Learning Pairwise Interaction for Generalizable DeepFake Detection
2023 IEEE/CVF Winter Conference on Applications of Computer Vision Workshops (WACVW)(2023)
摘要
A fast-paced development of DeepFake generation techniques challenge the detection schemes designed for known type DeepFakes. A reliable Deepfake detection approach must be agnostic to generation types, which can present diverse quality and appearance. Limited generalizability across different generation schemes will restrict the wide-scale deployment of detectors if they fail to handle unseen attacks in an open set scenario. We propose a new approach, Multi-Channel Xception Attention Pairwise Interaction (MCX-API), that exploits the power of pairwise learning and complementary information from different color space representations in a fine-grained manner. We first validate our idea on a publicly available dataset in a intra-class setting (closed set) with four different Deepfake schemes. Further, we report all the results using balanced-open-set-classification (BOSC) accuracy in an inter-class setting (open-set) using three public datasets. Our experiments indicate that our proposed method can generalize better than the state-of-the-art Deepfakes detectors. We obtain 98.48% BOSC accuracy on the
$FF++$
dataset and 90.87% BOSC accuracy on the CelebDF dataset suggesting a promising direction for generalization of DeepFake detection. We further utilize t-SNE and attention maps to interpret and visualize the decision-making process of our proposed network.
更多查看译文
关键词
pairwise interaction,detection
AI 理解论文
溯源树
样例
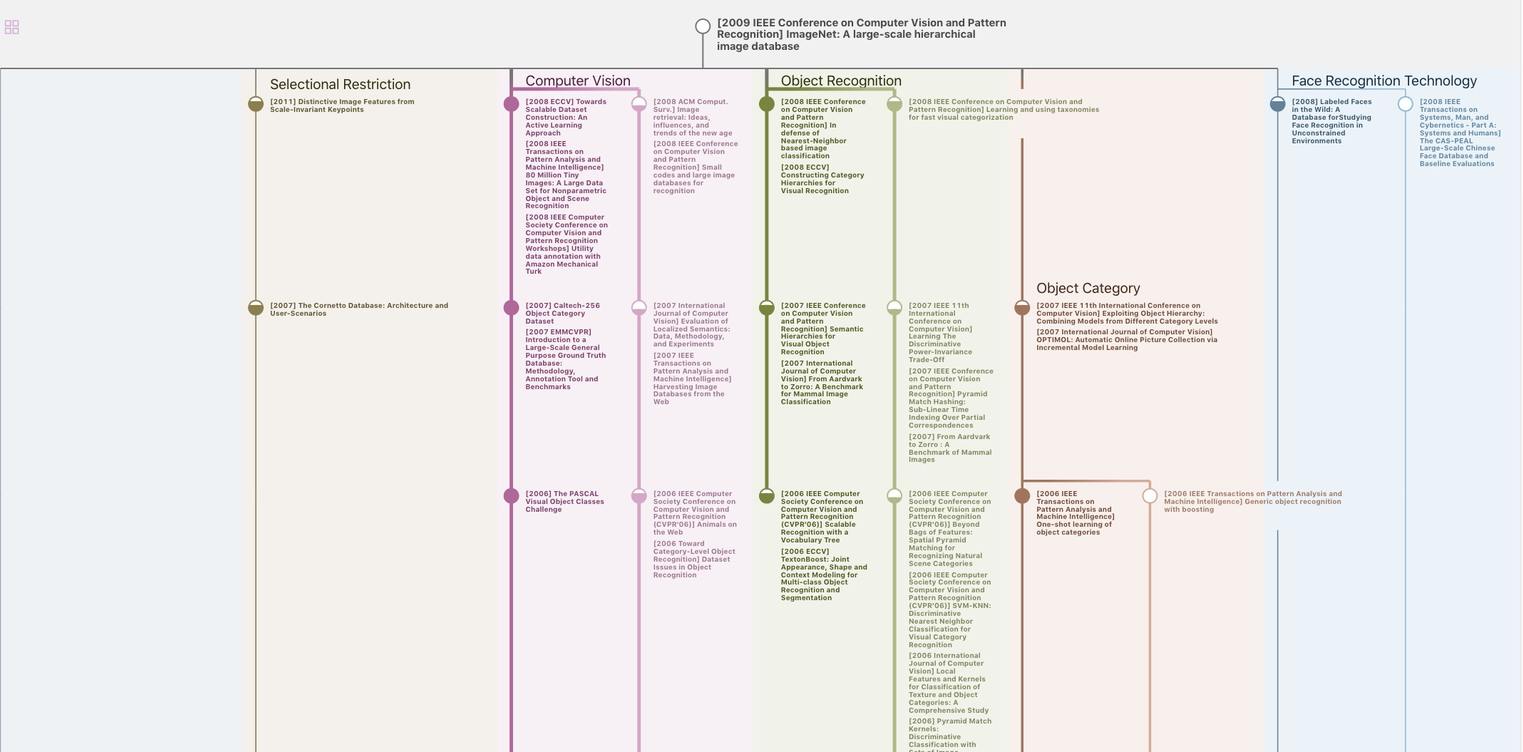
生成溯源树,研究论文发展脉络
Chat Paper
正在生成论文摘要