Detection of Alzheimer’s Disease Using Dynamic Functional Connectivity Patterns in Resting-State fMRI
2022 International Conference on Future Trends in Smart Communities (ICFTSC)(2022)
摘要
Alzheimer’s disease (AD) is a slowly progressive neurological disorder associated with impaired functional connectivity of the brain. A common approach is to examine functional connectivity patterns (FC) for AD diagnosis either statically based on Pearson correlation coefficients (PCC) or dynamically based on time-frequency coefficients of resting-state fMRI BOLD signals. However, there is still a need to develop a AD diagnostic model with dynamic FC patterns that can improve the performance of the classifier. In this paper, a classification of AD from normal cases is proposed by combining a machine learning algorithm with dynamic FC patterns (DFC). The proposed method introduces a new feature vector for the maximum value of variation in the time-frequency domain, called (MWCF). Moreover, analysis of variance (ANOVA) is used to select the most informative features. Compared to previous studies, the proposed method outperforms state-of-the-art methods with an accuracy of 98.4%. The proposed method is an efficient predictor for the classification of AD vs. NC and can be used as a potential biomarker in AD diagnosis.
更多查看译文
关键词
Alzheimer disease,Resting state fMRI,BOLD signal,Dynamic functional connectivity,SVM
AI 理解论文
溯源树
样例
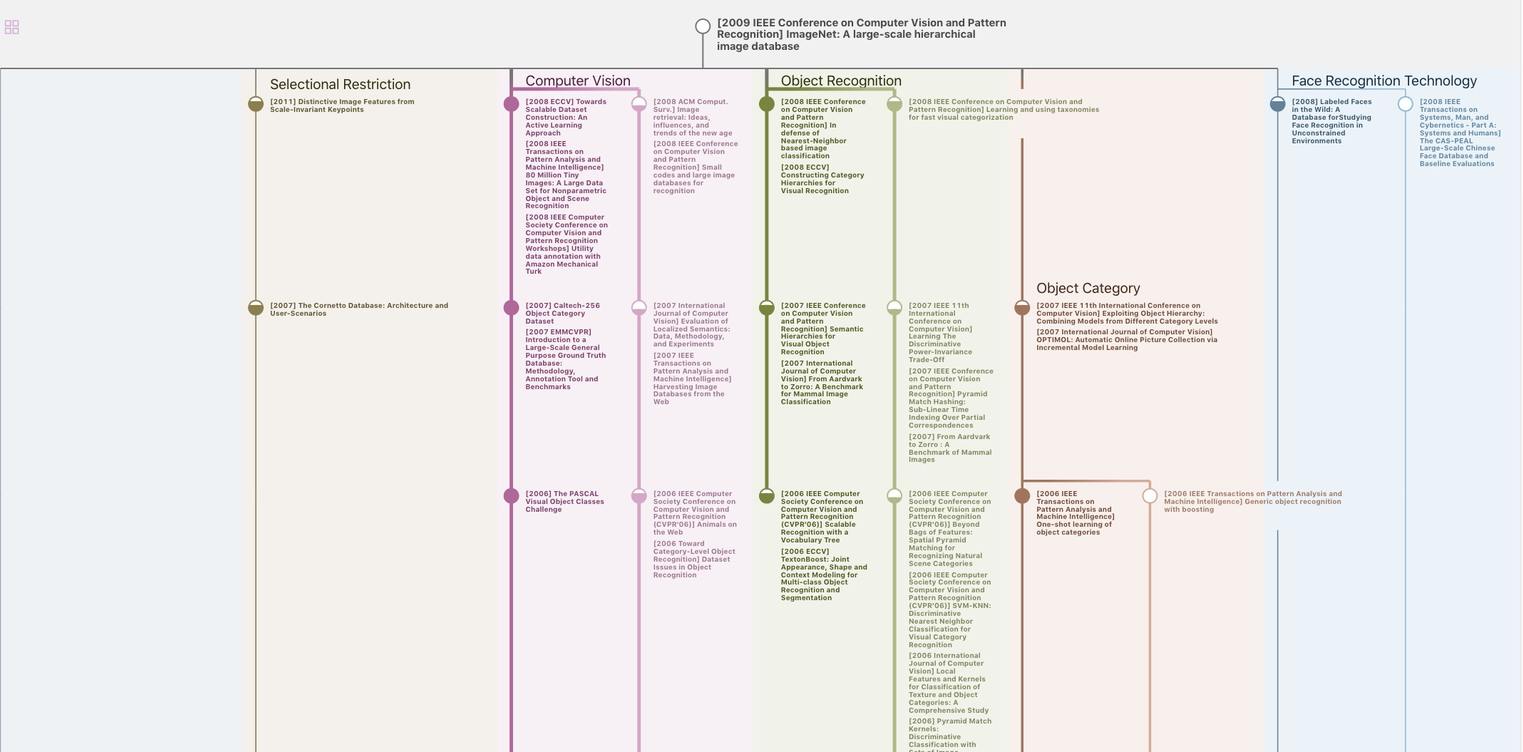
生成溯源树,研究论文发展脉络
Chat Paper
正在生成论文摘要