Do Neural Networks Generalize from Self-Averaging Sub-classifiers in the Same Way As Adaptive Boosting?
arXiv (Cornell University)(2023)
摘要
In recent years, neural networks (NNs) have made giant leaps in a wide variety of domains. NNs are often referred to as black box algorithms due to how little we can explain their empirical success. Our foundational research seeks to explain why neural networks generalize. A recent advancement derived a mutual information measure for explaining the performance of deep NNs through a sequence of increasingly complex functions. We show deep NNs learn a series of boosted classifiers whose generalization is popularly attributed to self-averaging over an increasing number of interpolating sub-classifiers. To our knowledge, we are the first authors to establish the connection between generalization in boosted classifiers and generalization in deep NNs. Our experimental evidence and theoretical analysis suggest NNs trained with dropout exhibit similar self-averaging behavior over interpolating sub-classifiers as cited in popular explanations for the post-interpolation generalization phenomenon in boosting.
更多查看译文
关键词
adaptive boosting,neural networks generalize,neural networks,self-averaging,sub-classifiers
AI 理解论文
溯源树
样例
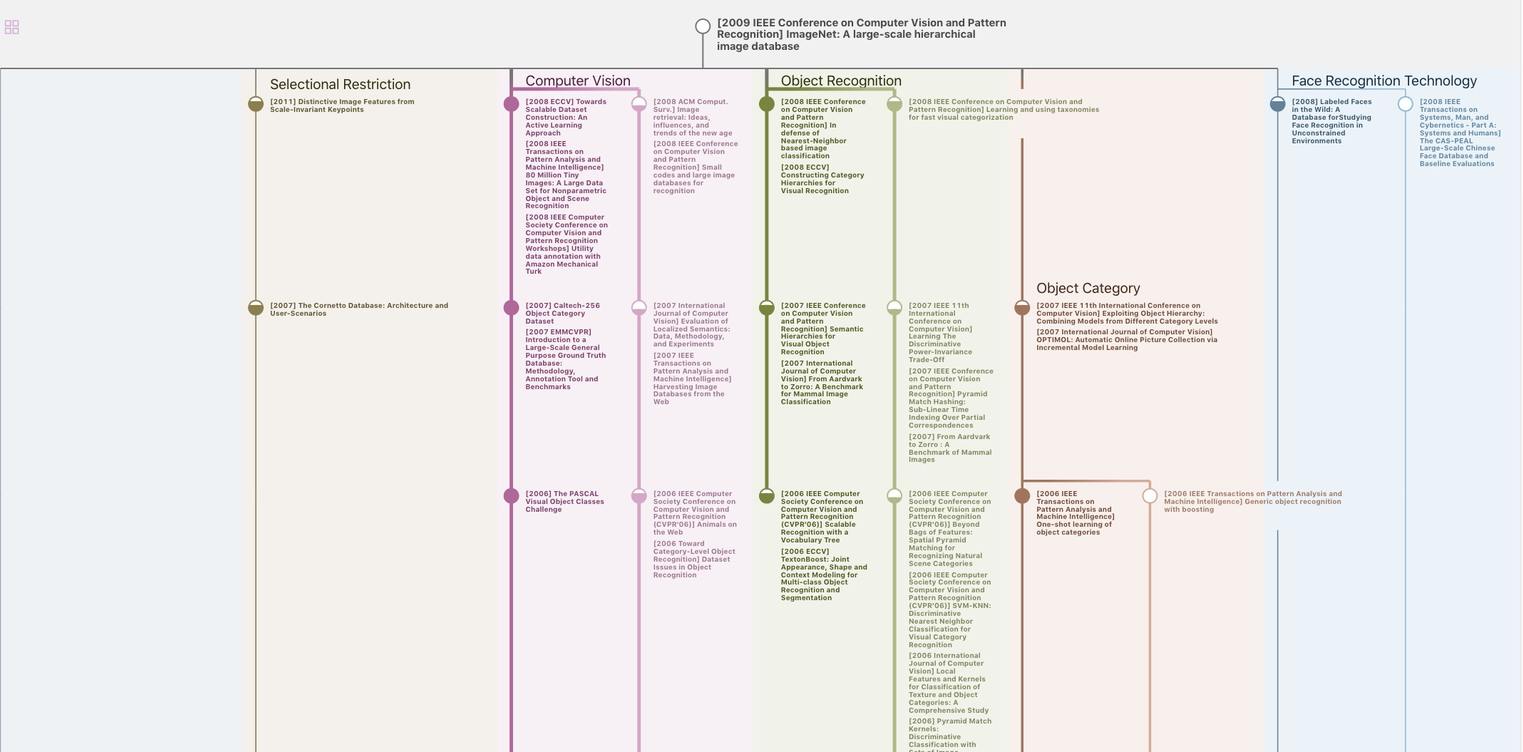
生成溯源树,研究论文发展脉络
Chat Paper
正在生成论文摘要