Tailored forecasts can predict extreme climate informing proactive interventions in East Africa
EARTHS FUTURE(2023)
摘要
This commentary discusses new advances in the predictability of east African rains and highlights the potential for improved early warning systems (EWS), humanitarian relief efforts, and agricultural decision-making. Following an unprecedented sequence of five droughts, in 2022 23 million east Africans faced starvation, requiring >$2 billion in aid. Here, we update climate attribution studies showing that these droughts resulted from an interaction of climate change and La Niña. Then we describe, for the first time, how attribution-based insights can be combined with the latest dynamic models to predict droughts at eight-month lead-times. We then discuss behavioral and social barriers to forecast use, and review literature examining how EWS might (or might not) enhance agro-pastoral advisories and humanitarian interventions. Finally, in reference to the new World Meteorological Organization (WMO) “Early Warning for All” plan, we conclude with a set of recommendations supporting actionable and authoritative climate services. Trust, urgency, and accuracy can help overcome barriers created by limited funding, uncertain tradeoffs, and inertia. Understanding how climate change is producing predictable climate extremes now, investing in African-led EWS, and building better links between EWS and agricultural development efforts can support long-term adaptation, reducing chronic needs for billions of dollars in reactive assistance. The main messages of this commentary will be widely. Climate change is interacting with La Niña to produce extreme, but extremely predictable, Pacific sea surface temperature gradients. These gradients will affect the climate in many countries creating opportunities for prediction. Effective use of such predictions, however, will demand cross-silo collaboration.
更多查看译文
关键词
climate,forecasts,interventions,africa
AI 理解论文
溯源树
样例
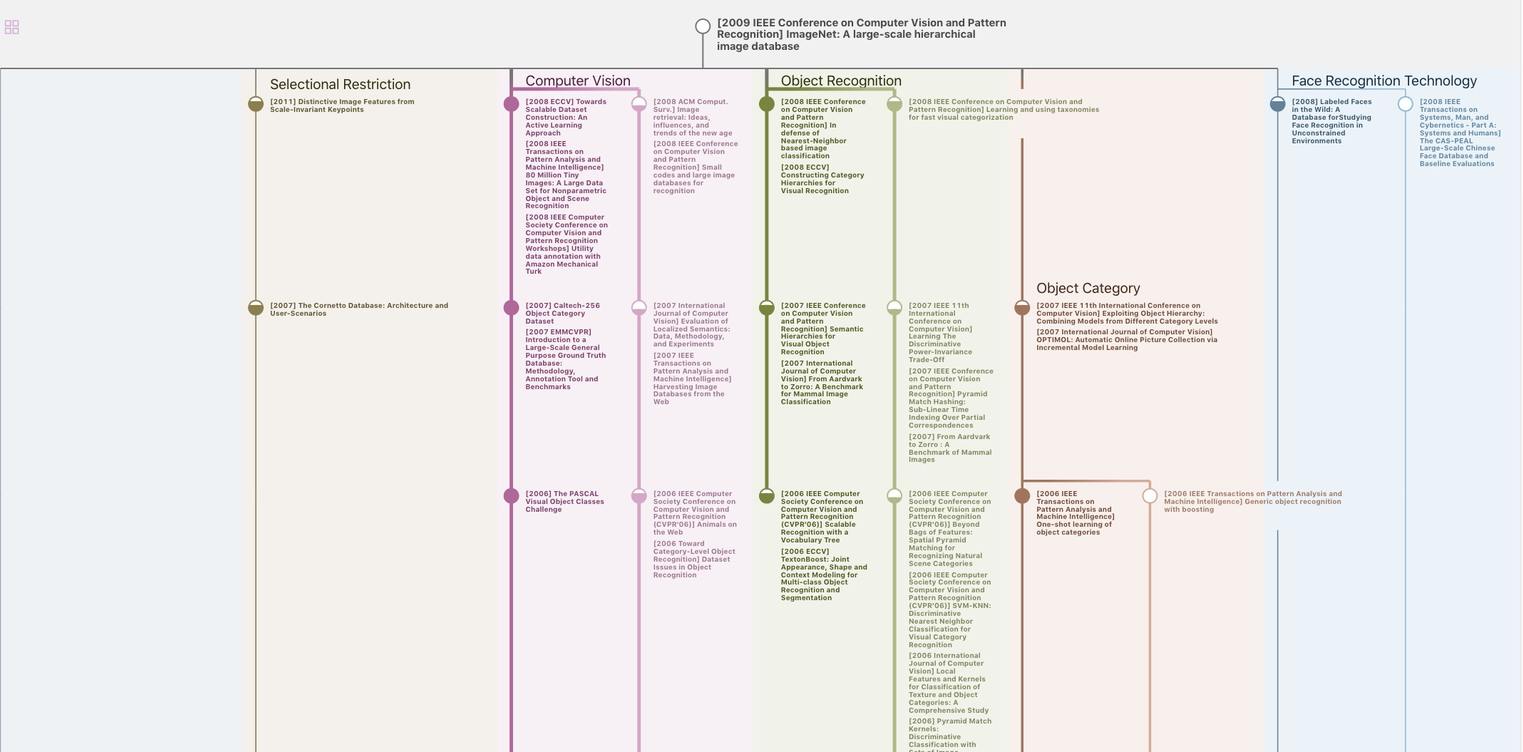
生成溯源树,研究论文发展脉络
Chat Paper
正在生成论文摘要