Masking Kernel for Learning Energy-Efficient Speech Representation
arxiv(2023)
摘要
Modern smartphones are equipped with powerful audio hardware and processors, allowing them to acquire and perform on-device speech processing at high sampling rates. However, energy consumption remains a concern, especially for resource-intensive DNNs. Prior mobile speech processing reduced computational complexity by compacting the model or reducing input dimensions via hyperparameter tuning, which reduced accuracy or required more training iterations. This paper proposes gradient descent for optimizing energy-efficient speech recording format (length and sampling rate). The goal is to reduce the input size, which reduces data collection and inference energy. For a backward pass, a masking function with non-zero derivatives (Gaussian, Hann, and Hamming) is used as a windowing function and a lowpass filter. An energy-efficient penalty is introduced to incentivize the reduction of the input size. The proposed masking outperformed baselines by 8.7% in speaker recognition and traumatic brain injury detection using 49% shorter duration, sampled at a lower frequency.
更多查看译文
关键词
kernel,speech,representation,energy-efficient
AI 理解论文
溯源树
样例
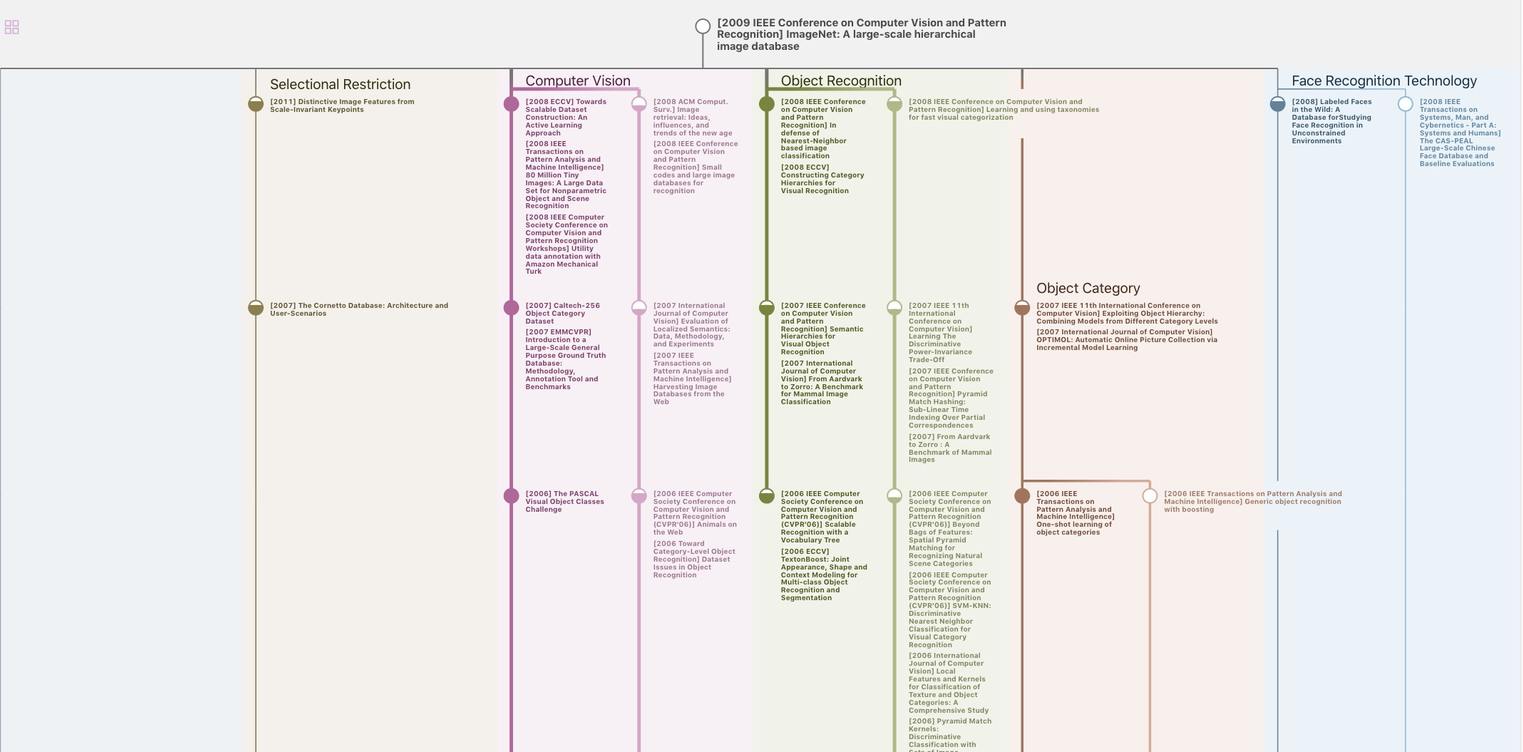
生成溯源树,研究论文发展脉络
Chat Paper
正在生成论文摘要