Characterization of oxidative damage induced by nanoparticles via mechanism-driven machine learning approaches.
The Science of the total environment(2023)
摘要
The wide application of TiO-based engineered nanoparticles (nTiO) inevitably led to release into aquatic ecosystems. Importantly, increasing studies have emphasized the high risks of nTiO to coastal environments. Bivalves, the representative benthic filter feeders in coastal zones, acted as important roles to assess and monitor the toxic effects of nanoparticles. Oxidative damage was one of the main toxic mechanisms of nTiO on bivalves, but the experimental variables/nanomaterial characteristics were diverse and the toxicity mechanism was complex. Therefore, it was very necessary to develop machine learning model to characterize and predict the potential toxicity. In this study, thirty-six machine learning models were built by nanodescriptors combined with six machine learning algorithms. Among them, random forest (RF) - catalase (CAT), k-neighbors classifier (KNN) - glutathione peroxidase (GPx), neural networks - multilayer perceptron (ANN) - glutathione s-transferase (GST), random forest (RF) - malondialdehyde (MDA), random forest (RF) - reactive oxygen species (ROS), and extreme gradient boosting decision tree (XGB) - superoxide dismutase (SOD) models performed good with high accuracy and balanced accuracy for both training sets and external validation sets. Furthermore, the best model revealed the predominant factors (exposure concentration, exposure periods, and exposure matrix) influencing the oxidative stress induced by nTiO. These results showed that high exposure concentrations and short exposure-intervals tended to cause oxidative damage to bivalves. In addition, gills and digestive glands could be vulnerable to nTiO-induced oxidative damage as tissues/organs differences were the important factors controlling MDA activity. This study provided insights into important nano-features responsible for the different indicators of oxidative stress and thereby extended the application of machine learning approaches in toxicological assessment for nanoparticles.
更多查看译文
关键词
Machine learning,Mechanism-driven analysis,Risk assessment,TiO(2)-based engineered nanoparticles (nTiO(2)),Toxic effects
AI 理解论文
溯源树
样例
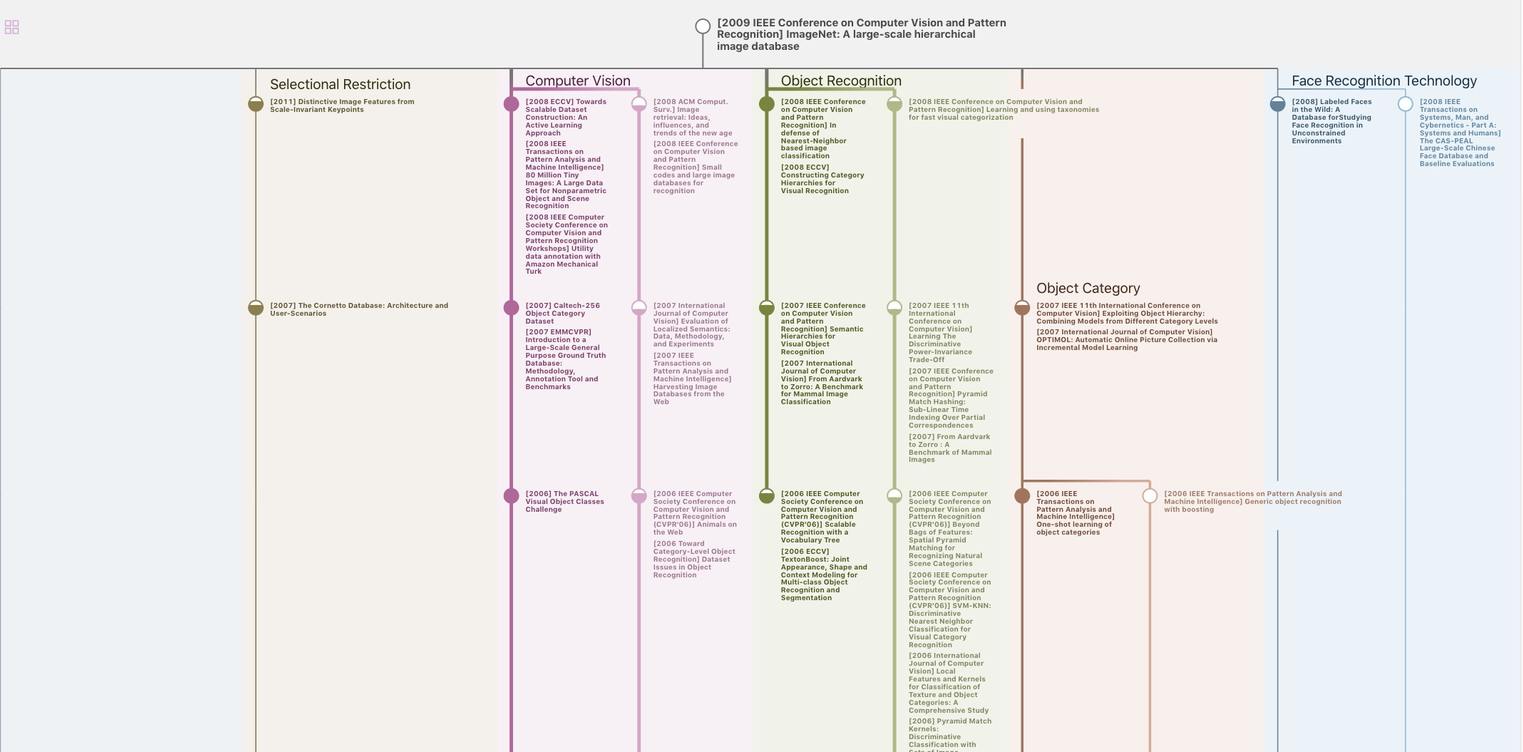
生成溯源树,研究论文发展脉络
Chat Paper
正在生成论文摘要