One Transformer for All Time Series: Representing and Training with Time-Dependent Heterogeneous Tabular Data
arxiv(2023)
摘要
There is a recent growing interest in applying Deep Learning techniques to tabular data, in order to replicate the success of other Artificial Intelligence areas in this structured domain. Specifically interesting is the case in which tabular data have a time dependence, such as, for instance financial transactions. However, the heterogeneity of the tabular values, in which categorical elements are mixed with numerical items, makes this adaptation difficult. In this paper we propose a Transformer architecture to represent heterogeneous time-dependent tabular data, in which numerical features are represented using a set of frequency functions and the whole network is uniformly trained with a unique loss function.
更多查看译文
关键词
transformer,time series,data,time-dependent
AI 理解论文
溯源树
样例
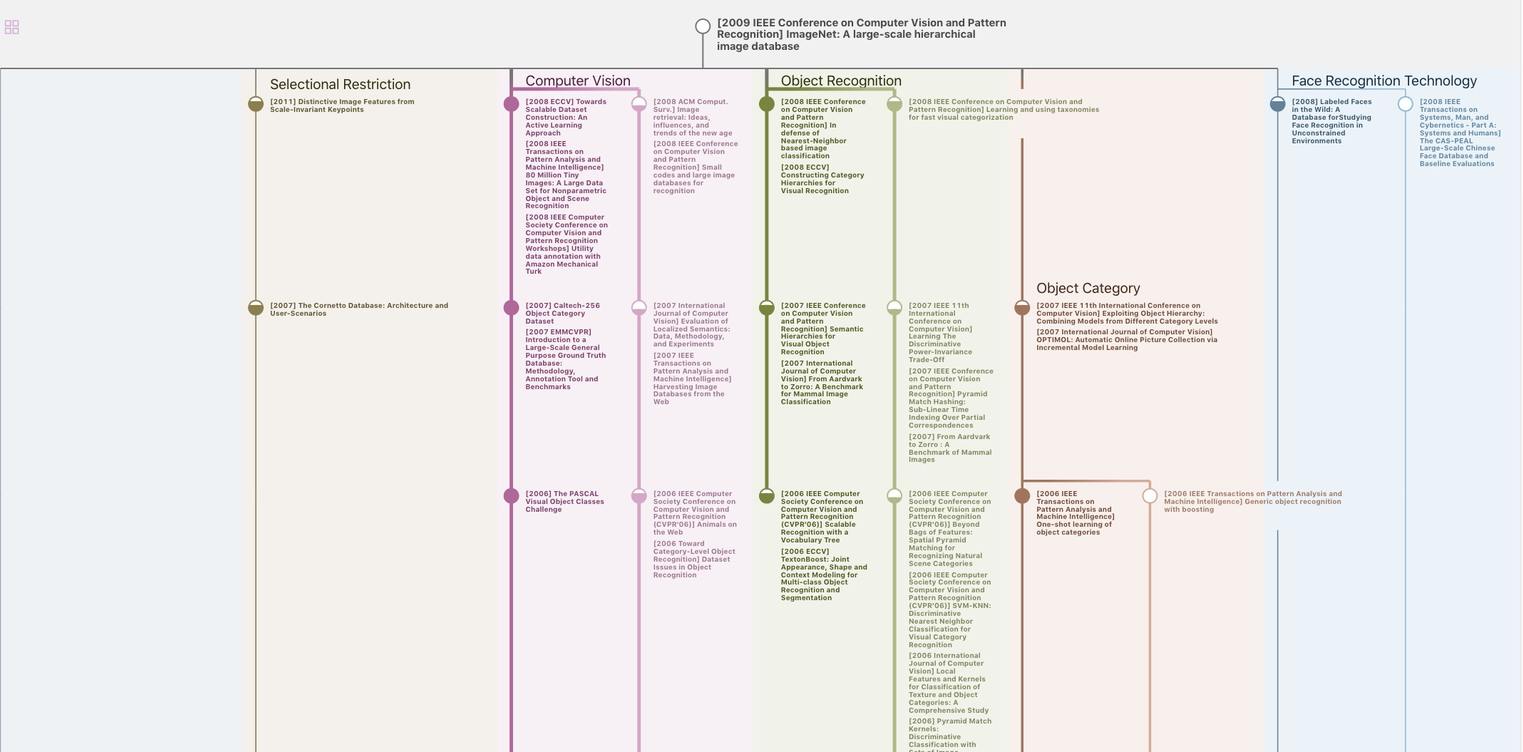
生成溯源树,研究论文发展脉络
Chat Paper
正在生成论文摘要