Long-Context Language Decision Transformers and Exponential Tilt for Interactive Text Environments
arxiv(2023)
摘要
Text-based game environments are challenging because agents must deal with long sequences of text, execute compositional actions using text and learn from sparse rewards. We address these challenges by proposing Long-Context Language Decision Transformers (LLDTs), a framework that is based on long transformer language models and decision transformers (DTs). LLDTs extend DTs with 3 components: (1) exponential tilt to guide the agent towards high obtainable goals, (2) novel goal conditioning methods yielding significantly better results than the traditional return-to-go (sum of all future rewards), and (3) a model of future observations. Our ablation results show that predicting future observations improves agent performance. To the best of our knowledge, LLDTs are the first to address offline RL with DTs on these challenging games. Our experiments show that LLDTs achieve the highest scores among many different types of agents on some of the most challenging Jericho games, such as Enchanter.
更多查看译文
关键词
interactive long-context environments,exponential tilt,language
AI 理解论文
溯源树
样例
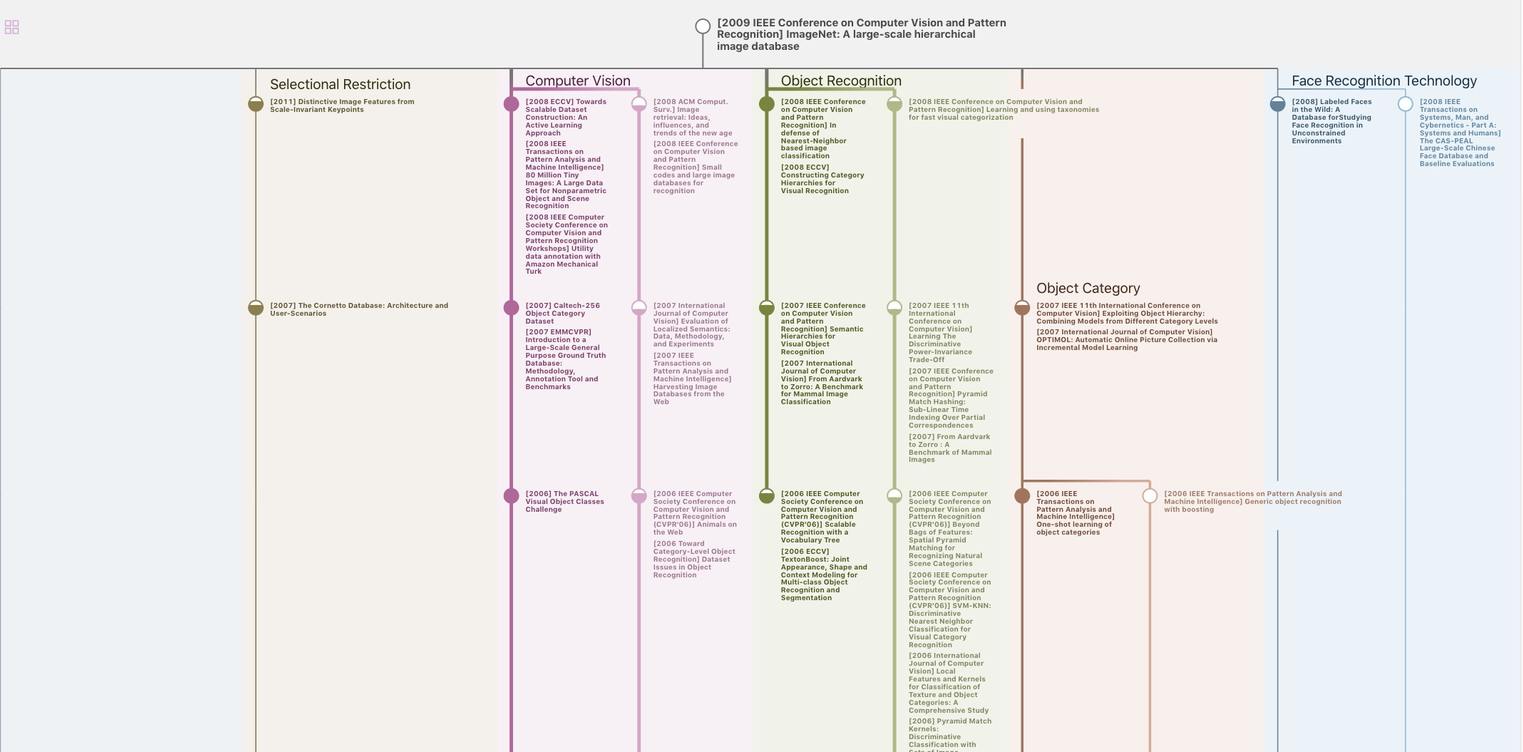
生成溯源树,研究论文发展脉络
Chat Paper
正在生成论文摘要