Marine Objects Detection Using Deep Learning on Embedded Edge Devices
2022 IEEE International Workshop on Rapid System Prototyping (RSP)(2022)
摘要
Artificial Intelligence techniques based on convolution neural networks (CNNs) are now dominant in the field of object detection and classification. The deployment of CNNs on embedded edge devices targeting real-time inference sets a challenge due to the limited computing resources and power budgets. Several optimization techniques such as pruning, quantization and use of light neural networks enable the real-time inference but at the cost of precision degradation. However, using efficient approaches to apply the optimization techniques at training and inference stages enable high inference speed with limited degradation of detection performance. In this paper, we revisit the problem of detecting and classifying maritime objects. We investigate different versions of the You Only Look Once (YOLO), a state-of-the-art deep neural network, for real-time object detection and compare their performance for the specific application of detecting maritime objects. The trained YOLO networks are efficiently optimized targeting three recent edge devices: Nvidia Jetson Xavier AGX, AMD-Xilinx Kria KV260 Vision AI Kit, and Movidius Myriad X VPU. The proposed deployments demonstrate promising results with an inference speed of 90 FPS and a limited degradation of 2.4% in mean average precision.
更多查看译文
关键词
Marine,Deep learning,Embedded edge devices,YOLO,Ship detection,Ship dataset,Optimization
AI 理解论文
溯源树
样例
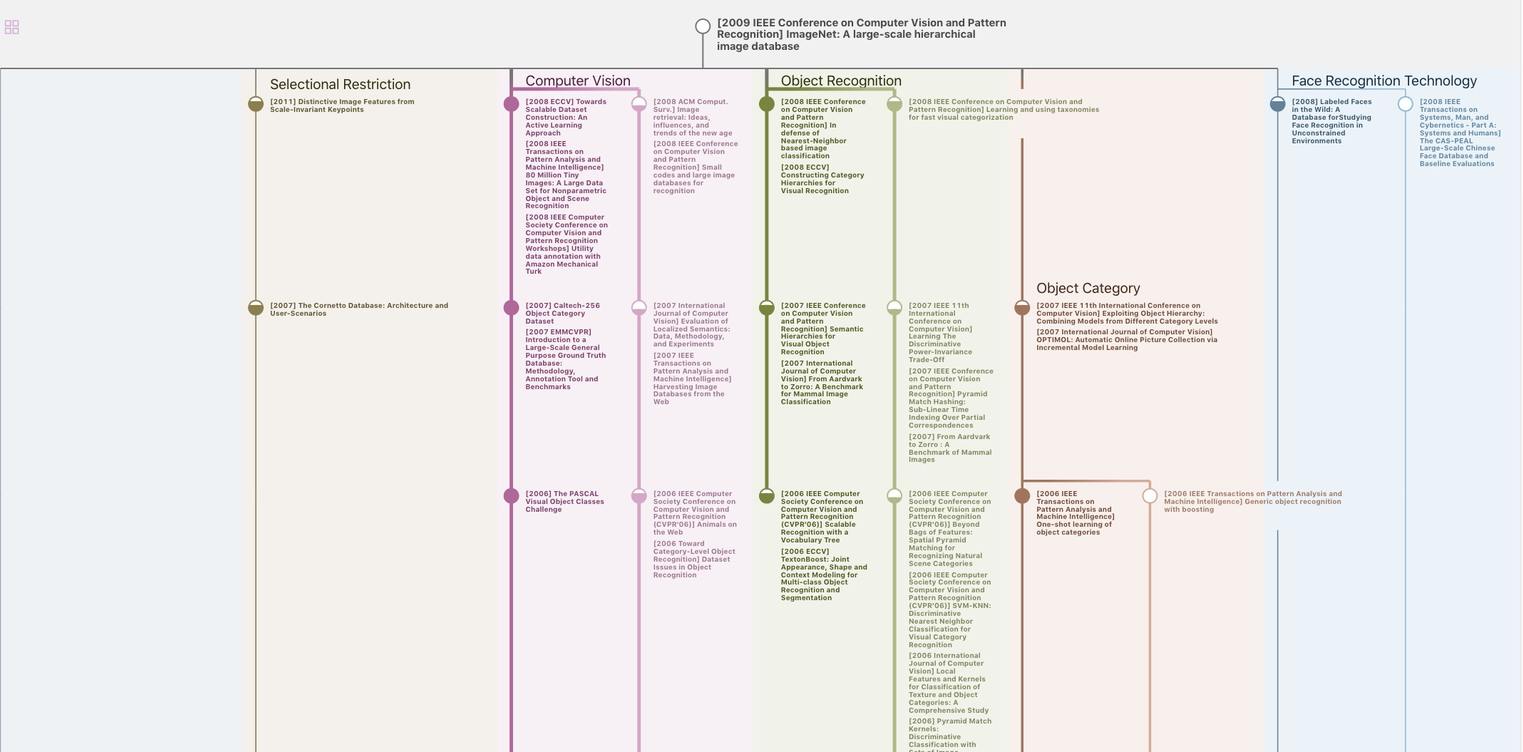
生成溯源树,研究论文发展脉络
Chat Paper
正在生成论文摘要