Deep-Learning Quantitative Structural Characterization in Additive Manufacturing
arxiv(2023)
摘要
With a goal of accelerating fabrication of additively manufactured components with precise microstructures, we developed a method for structural characterization of key features in additively manufactured materials and parts. The method utilizes deep learning based on an image-to-image translation conditional Generative Adversarial Neural Network architecture and enables fast and incrementally more accurate predictions of the prevalent geometric features, including melt pool boundaries and printing induced defects visible in etched optical images. These structural details are heterogeneous in nature. Our method specifies the microstructure state of an additive built via statistical distribution of structural details, based on an ensemble of collected images. Extensions of the method are proposed to address Artificial Intelligence implementation of developed machine learning model for in real time control of additive manufacturing.
更多查看译文
关键词
deep-learning deep-learning,structural characterization,additive
AI 理解论文
溯源树
样例
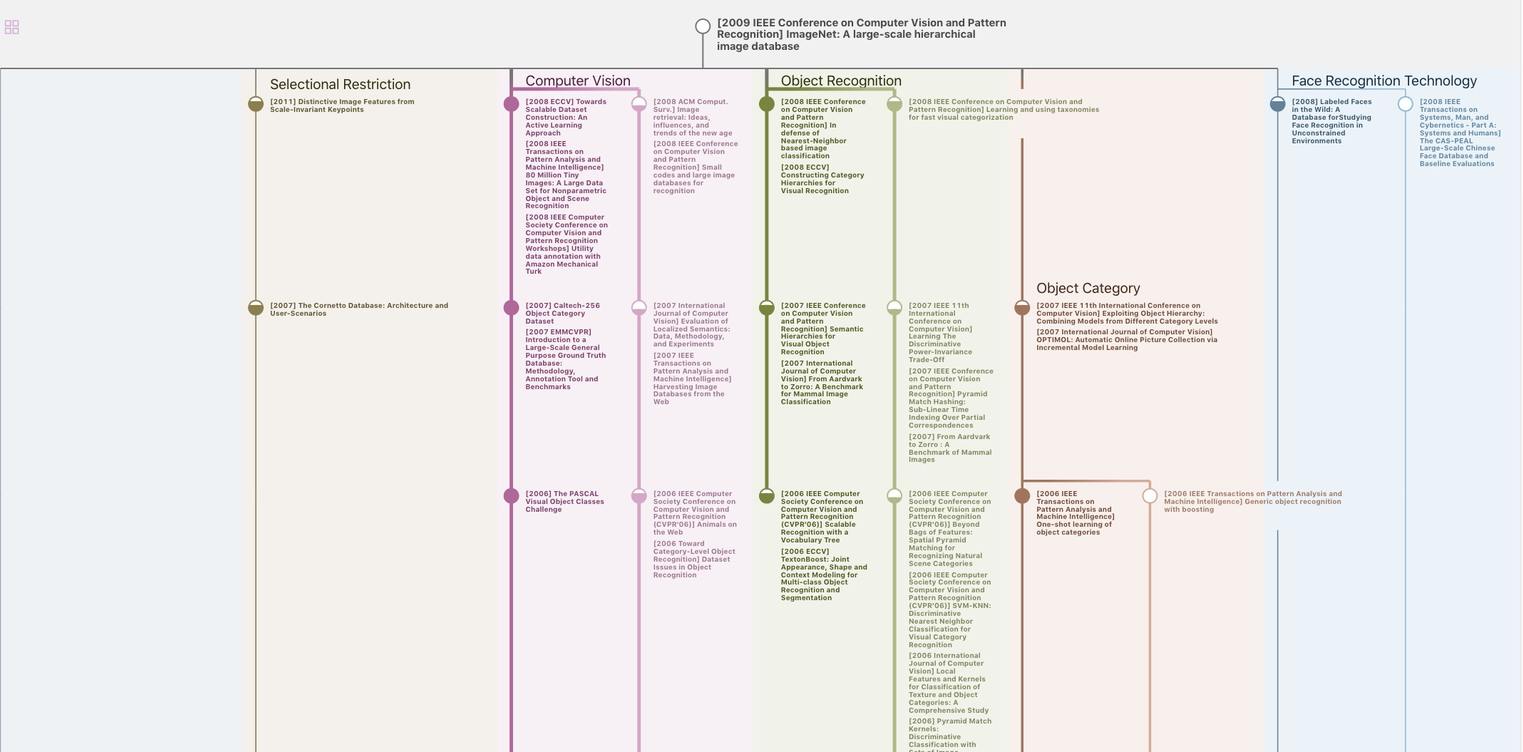
生成溯源树,研究论文发展脉络
Chat Paper
正在生成论文摘要