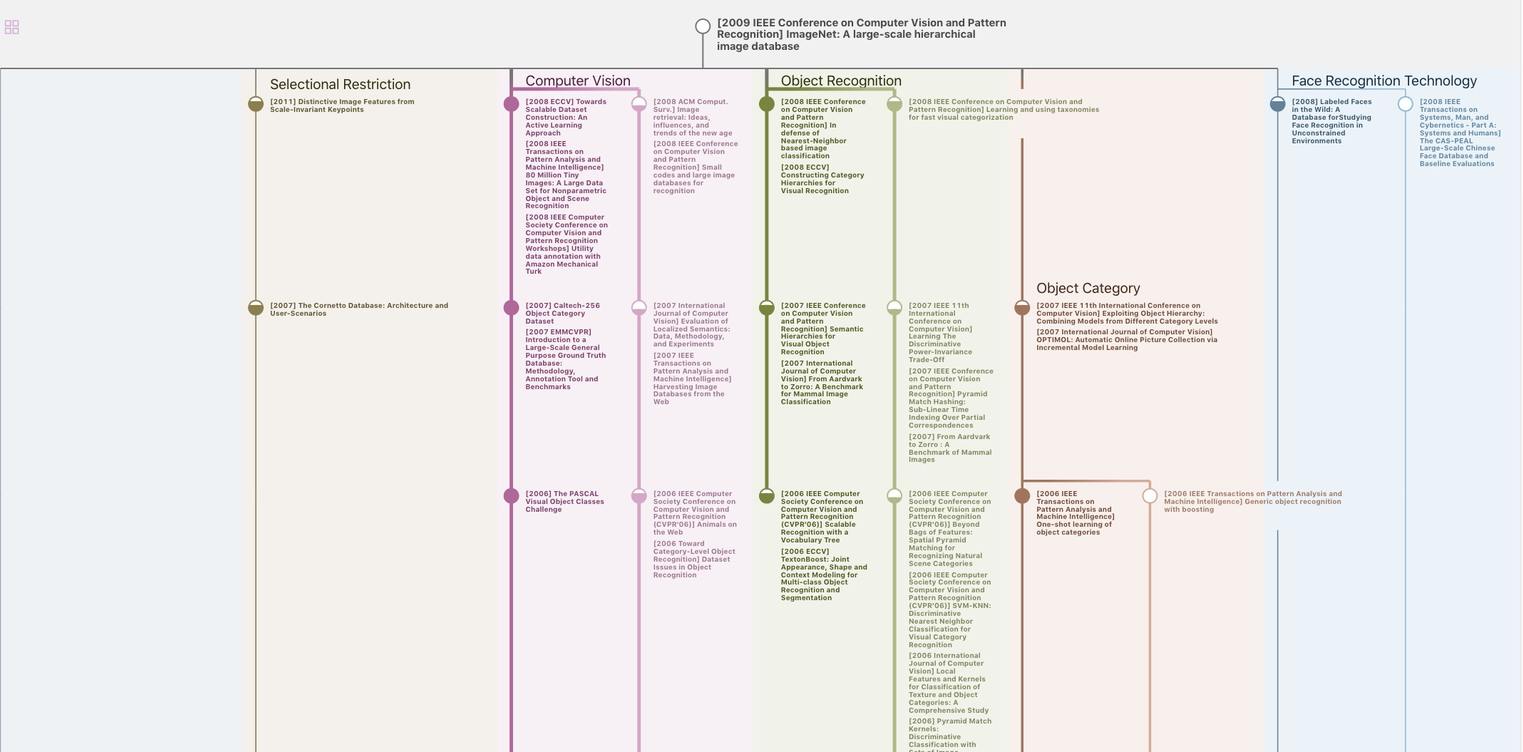
生成溯源树,研究论文发展脉络
Chat Paper
正在生成论文摘要