Accelerated Fuzzy C-Means Clustering Based on New Affinity Filtering and Membership Scaling
IEEE TRANSACTIONS ON KNOWLEDGE AND DATA ENGINEERING(2023)
摘要
Fuzzy C-Means (FCM) is a widely used clustering method. However, FCM and its many accelerated variants have low efficiency in the mid-to-late stage of the clustering process. In this stage, all samples are involved in updating their non-affinity centers, and the membership grades of most samples, whose assignments remain unchanged, are still updated by calculating the sample-center distances. All these factors lead to the algorithms converging slowly. In this paper, a new affinity filtering technique is developed to recognize a complete set of non-affinity centers for each sample with low computations. Then, a new membership scaling technique is suggested to set the membership grades between each sample and its non-affinity centers to 0 and maintain the fuzzy membership grades for others. By integrating these two techniques, FCM based on new affinity filtering and membership scaling (AMFCM) is proposed to accelerate the whole convergence process of FCM. Numerous experimental results performed on synthetic and real-world data sets have shown the feasibility and efficiency of the proposed algorithm. Compared with state-of-the-art algorithms, AMFCM is significantly faster and more effective. For example, AMFCM reduces the number of FCM iterations by 80% on average.
更多查看译文
关键词
Fuzzy C-means,affinity filtering,triangle inequality,non-affinity center,non-affinity sample,membership scaling
AI 理解论文
溯源树
样例
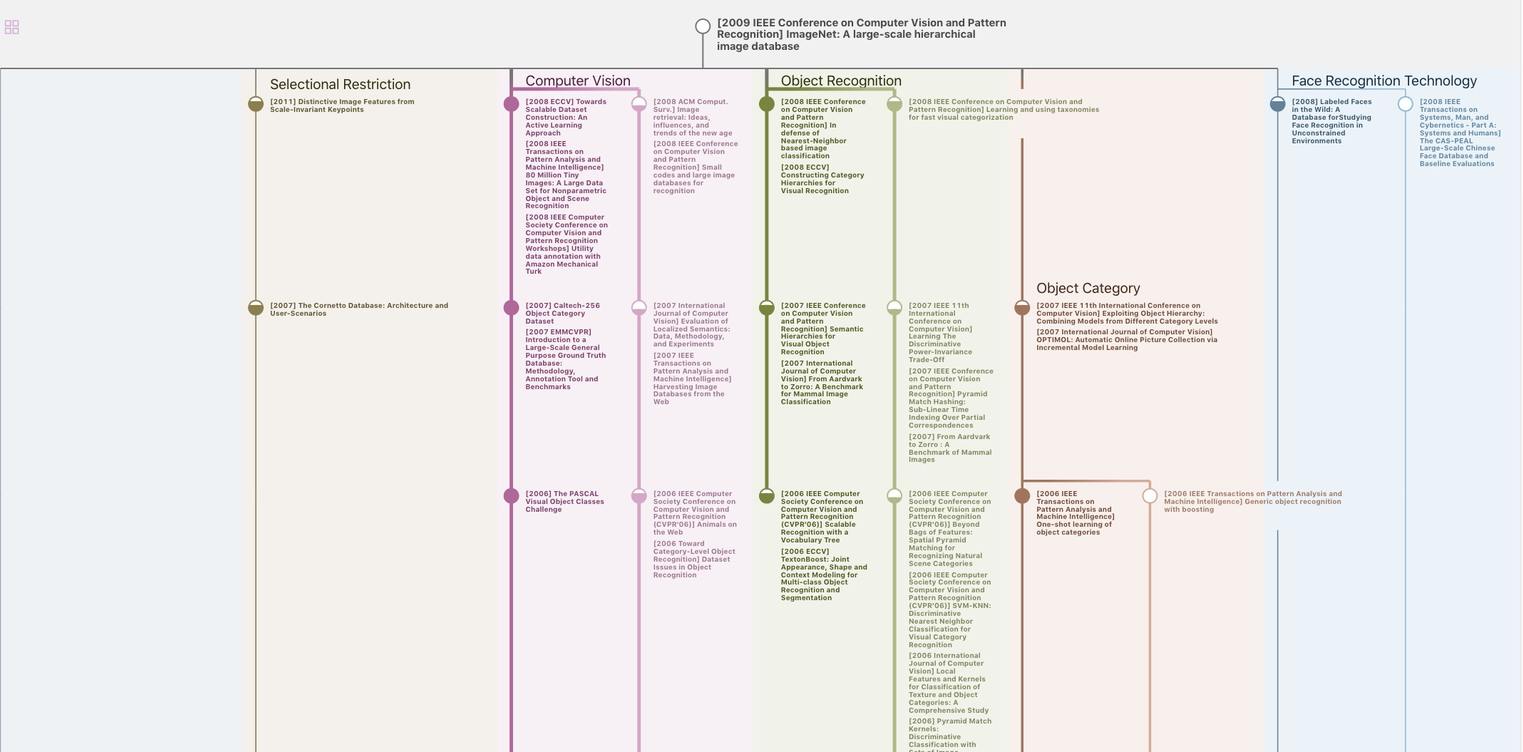
生成溯源树,研究论文发展脉络
Chat Paper
正在生成论文摘要