Enhancing Individual Fairness through Propensity Score Matching
2022 IEEE 9th International Conference on Data Science and Advanced Analytics (DSAA)(2022)
摘要
The central idea of individual fairness is based on an auspicious yet intuitive assertion: similar individuals should be treated similarly. Nevertheless, the fulfillment of individual fairness is hindered by three major obstacles. First, one needs to determine individuals who should receive similar treatment. Second, seamlessly formulating the notion of individual fairness in an ML learning process is another challenge. Third, effectively evaluating the notion of similar treatment in probabilistic classifiers is another challenge. To overcome these challenges, we propose a novel framework called FairMatch. Our proposed framework offers a new approach to pairing similar and dissimilar individuals using a causal analysis method called propensity score matching. Moreover, we formulate individual fairness as a representation learning problem where we incorporate similar and dissimilar pairs in a triplet-based loss function. Eventually, we devise a novel metric to evaluate individual fairness that captures the notion of similar treatment in probabilistic classifiers in a better way. Experimental results on four real-world datasets verify the superiority of FairMatch to existing solutions where we demonstrate it can deliver fairer decisions without scarifying the predictive performance.
更多查看译文
关键词
Fairness,Machine Learning,Individual Fairness,Propensity Score Matching,Triplet Loss
AI 理解论文
溯源树
样例
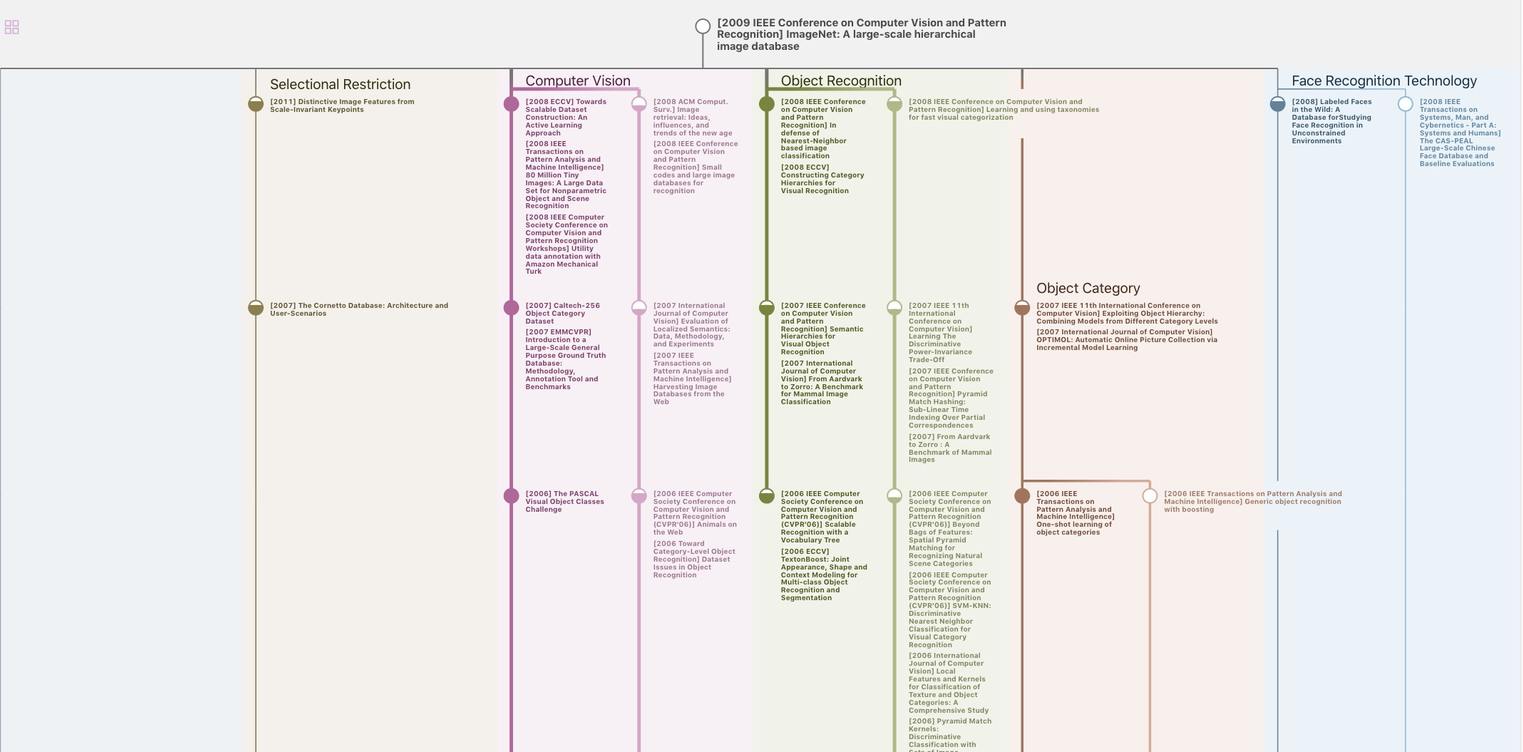
生成溯源树,研究论文发展脉络
Chat Paper
正在生成论文摘要