Transparent reporting of multivariable prediction models developed or validated using clustered data: TRIPOD-Cluster checklist.
BMJ (Clinical research ed.)(2023)
摘要
The increasing availability of large combined datasets (or big data), such as those from electronic health records and from individual participant data meta-analyses, provides new opportunities and challenges for researchers developing and validating (including updating) prediction models. These datasets typically include individuals from multiple clusters (such as multiple centres, geographical locations, or different studies). Accounting for clustering is important to avoid misleading conclusions and enables researchers to explore heterogeneity in prediction model performance across multiple centres, regions, or countries, to better tailor or match them to these different clusters, and thus to develop prediction models that are more generalisable. However, this requires prediction model researchers to adopt more specific design, analysis, and reporting methods than standard prediction model studies that do not have any inherent substantial clustering. Therefore, prediction model studies based on clustered data need to be reported differently so that readers can appraise the study methods and findings, further increasing the use and implementation of such prediction models developed or validated from clustered datasets.
更多查看译文
关键词
multivariable prediction models,transparent reporting,tripod-cluster
AI 理解论文
溯源树
样例
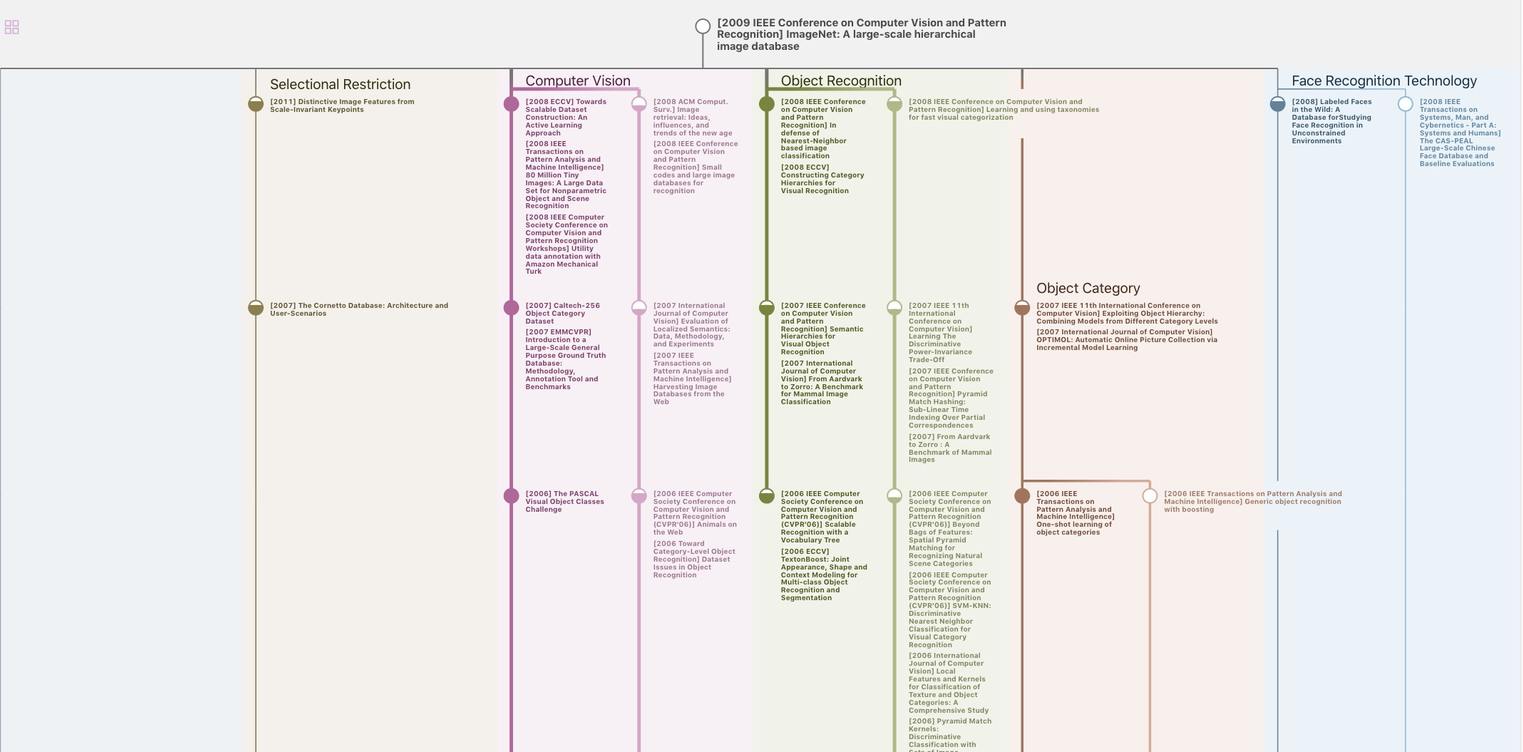
生成溯源树,研究论文发展脉络
Chat Paper
正在生成论文摘要