Fall detection from a manual wheelchair: preliminary findings based on accelerometers using machine learning techniques.
Assistive technology : the official journal of RESNA(2023)
摘要
Automated fall detection devices for individuals who use wheelchairs to minimize the consequences of falls are lacking. This study aimed to develop and train a fall detection algorithm to differentiate falls from wheelchair mobility activities using machine learning techniques. Thirty, healthy, ambulatory, young adults simulated falls from a wheelchair and performed other wheelchair-related mobility activities in a laboratory. Neural Network classifiers were used to train the algorithm developed based on data retrieved from accelerometers mounted at the participant's wrist, chest, and head. Results indicate excellent accuracy to differentiate between falls and wheelchair mobility activities. The sensors mounted at the wrist, chest, and head presented with an accuracy of 100%, 96.9%, and 94.8%, respectively, using data from 258 falls and 220 wheelchair mobility activities. This pilot study indicates that a fall detection algorithm developed in a laboratory setting based on fall accelerometer patterns can accurately differentiate wheelchair-related falls and wheelchair mobility activities. This algorithm should be integrated into a wrist-worn devices and tested among individuals who use a wheelchair in the community.
更多查看译文
关键词
accidental falls,activity recognition,fall detection,wearable sensor,wheelchair
AI 理解论文
溯源树
样例
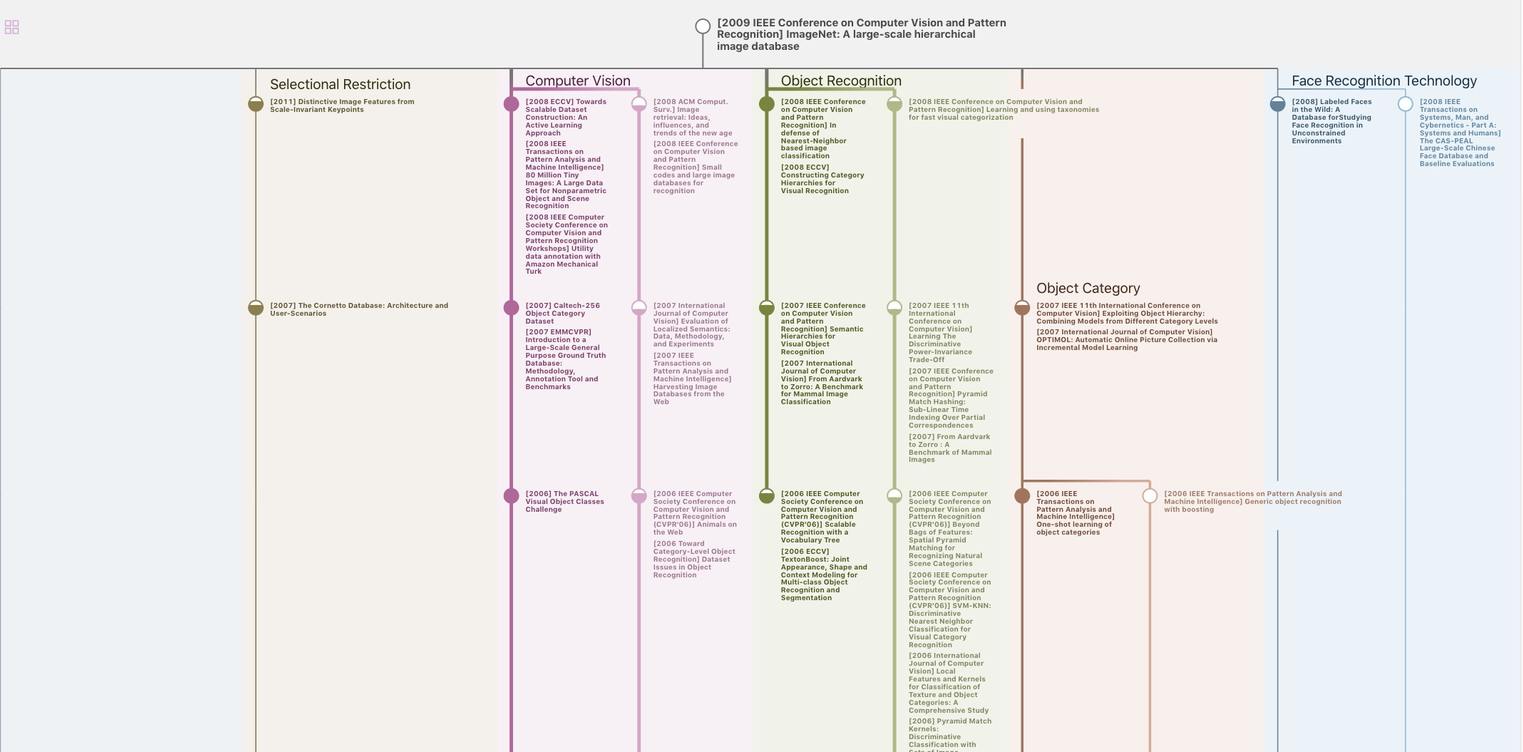
生成溯源树,研究论文发展脉络
Chat Paper
正在生成论文摘要