STR-based feature extraction and selection for genetic feature discovery in neurological disease genes
Scientific reports(2023)
摘要
Gene expression, often determined by single nucleotide polymorphisms, short repeated sequences known as short tandem repeats (STRs), structural variants, and environmental factors, provides means for an organism to produce gene products necessary to live. Variation in expression levels, sometimes known as enrichment patterns, has been associated with disease progression. Thus, the STR enrichment patterns have recently gained interest as potential genetic markers for disease progression. However, to the best of our knowledge, we are unaware of any study that evaluates and explores STRs, particularly trinucleotide sequences, as machine learning features for classifying neurological disease genes for the purpose of discovering genetic features. Thus, in this paper, we proposed a new metric and a novel feature extraction and selection algorithm based on statistically significant STR-based features and their respective enrichment patterns to create a statistically significant feature set. The proposed new metric has shown that the neurological disease family genes have a non-random AA, AT, TA, TG, and TT enrichment pattern. This is an important result, as it supports prior research that has established that certain trinucleotides, such as AAT, ATA, ATT, TAT, and TTA, are favored during protein misfolding. In contrast, trinucleotides, such as TAA, TAG, and TGA, are favored during premature termination codon mutations as they are stop codons. This suggests that the metric has the potential to identify patterns that may be genetic features in a sample of neurological genes. Moreover, the practical performance and high prediction results of the statistically significant STR-based feature set indicate that variations in STR enrichment patterns can distinguish neurological disease genes. In conclusion, the proposed approach may have the potential to discover differential genetic features for other diseases.
更多查看译文
关键词
Biomarkers,Gene expression,Genetic markers,Machine learning,Science,Humanities and Social Sciences,multidisciplinary
AI 理解论文
溯源树
样例
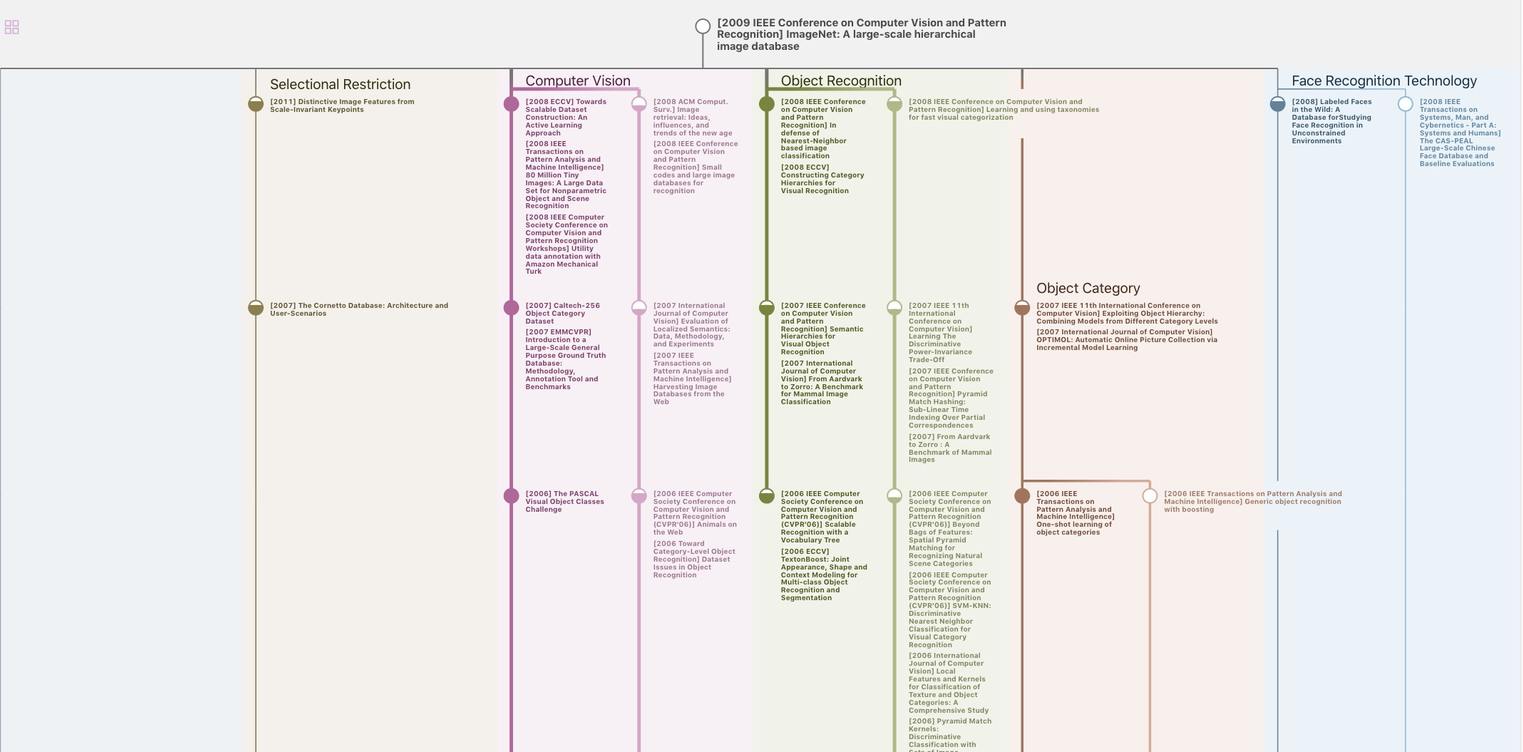
生成溯源树,研究论文发展脉络
Chat Paper
正在生成论文摘要