Developing prediction models when there are systematically missing predictors in individual patient data meta-analysis.
Research synthesis methods(2023)
摘要
Clinical prediction models are widely used in modern clinical practice. Such models are often developed using individual patient data (IPD) from a single study, but often there are IPD available from multiple studies. This allows using meta-analytical methods for developing prediction models, increasing power and precision. Different studies, however, often measure different sets of predictors, which may result to systematically missing predictors, that is, when not all studies collect all predictors of interest. This situation poses challenges in model development. We hereby describe various approaches that can be used to develop prediction models for continuous outcomes in such situations. We compare four approaches: a "restrict predictors" approach, where the model is developed using only predictors measured in all studies; a multiple imputation approach that ignores study-level clustering; a multiple imputation approach that accounts for study-level clustering; and a new approach that develops a prediction model in each study separately using all predictors reported, and then synthesizes all predictions in a multi-study ensemble. We explore in simulations the performance of all approaches under various scenarios. We find that imputation methods and our new method outperform the restrict predictors approach. In several scenarios, our method outperformed imputation methods, especially for few studies, when predictor effects were small, and in case of large heterogeneity. We use a real dataset of 12 trials in psychotherapies for depression to illustrate all methods in practice, and we provide code in R.
更多查看译文
关键词
ensemble predictive modeling,individual patient data,meta-analysis,multilevel model,prediction research
AI 理解论文
溯源树
样例
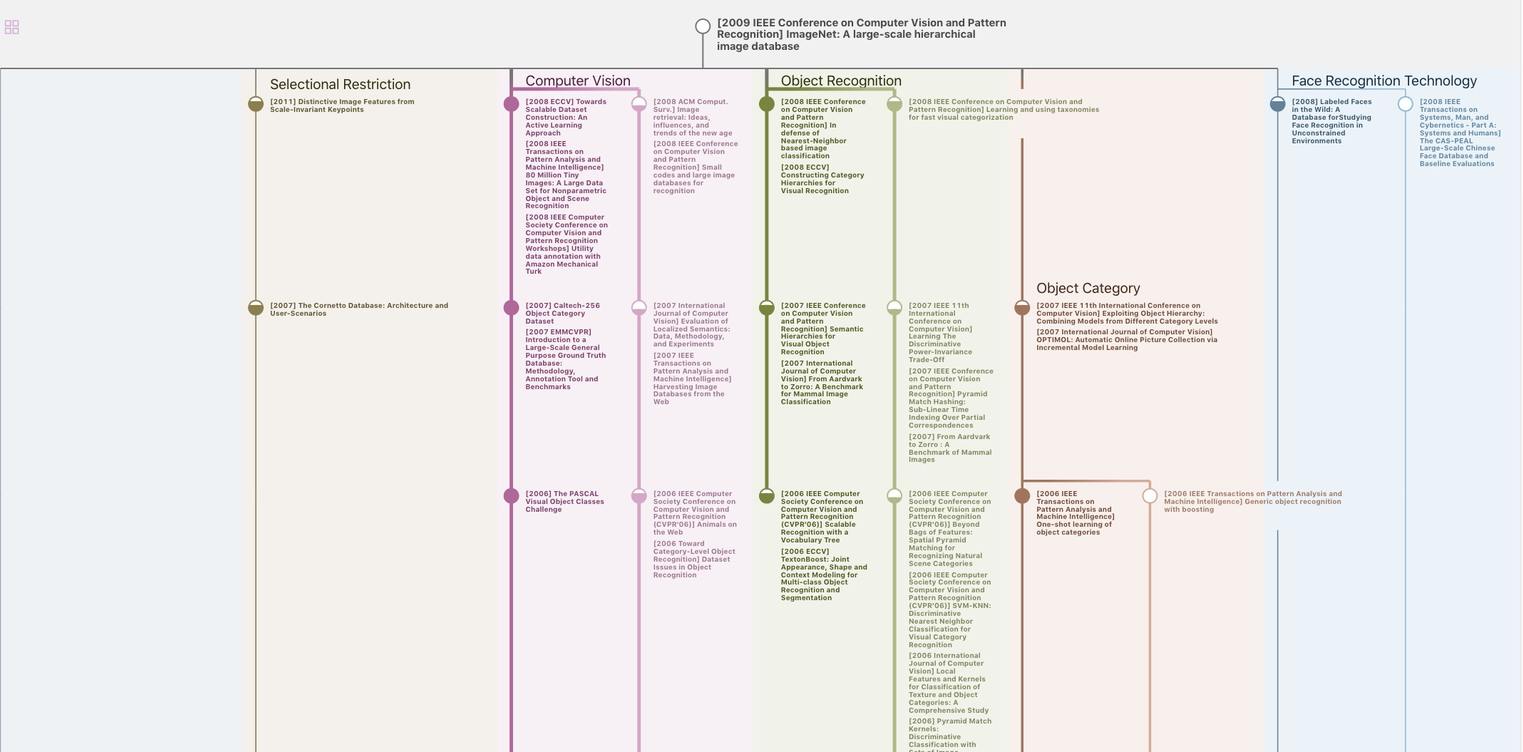
生成溯源树,研究论文发展脉络
Chat Paper
正在生成论文摘要