A Distributed Nesterov-Like Gradient Tracking Algorithm for Composite Constrained Optimization
IEEE Transactions on Signal and Information Processing over Networks(2023)
摘要
This paper focuses on the constrained optimization problem where the objective function is composed of smooth (possibly nonconvex) and nonsmooth parts. The proposed algorithm integrates the successive convex approximation (SCA) technique with the gradient tracking mechanism that aims at achieving a linear convergence rate and employing the momentum term to regulate update directions in each time instant. It is proved that the proposed algorithm converges provided that the constant step size and momentum parameter are lower than the given upper bounds. When the smooth part is strongly convex, the proposed algorithm linearly converges to the global optimal solution, whereas it converges to a local stationary solution with a sub-linear convergence rate if the smooth part is nonconvex. Numerical simulations are applied to demonstrate the validity of the proposed algorithm and the theoretical analysis.
更多查看译文
关键词
Successive convex approximation (SCA),nonconvex optimization,Nesterov method,gradient tracking,distributed optimization
AI 理解论文
溯源树
样例
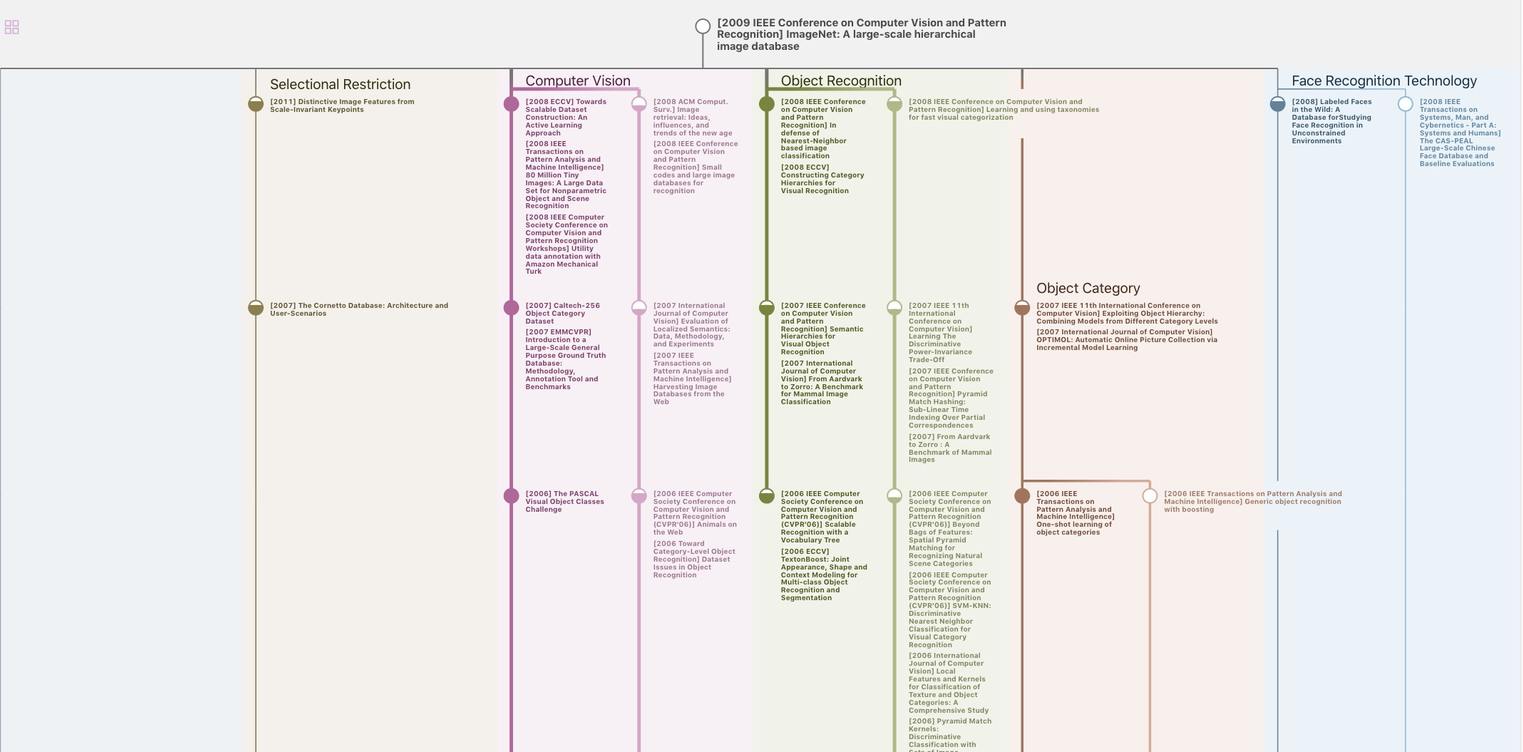
生成溯源树,研究论文发展脉络
Chat Paper
正在生成论文摘要