ASTRIDE: Adaptive Symbolization for Time Series Databases
arxiv(2023)
摘要
We introduce ASTRIDE (Adaptive Symbolization for Time seRIes DatabasEs), a novel symbolic representation of time series, along with its accelerated variant FASTRIDE (Fast ASTRIDE). Unlike most symbolization procedures, ASTRIDE is adaptive during both the segmentation step by performing change-point detection and the quantization step by using quantiles. Instead of proceeding signal by signal, ASTRIDE builds a dictionary of symbols that is common to all signals in a data set. We also introduce D-GED (Dynamic General Edit Distance), a novel similarity measure on symbolic representations based on the general edit distance. We demonstrate the performance of the ASTRIDE and FASTRIDE representations compared to SAX (Symbolic Aggregate approXimation), 1d-SAX, SFA (Symbolic Fourier Approximation), and ABBA (Adaptive Brownian Bridge-based Aggregation) on reconstruction and, when applicable, on classification tasks. These algorithms are evaluated on 86 univariate equal-size data sets from the UCR Time Series Classification Archive. An open source GitHub repository called astride is made available to reproduce all the experiments in Python.
更多查看译文
关键词
adaptive symbolization,time series,databases
AI 理解论文
溯源树
样例
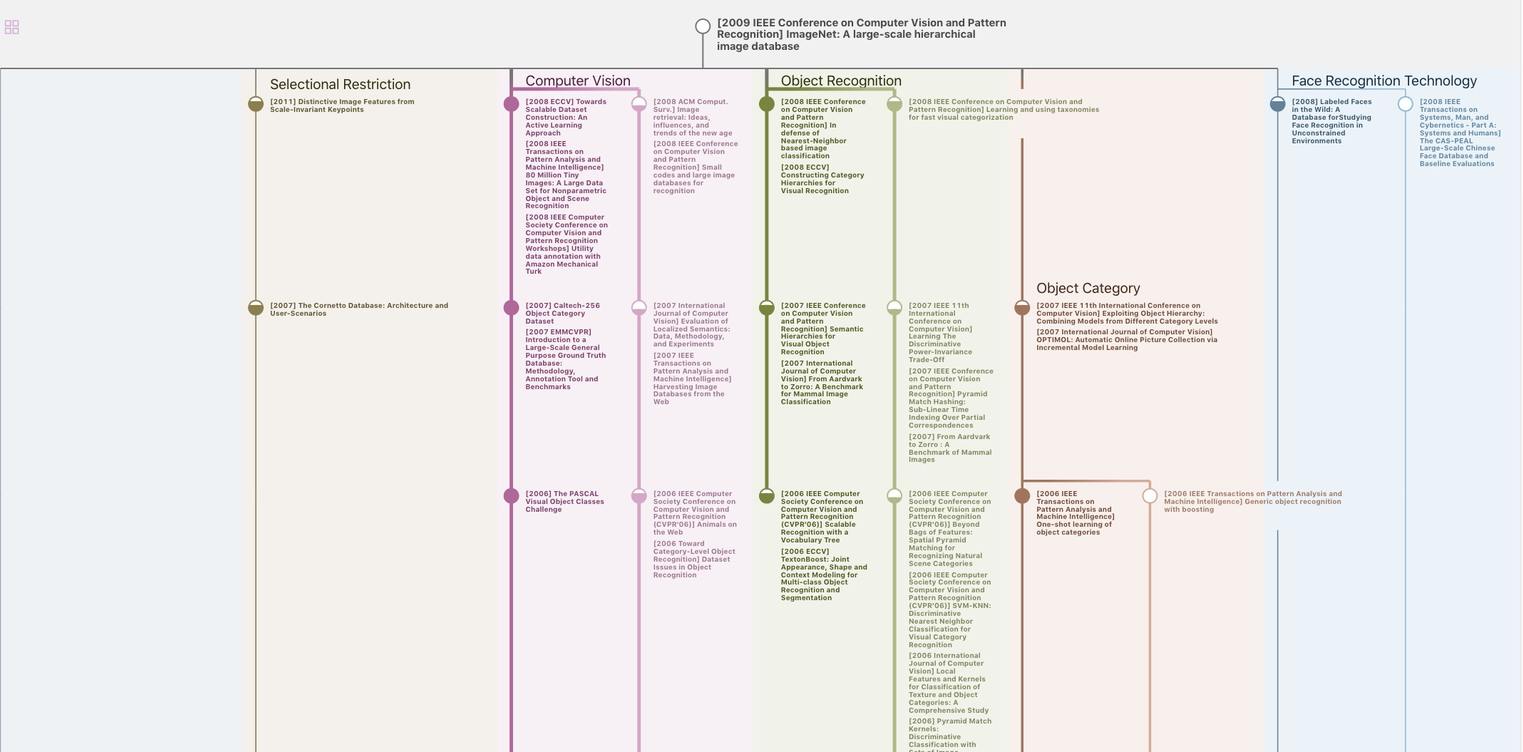
生成溯源树,研究论文发展脉络
Chat Paper
正在生成论文摘要