From high-dimensional & mean-field dynamics to dimensionless ODEs: A unifying approach to SGD in two-layers networks
arxiv(2023)
摘要
This manuscript investigates the one-pass stochastic gradient descent (SGD) dynamics of a two-layer neural network trained on Gaussian data and labels generated by a similar, though not necessarily identical, target function. We rigorously analyse the limiting dynamics via a deterministic and low-dimensional description in terms of the sufficient statistics for the population risk. Our unifying analysis bridges different regimes of interest, such as the classical gradient-flow regime of vanishing learning rate, the high-dimensional regime of large input dimension, and the overparameterised "mean-field" regime of large network width, covering as well the intermediate regimes where the limiting dynamics is determined by the interplay between these behaviours. In particular, in the high-dimensional limit, the infinite-width dynamics is found to remain close to a low-dimensional subspace spanned by the target principal directions. Our results therefore provide a unifying picture of the limiting SGD dynamics with synthetic data.
更多查看译文
关键词
sgd,networks,dynamics,odes,high-dimensional,mean-field,two-layers
AI 理解论文
溯源树
样例
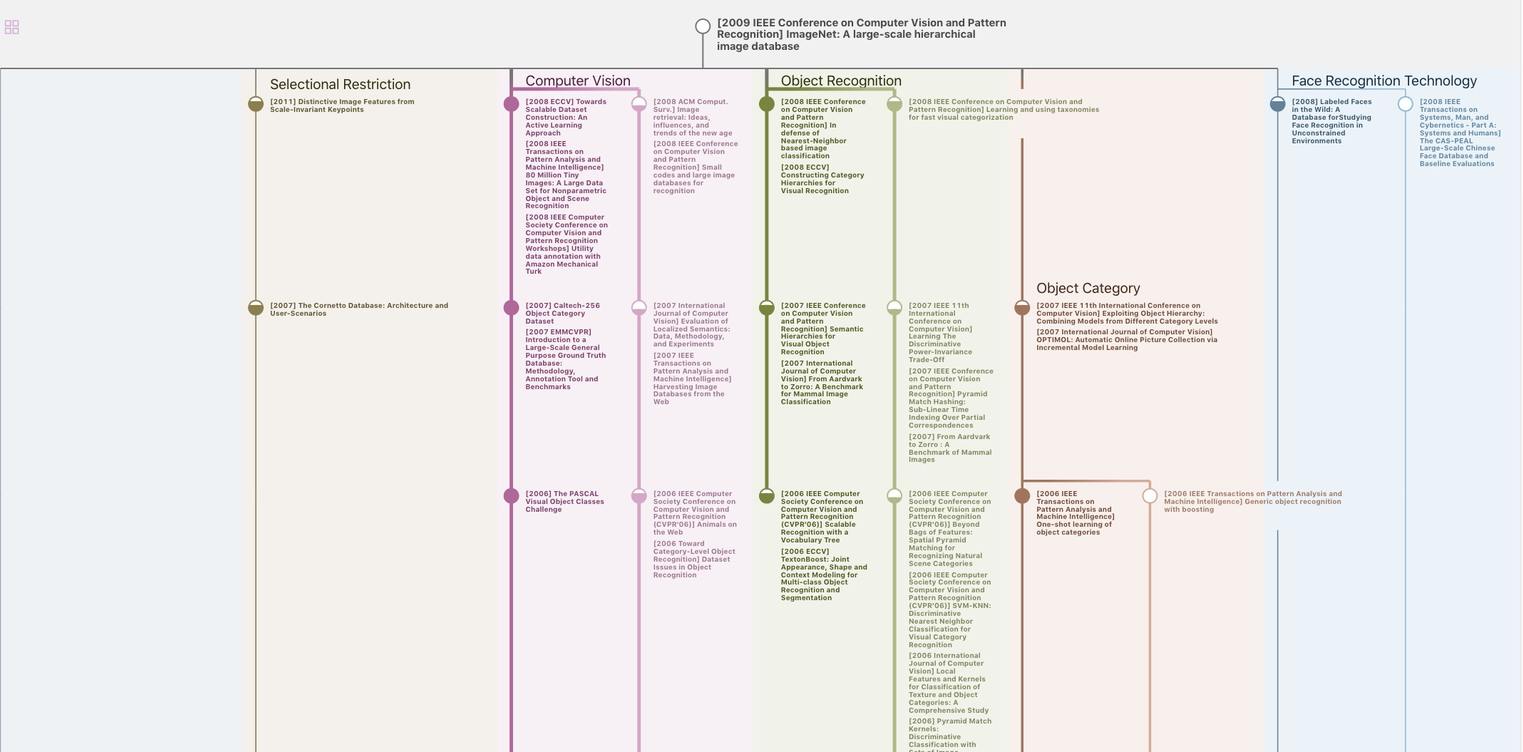
生成溯源树,研究论文发展脉络
Chat Paper
正在生成论文摘要