Dynamic IoT Malware Detection in Android Systems Using Profile Hidden Markov Models
APPLIED SCIENCES-BASEL(2023)
摘要
The prevalence of malware attacks that target IoT systems has raised an alarm and highlighted the need for efficient mechanisms to detect and defeat them. However, detecting malware is challenging, especially malware with new or unknown behaviors. The main problem is that malware can hide, so it cannot be detected easily. Furthermore, information about malware families is limited which restricts the amount of "big data" that is available for analysis. The motivation of this paper is two-fold. First, to introduce a new Profile Hidden Markov Model (PHMM) that can be used for both app analysis and classification in Android systems. Second, to dynamically identify suspicious calls while reducing infection risks of executed codes. We focused on Android systems, as they are more vulnerable than other IoT systems due to their ubiquitousness and sideloading features. The experimental results showed that the proposed Dynamic IoT malware Detection in Android Systems using PHMM (DIP) achieved superior performance when benchmarked against eight rival malware detection frameworks, showing up to 96.3% accuracy at 5% False Positive Rate (FP rate), 3% False Negative Rate (FN rate) and 94.9% F-measure.
更多查看译文
关键词
cybersecurity,Internet of Things,Markov Model,Android,malware detection
AI 理解论文
溯源树
样例
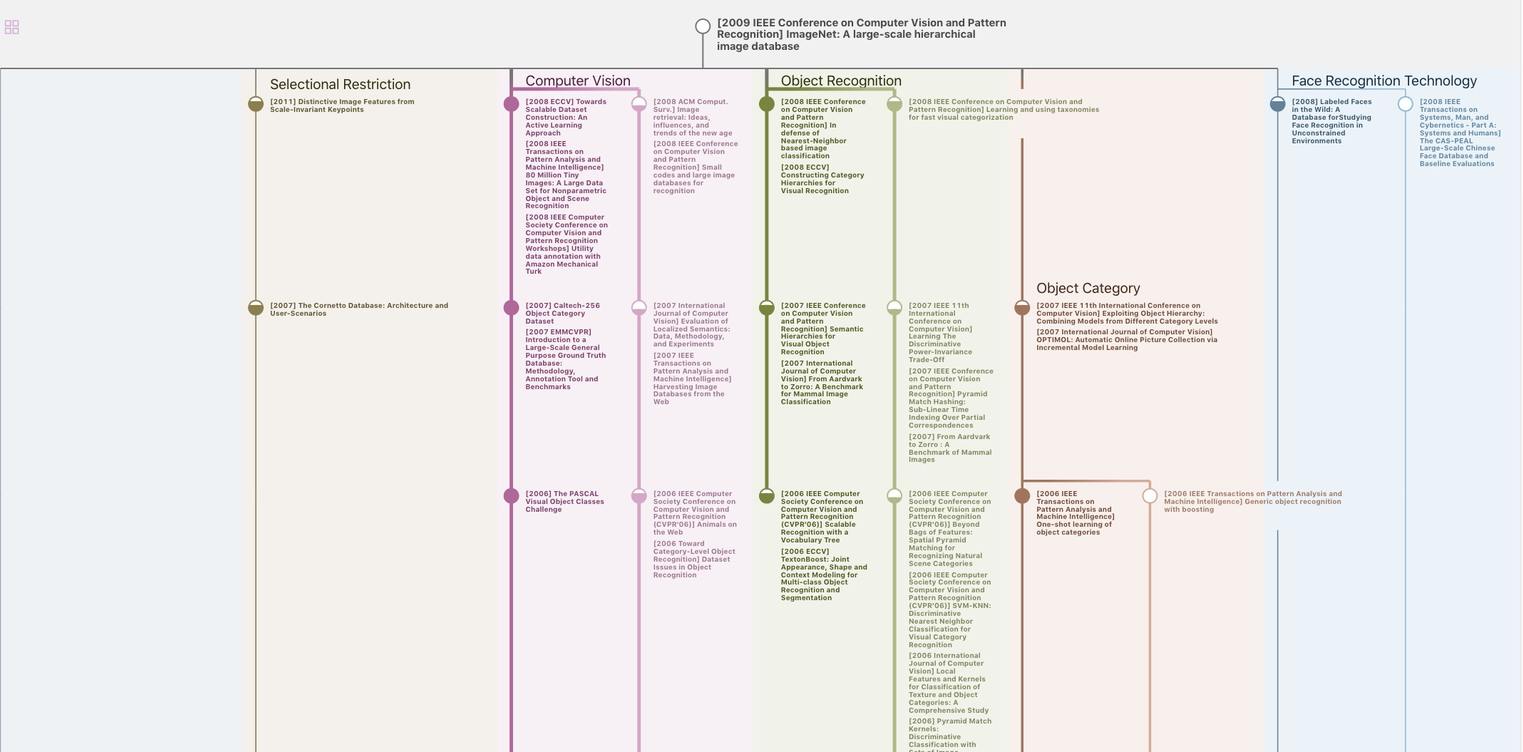
生成溯源树,研究论文发展脉络
Chat Paper
正在生成论文摘要