Action Dynamics Task Graphs for Learning Plannable Representations of Procedural Tasks
arxiv(2023)
摘要
Given video demonstrations and paired narrations of an at-home procedural task such as changing a tire, we present an approach to extract the underlying task structure -- relevant actions and their temporal dependencies -- via action-centric task graphs. Learnt structured representations from our method, Action Dynamics Task Graphs (ADTG), can then be used for understanding such tasks in unseen videos of humans performing them. Furthermore, ADTG can enable providing user-centric guidance to humans in these tasks, either for performing them better or for learning new tasks. Specifically, we show how ADTG can be used for: (1) tracking an ongoing task, (2) recommending next actions, and (3) planning a sequence of actions to accomplish a procedural task. We compare against state-of-the-art Neural Task Graph method and demonstrate substantial gains on 18 procedural tasks from the CrossTask dataset, including 30.1% improvement in task tracking accuracy and 20.3% accuracy gain in next action prediction.
更多查看译文
关键词
learning plannable representations,tasks,action,graphs
AI 理解论文
溯源树
样例
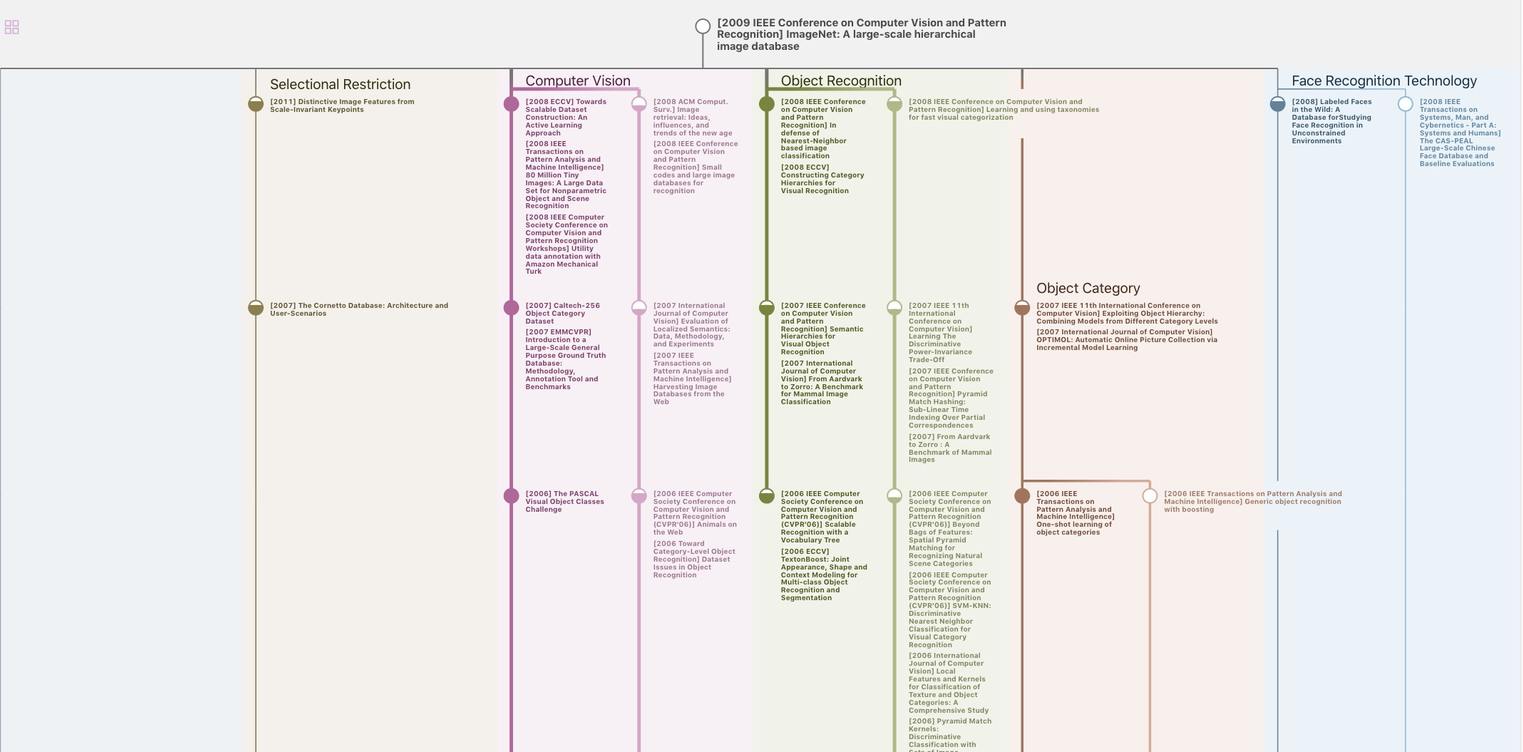
生成溯源树,研究论文发展脉络
Chat Paper
正在生成论文摘要