Unified Topological Inference for Brain Networks in Temporal Lobe Epilepsy Using the Wasserstein Distance
arxiv(2023)
摘要
Persistent homology can extract hidden topological signals present in brain networks. Persistent homology summarizes the changes of topological structures over multiple different scales called filtrations. Doing so detect hidden topological signals that persist over multiple scales. However, a key obstacle of applying persistent homology to brain network studies has always been the lack of coherent statistical inference framework. To address this problem, we present a unified topological inference framework based on the Wasserstein distance. Our approach has no explicit models and distributional assumptions. The inference is performed in a completely data driven fashion. The method is applied to the resting-state functional magnetic resonance images (rs-fMRI) of the temporal lobe epilepsy patients collected at two different sites: University of Wisconsin-Madison and the Medical College of Wisconsin. However, the topological method is robust to variations due to sex and acquisition, and thus there is no need to account for sex and site as categorical nuisance covariates. We are able to localize brain regions that contribute the most to topological differences. We made MATLAB package available at https://github.com/laplcebeltrami/dynamicTDA that was used to perform all the analysis in this study.
更多查看译文
关键词
brain networks,wasserstein distance,epilepsy,temporal lobe
AI 理解论文
溯源树
样例
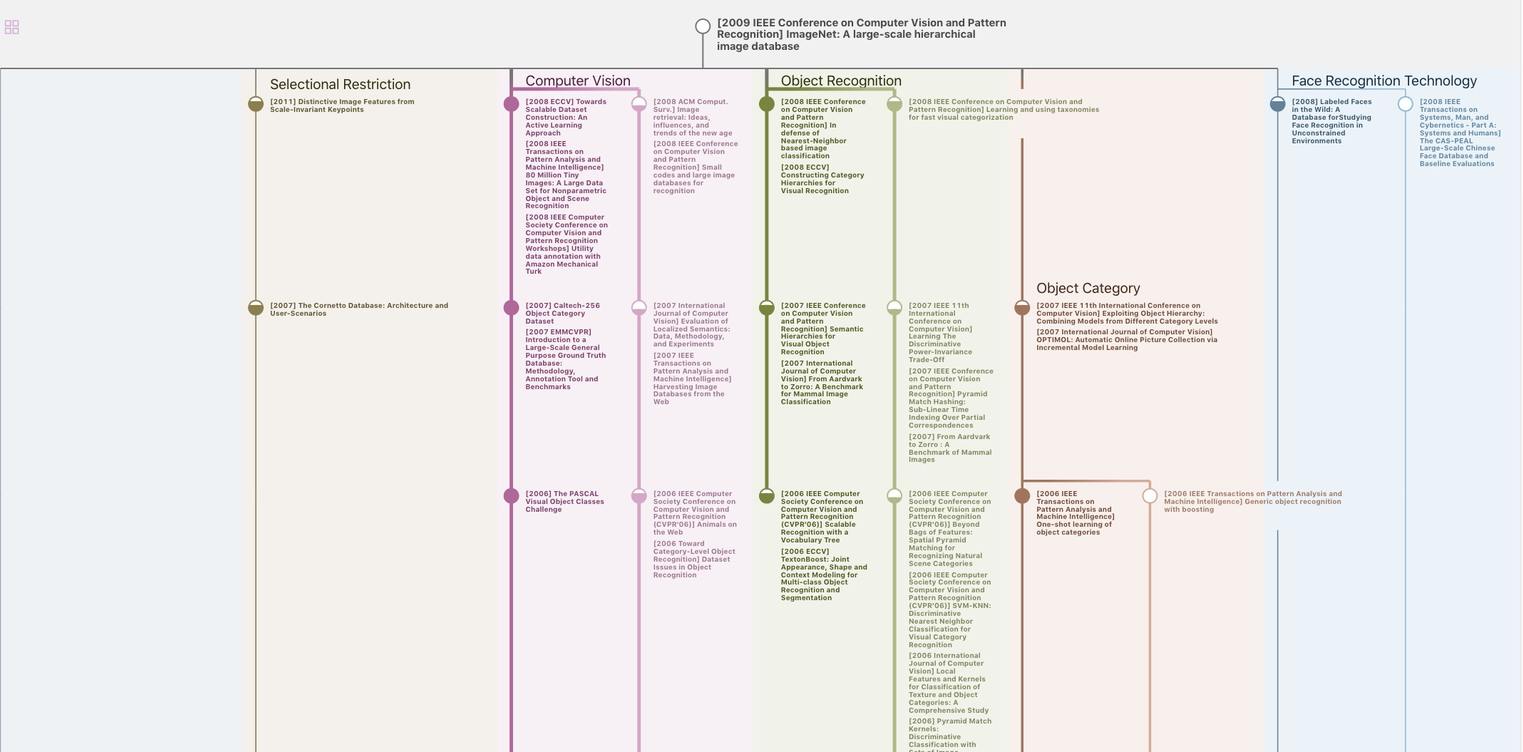
生成溯源树,研究论文发展脉络
Chat Paper
正在生成论文摘要