Exploiting Certified Defences to Attack Randomised Smoothing
ICLR 2023(2023)
摘要
In guaranteeing that no adversarial examples exist within a bounded region, certification mechanisms play an important role in neural network robustness. Concerningly, this work demonstrates that the certification mechanisms themselves introduce a new, heretofore undiscovered attack surface, that can be exploited by attackers to construct smaller adversarial perturbations. While these attacks exist outside the certification region in no way invalidate certifications, minimising a perturbation's norm significantly increases the level of difficulty associated with attack detection. In comparison to baseline attacks, our new framework yields smaller perturbations more than twice as frequently as any other approach, resulting in an up to $34 \%$ reduction in the median perturbation norm. That this approach also requires $90 \%$ less computational time than approaches like PGD. That these reductions are possible suggests that exploiting this new attack vector would allow attackers to more frequently construct hard to detect adversarial attacks, by exploiting the very systems designed to defend deployed models.
更多查看译文
关键词
adversarial,attack,certified robustness,machine learning
AI 理解论文
溯源树
样例
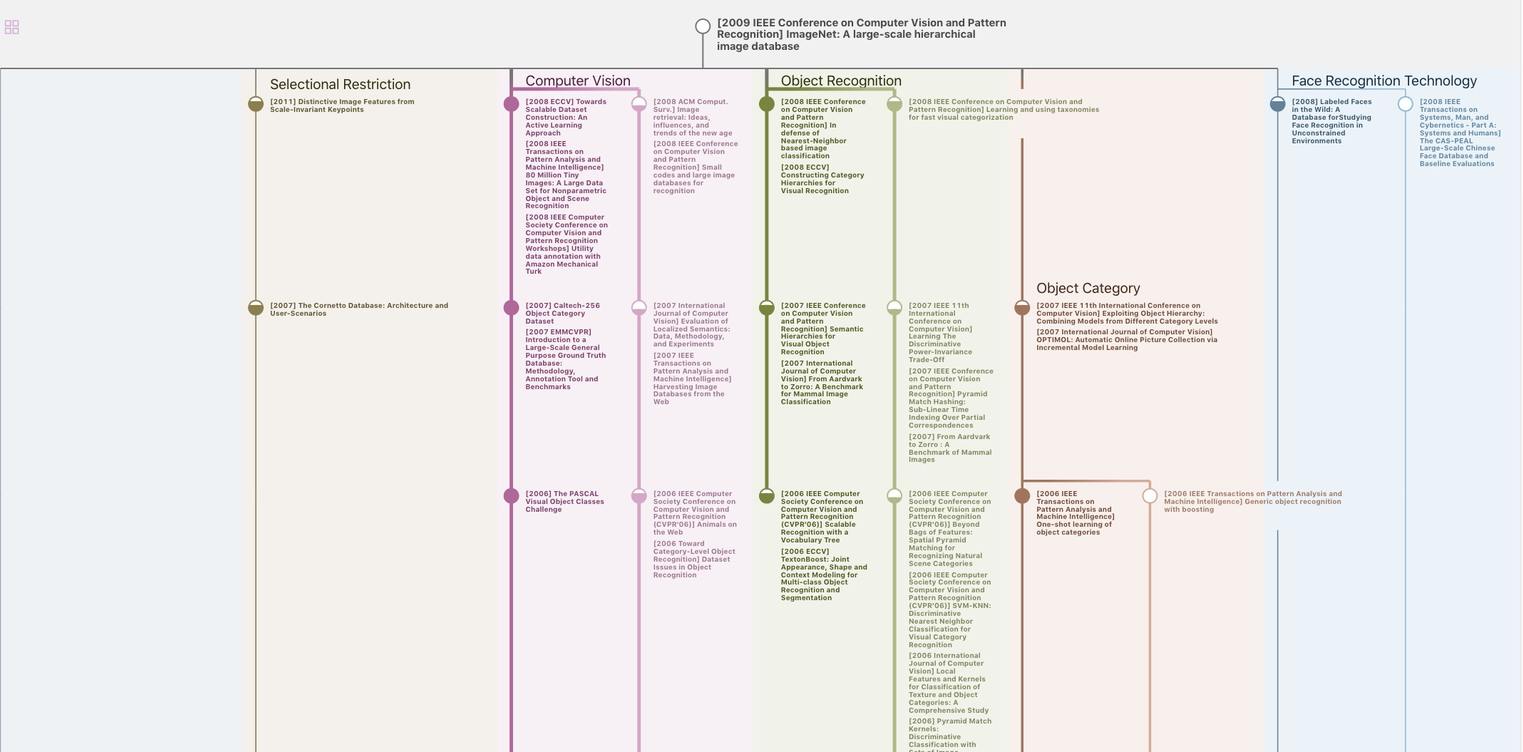
生成溯源树,研究论文发展脉络
Chat Paper
正在生成论文摘要