Mixed-order self-paced curriculum learning for universal lesion detection
arxiv(2023)
摘要
Self-paced curriculum learning (SCL) has demonstrated its great potential in computer vision, natural language processing, etc. During training, it implements easy-to-hard sampling based on online estimation of data difficulty. Most SCL methods commonly adopt a loss-based strategy of estimating data difficulty and deweighting the `hard' samples in the early training stage. While achieving success in a variety of applications, SCL stills confront two challenges in a medical image analysis task, such as universal lesion detection, featuring insufficient and highly class-imbalanced data: (i) the loss-based difficulty measurer is inaccurate; ii) the hard samples are under-utilized from a deweighting mechanism. To overcome these challenges, in this paper we propose a novel mixed-order self-paced curriculum learning (Mo-SCL) method. We integrate both uncertainty and loss to better estimate difficulty online and mix both hard and easy samples in the same mini-batch to appropriately alleviate the problem of under-utilization of hard samples. We provide a theoretical investigation of our method in the context of stochastic gradient descent optimization and extensive experiments based on the DeepLesion benchmark dataset for universal lesion detection (ULD). When applied to two state-of-the-art ULD methods, the proposed mixed-order SCL method can provide a free boost to lesion detection accuracy without extra special network designs.
更多查看译文
关键词
universal lesion
AI 理解论文
溯源树
样例
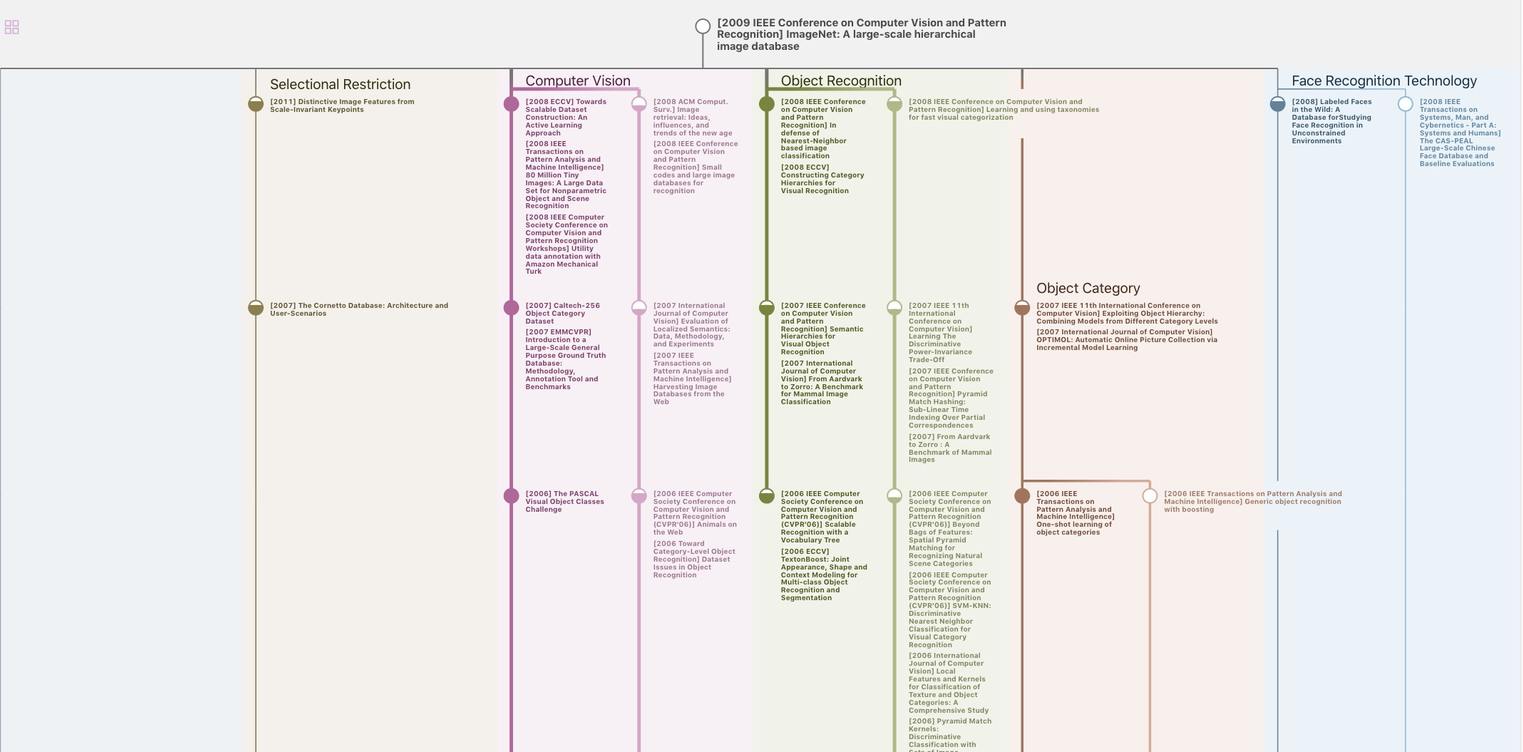
生成溯源树,研究论文发展脉络
Chat Paper
正在生成论文摘要