Detection of aspiration from images of a videofluoroscopic swallowing study adopting deep learning
Oral radiology(2023)
摘要
Objectives A videofluoroscopic swallowing study (VFSS) is conducted to detect aspiration. However, aspiration occurs within a short time and is difficult to detect. If deep learning can detect aspirations with high accuracy, clinicians can focus on the diagnosis of the detected aspirations. Whether VFSS aspirations can be classified using rapid-prototyping deep-learning tools was studied. Methods VFSS videos were separated into individual image frames. A region of interest was defined on the pharynx. Three convolutional neural networks (CNNs), namely a Simple-Layer CNN, Multiple-Layer CNN, and Modified LeNet, were designed for the classification. The performance results of the CNNs were compared in terms of the areas under their receiver-operating characteristic curves (AUCs). Results A total of 18,333 images obtained through data augmentation were selected for the evaluation. The different CNNs yielded sensitivities of 78.8%–87.6%, specificities of 91.9%–98.1%, and overall accuracies of 85.8%–91.7%. The AUC of 0.974 obtained for the Simple-Layer CNN and Modified LeNet was significantly higher than that obtained for the Multiple-Layer CNN (AUC of 0.936) ( p < 0.001). Conclusions The results of this study show that deep learning has potential for detecting aspiration with high accuracy.
更多查看译文
关键词
Videofluoroscopic swallowing study,Deep learning,Artificial intelligence,Aspiration,Deglutition disorders
AI 理解论文
溯源树
样例
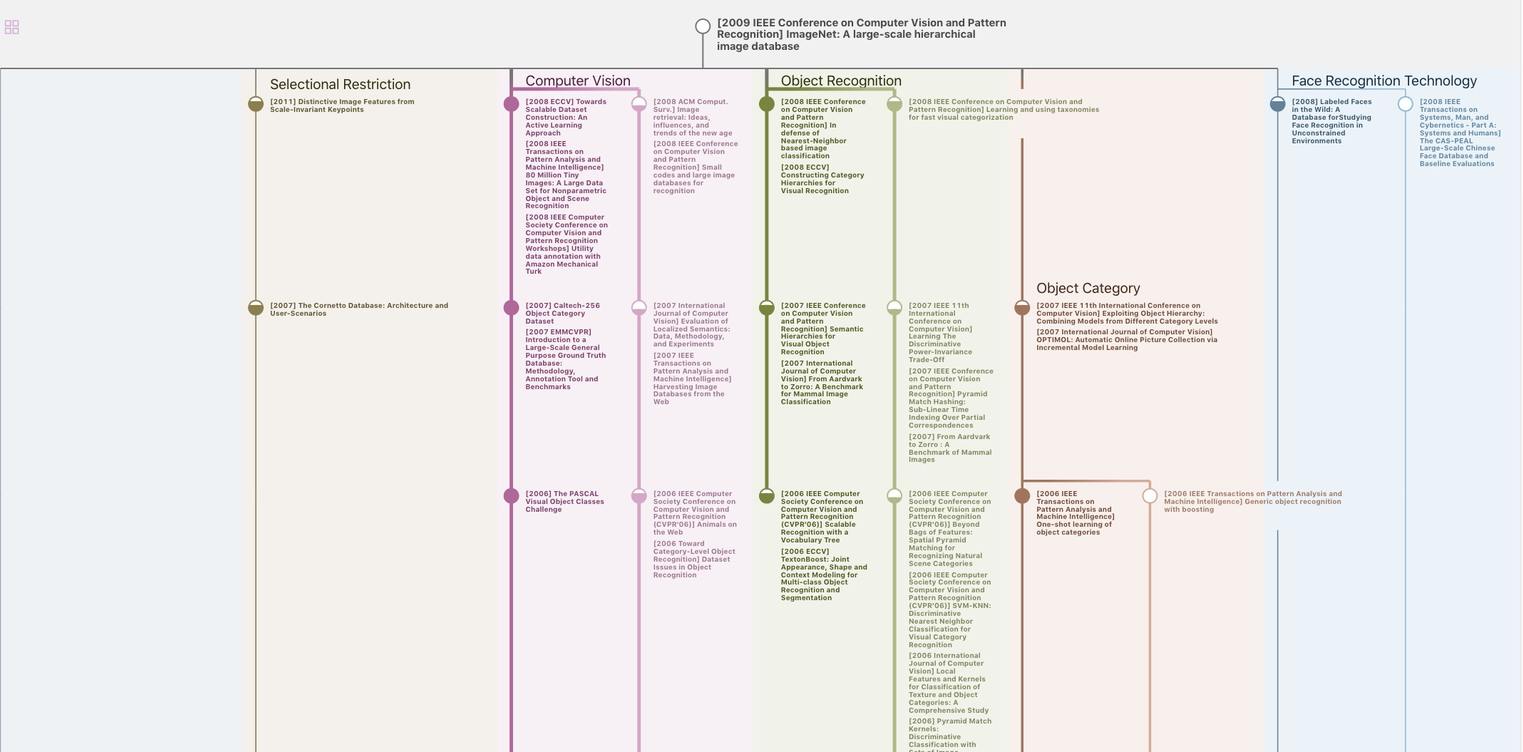
生成溯源树,研究论文发展脉络
Chat Paper
正在生成论文摘要