Robust Image Processing Framework for Intelligent Multi-Stage Malaria Parasite Recognition of Thick and Thin Smear Images.
Diagnostics (Basel, Switzerland)(2023)
摘要
Malaria is a pressing medical issue in tropical and subtropical regions. Currently, the manual microscopic examination remains the gold standard malaria diagnosis method. Nevertheless, this procedure required highly skilled lab technicians to prepare and examine the slides. Therefore, a framework encompassing image processing and machine learning is proposed due to inconsistencies in manual inspection, counting, and staging. Here, a standardized segmentation framework utilizing thresholding and clustering is developed to segment parasites' stages of and species. Moreover, a multi-stage classifier is designed for recognizing parasite species and staging in both species. Experimental results indicate the effectiveness of segmenting thick smear images based on Phansalkar thresholding garnered an accuracy of 99.86%. The employment of variance and new transferring process for the clustered members, enhanced -means (EKM) clustering has successfully segmented all malaria stages with accuracy and an F1-score of 99.20% and 0.9033, respectively. In addition, the accuracies of parasite detection, species recognition, and staging obtained through a random forest (RF) accounted for 86.89%, 98.82%, and 90.78%, respectively, simultaneously. The proposed framework enables versatile malaria parasite detection and staging with an interactive result, paving the path for future improvements by utilizing the proposed framework on all others malaria species.
更多查看译文
关键词
clustering,image segmentation,machine learning,plasmodium parasite,species and staging,thresholding
AI 理解论文
溯源树
样例
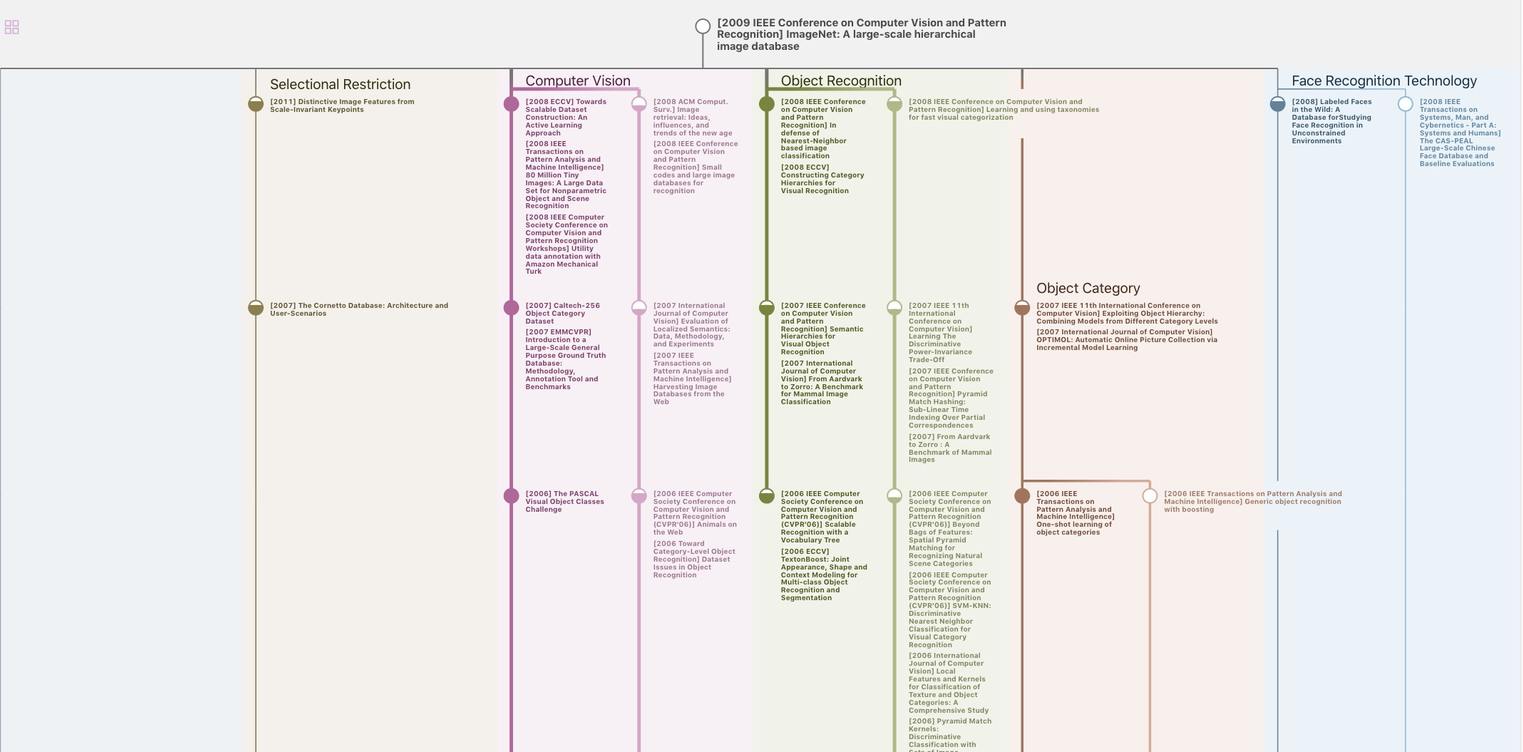
生成溯源树,研究论文发展脉络
Chat Paper
正在生成论文摘要