Two-Level Attention Module Based on Spurious-3D Residual Networks for Human Action Recognition.
Sensors(2023)
摘要
In recent years, deep learning techniques have excelled in video action recognition. However, currently commonly used video action recognition models minimize the importance of different video frames and spatial regions within some specific frames when performing action recognition, which makes it difficult for the models to adequately extract spatiotemporal features from the video data. In this paper, an action recognition method based on improved residual convolutional neural networks (CNNs) for video frames and spatial attention modules is proposed to address this problem. The network can guide what and where to emphasize or suppress with essentially little computational cost using the video frame attention module and the spatial attention module. It also employs a two-level attention module to emphasize feature information along the temporal and spatial dimensions, respectively, highlighting the more important frames in the overall video sequence and the more important spatial regions in some specific frames. Specifically, we create the video frame and spatial attention map by successively adding the video frame attention module and the spatial attention module to aggregate the spatial and temporal dimensions of the intermediate feature maps of the CNNs to obtain different feature descriptors, thus directing the network to focus more on important video frames and more contributing spatial regions. The experimental results further show that the network performs well on the UCF-101 and HMDB-51 datasets.
更多查看译文
关键词
CNNs,action recognition,attention mechanism,spatiotemporal features
AI 理解论文
溯源树
样例
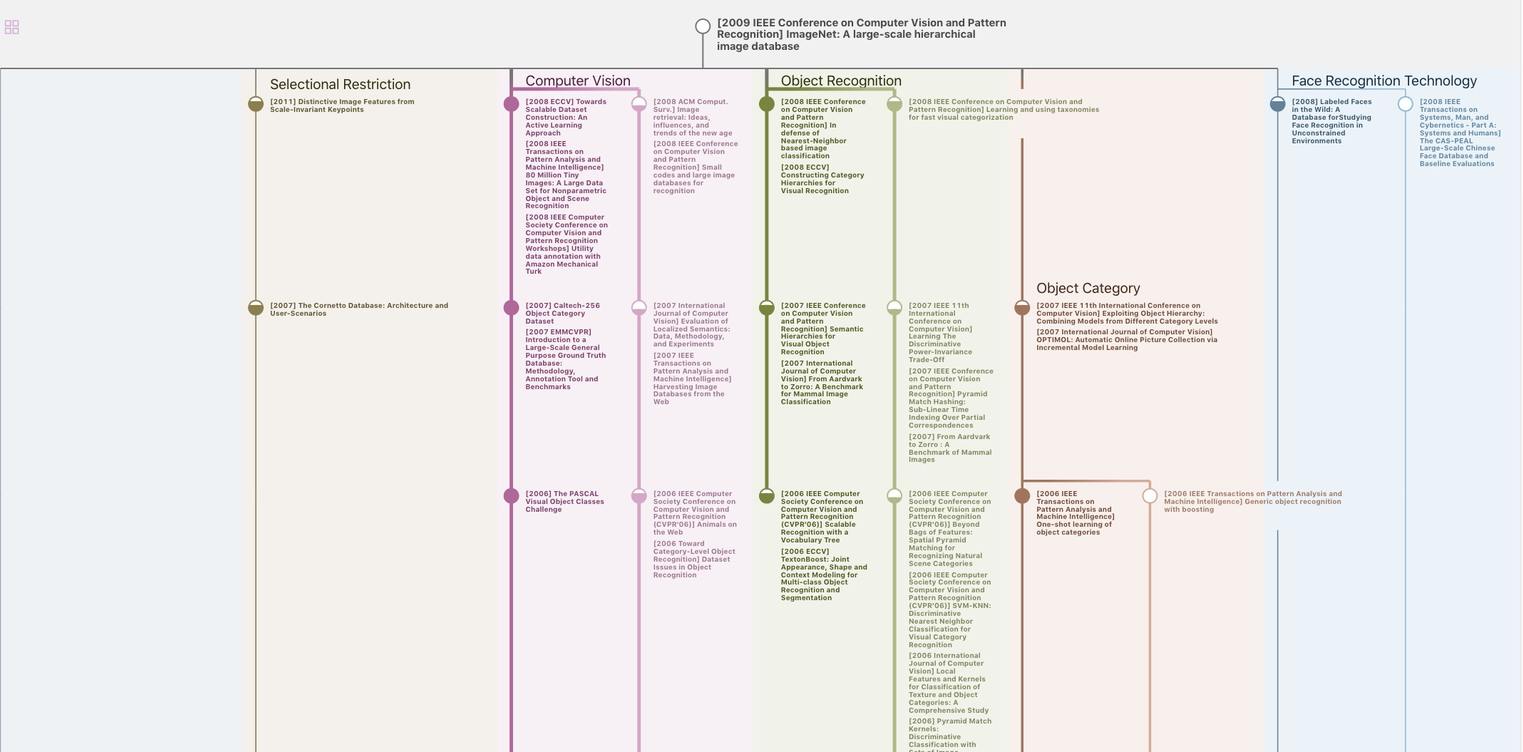
生成溯源树,研究论文发展脉络
Chat Paper
正在生成论文摘要