Modeling adaptive platoon and reservation-based intersection control for connected and autonomous vehicles employing deep reinforcement learning
COMPUTER-AIDED CIVIL AND INFRASTRUCTURE ENGINEERING(2023)
摘要
As a cutting-edge strategy to reduce travel delay and fuel consumption, platooning of connected and autonomous vehicles (CAVs) at signal-free intersections has become increasingly popular in academia. However, when determining optimal platoon size, few studies have attempted to comprehensively consider the relations between the size of a CAV platoon and traffic conditions around an intersection. To this end, this study develops an adaptive platoon-based autonomous intersection control model, named INTEL-PLT, which adopts deep reinforcement learning technique to realize the optimization of multiple dynamic objectives (e.g., efficiency, fairness, and energy saving). The framework of INTEL-PLT has a two-level structure: The first level employs a reservation-based policy integrated with a nonconflicting lane selection mechanism to determine the lanes' releasing priorities; and the second level uses a deep Q-network algorithm to identify the optimal platoon size based on real-time traffic conditions (e.g., traffic density, vehicle movement, etc.) of an intersection. The model is validated and examined on the simulator Simulation of Urban Mobility. It is found that the proposed model exhibits superior performances on both travel efficiency and fuel conservation as compared with state-of-the-art methods in three typical traffic conditions. Moreover, several in-depth insights learned from the simulations are provided in this paper, which could better explain the relation between platoon size and traffic condition.
更多查看译文
关键词
adaptive platoon,intersection control,autonomous vehicles
AI 理解论文
溯源树
样例
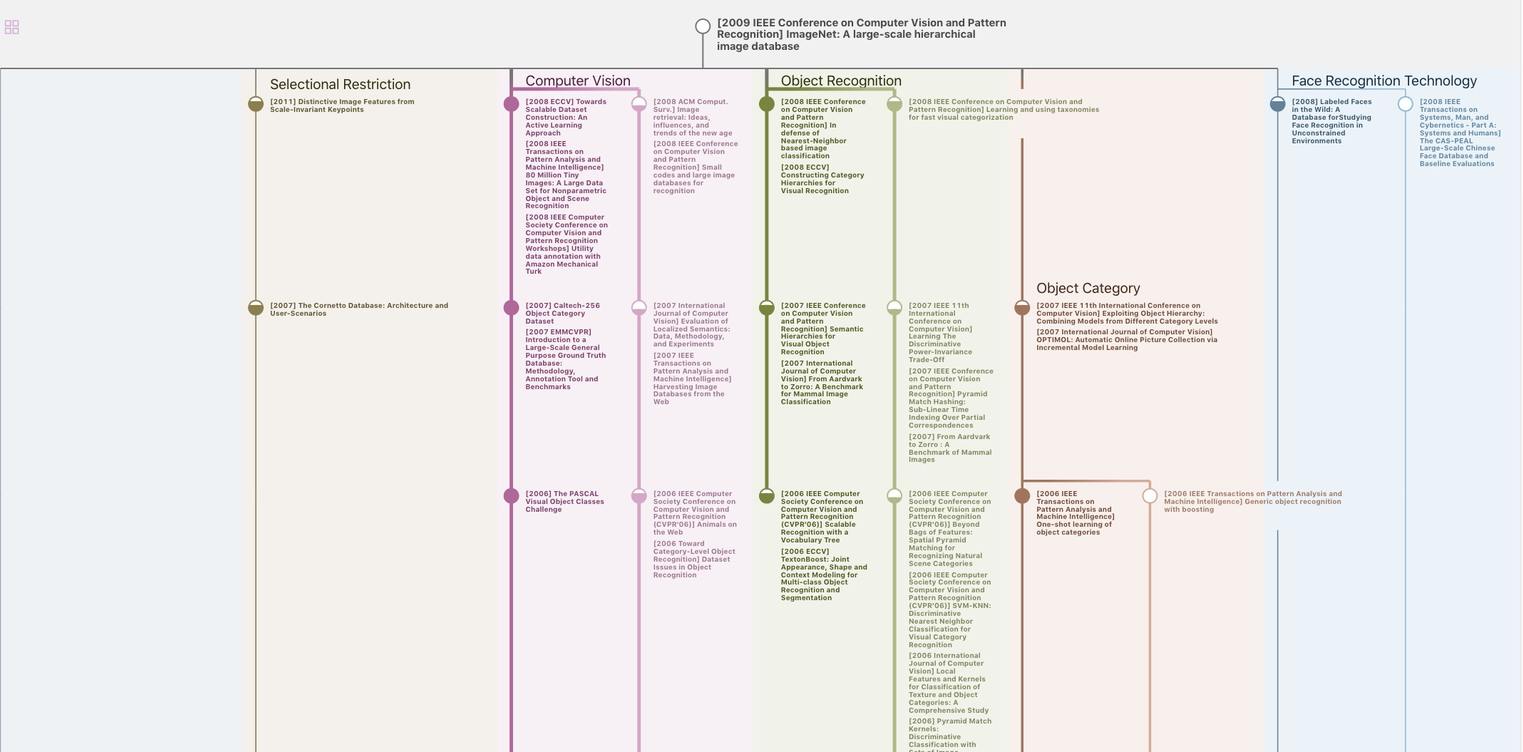
生成溯源树,研究论文发展脉络
Chat Paper
正在生成论文摘要