EMOD: Efficient Moving Object Detection via Image Eccentricity Analysis and Sparse Neural Networks
2023 IEEE/CVF Winter Conference on Applications of Computer Vision Workshops (WACVW)(2023)
摘要
This paper proposes an efficient moving objects detection pipeline focusing on dynamic object detection on video streams captured by traffic monitoring cameras. While developing autonomous vehicle systems, we found that views from self-driving vehicles can be occluded by dynamic or static objects on the street. Whereas infrastructure nodes such as traffic monitoring cameras having broader field-of-views and better perspectives can be used as auxiliary sensors to share traffic information with nearby self-driving cars in real-time. However, these infrastructure cameras usually have constrained computation resources, and detecting hundreds of static background objects in consecutive video frames is wasteful. In our detection pipeline, we leverage the image eccentricity analysis as a pre-processing step to fast generate moving objects segmentation maps. These maps are used to mask the original images to get images that only contain the moving objects in the scene. These sparse images are then passed to an object detection model built with a sparse convolution backbone network, resulting in significant reduction in computational costs. Our quantitative experiments illustrate that the proposed detection pipeline can achieve up to 50% inference speedup with negligible detection accuracy drop in images obtained from traffic monitoring cameras.
更多查看译文
AI 理解论文
溯源树
样例
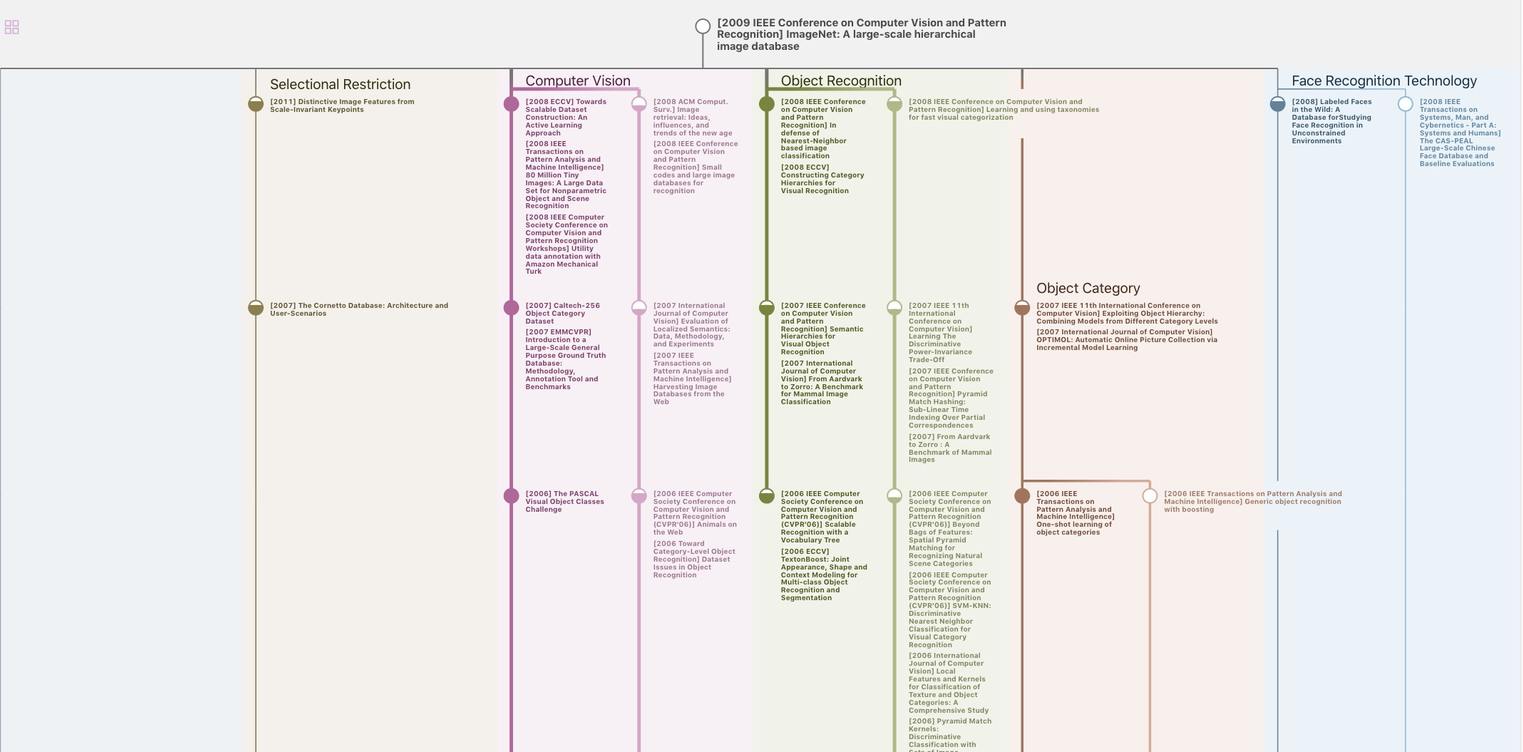
生成溯源树,研究论文发展脉络
Chat Paper
正在生成论文摘要