Gradient-Based Automated Iterative Recovery for Parameter-Efficient Tuning
arxiv(2023)
摘要
Pretrained large language models (LLMs) are able to solve a wide variety of tasks through transfer learning. Various explainability methods have been developed to investigate their decision making process. TracIn (Pruthi et al., 2020) is one such gradient-based method which explains model inferences based on the influence of training examples. In this paper, we explore the use of TracIn to improve model performance in the parameter-efficient tuning (PET) setting. We develop conversational safety classifiers via the prompt-tuning PET method and show how the unique characteristics of the PET regime enable TracIn to identify the cause for certain misclassifications by LLMs. We develop a new methodology for using gradient-based explainability techniques to improve model performance, G-BAIR: gradient-based automated iterative recovery. We show that G-BAIR can recover LLM performance on benchmarks after manually corrupting training labels. This suggests that influence methods like TracIn can be used to automatically perform data cleaning, and introduces the potential for interactive debugging and relabeling for PET-based transfer learning methods.
更多查看译文
关键词
tuning,automated iterative recovery,gradient-based,parameter-efficient
AI 理解论文
溯源树
样例
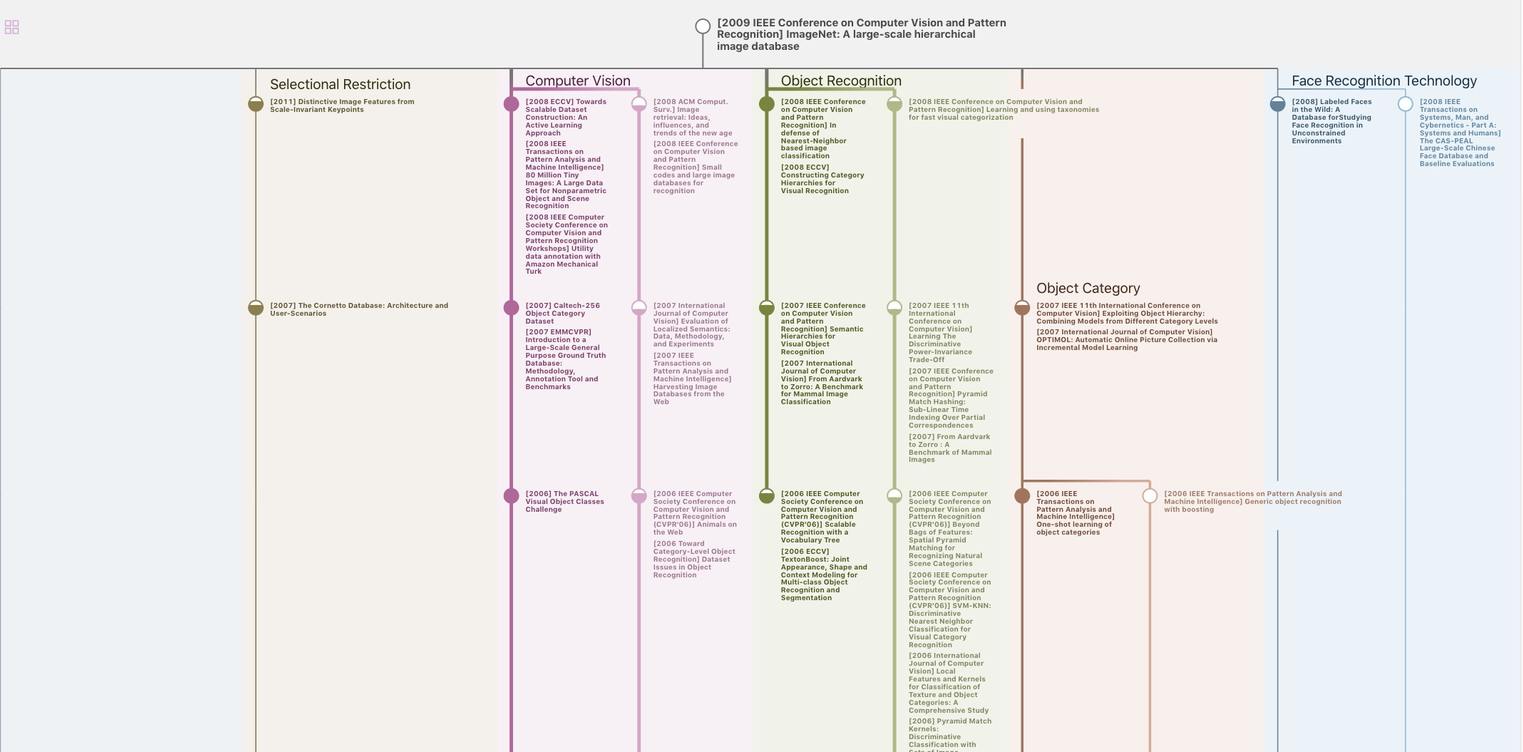
生成溯源树,研究论文发展脉络
Chat Paper
正在生成论文摘要