A Multifunctional Network with Uncertainty Estimation and Attention-Based Knowledge Distillation to Address Practical Challenges in Respiration Rate Estimation
Sensors(2023)
摘要
Respiration rate is a vital parameter to indicate good health, wellbeing, and performance. As the estimation through classical measurement modes are limited only to rest or during slow movements, respiration rate is commonly estimated through physiological signals such as electrocardiogram and photoplethysmography due to the unobtrusive nature of wearable devices. Deep learning methodologies have gained much traction in the recent past to enhance accuracy during activities involving a lot of movement. However, these methods pose challenges, including model interpretability, uncertainty estimation in the context of respiration rate estimation, and model compactness in terms of deployment in wearable platforms. In this direction, we propose a multifunctional framework, which includes the combination of an attention mechanism, an uncertainty estimation functionality, and a knowledge distillation framework. We evaluated the performance of our framework on two datasets containing ambulatory movement. The attention mechanism visually and quantitatively improved instantaneous respiration rate estimation. Using Monte Carlo dropouts to embed the network with inferential uncertainty estimation resulted in the rejection of 3.7% of windows with high uncertainty, which consequently resulted in an overall reduction of 7.99% in the mean absolute error. The attention-aware knowledge distillation mechanism reduced the model's parameter count and inference time by 49.5% and 38.09%, respectively, without any increase in error rates. Through experimentation, ablation, and visualization, we demonstrated the efficacy of the proposed framework in addressing practical challenges, thus taking a step towards deployment in wearable edge devices.
更多查看译文
关键词
deep learning,respiration rate,wearable technology,multifunctional network,attention mechanism,uncertainty estimation,model compactness
AI 理解论文
溯源树
样例
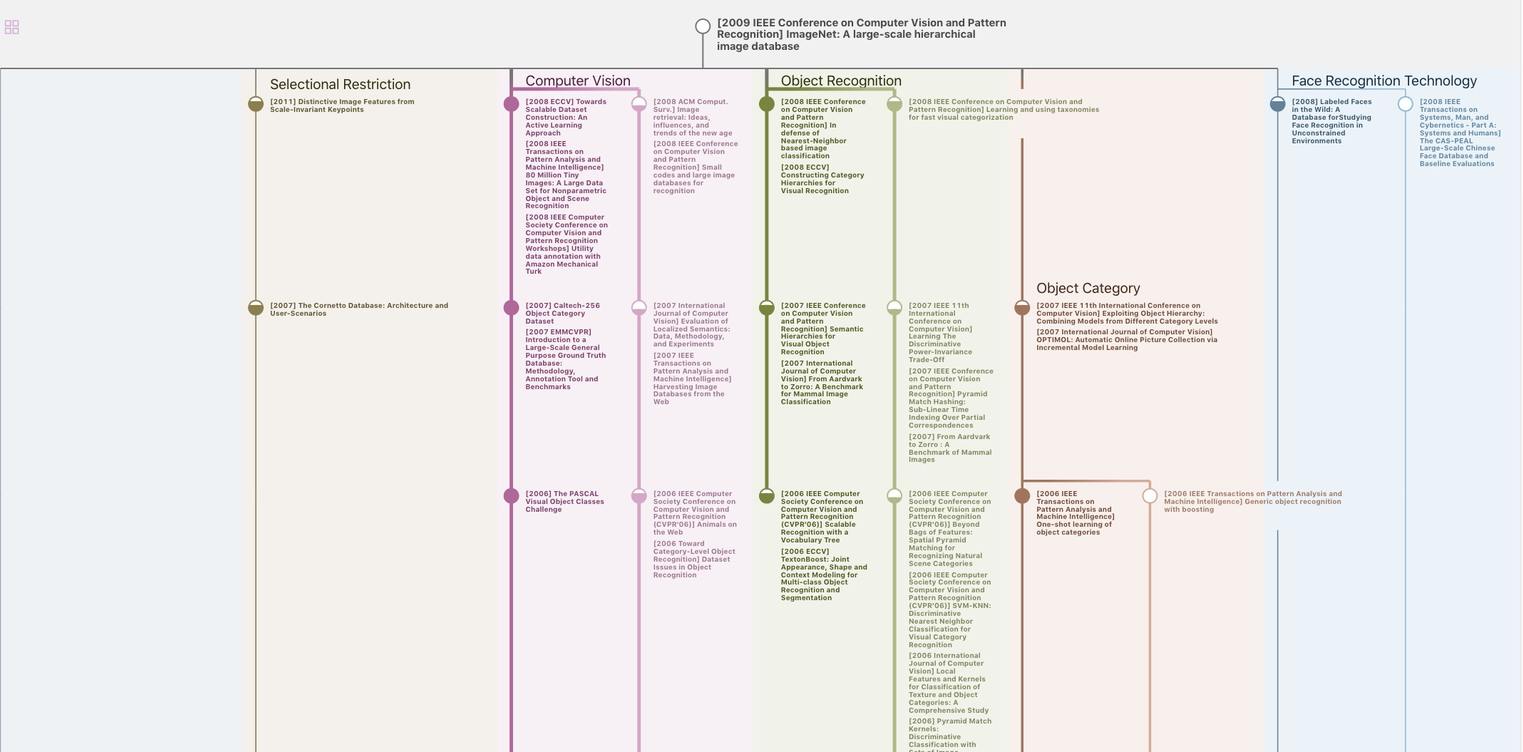
生成溯源树,研究论文发展脉络
Chat Paper
正在生成论文摘要