Sparse Mutation Decompositions: Fine Tuning Deep Neural Networks with Subspace Evolution
PROCEEDINGS OF THE 2023 GENETIC AND EVOLUTIONARY COMPUTATION CONFERENCE COMPANION, GECCO 2023 COMPANION(2023)
摘要
Neuroevolution is a promising area of research that combines evolutionary algorithms and neural networks. A popular subclass of neuroevolutionary methods, called evolution strategies, rely on dense noise perturbations to mutate networks, which can be sample inefficient and challenging for large models with millions of parameters. We introduce an approach to alleviating this problem by decomposing dense mutations into low-dimensional subspaces. Restricting mutations in this way can significantly reduce variance as networks can handle stronger perturbations while maintaining performance. This approach is uniquely effective for the task of fine tuning pre-trained models, which is an increasingly valuable area of research as networks continue to scale in size and open source models become more widely available. We conduct an exploration of sparse mutation decompositions on the difficult ImageNet dataset, where we see small generalization improvements with only a single evolutionary generation across a wide variety of deep neural network architectures.
更多查看译文
关键词
neural networks,evolution strategies,ensemble methods
AI 理解论文
溯源树
样例
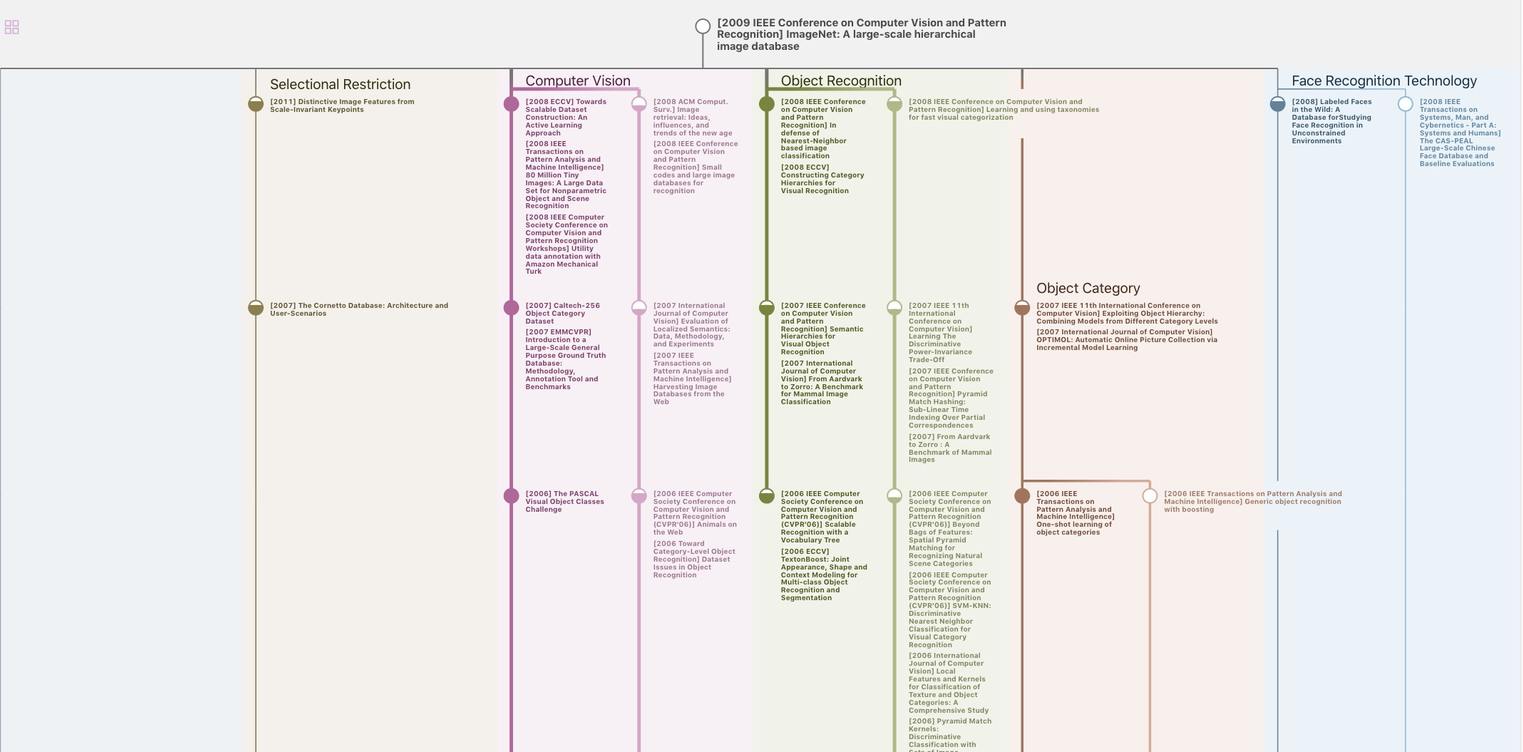
生成溯源树,研究论文发展脉络
Chat Paper
正在生成论文摘要