Short-Term Load Forecasting with Temporal Fusion Transformers for Power Distribution Networks
2022 IEEE Sustainable Power and Energy Conference (iSPEC)(2022)
摘要
Short-Term Load Forecasting (STLF) is essential to the operation and management of modern power distribution networks. Accurate STLF can significantly improve the demand-side management of the power system. In this paper, a new method with high-performance forecasting performance is presented to forecast short-term loads with deep learning. The temporal fusion transformers (TFT) approach is an attention-based deep learning model with interpretable insights into temporal dynamics. The sequence-to-sequence model processes the historical and future covariates to enhance the forecasting performance. Gated Residual Network (GRN) is applied to drop out unnecessary information and improve efficiency. The proposed method is tested on anonymized data from a university campus. The anomalies and missing data are imputed with the k-nearest neighbor (KNN) method. The testing results demonstrate the effectiveness of the proposed method.
更多查看译文
关键词
deep learning,temporal fusion transformer,load forecasting,time series forecasting.
AI 理解论文
溯源树
样例
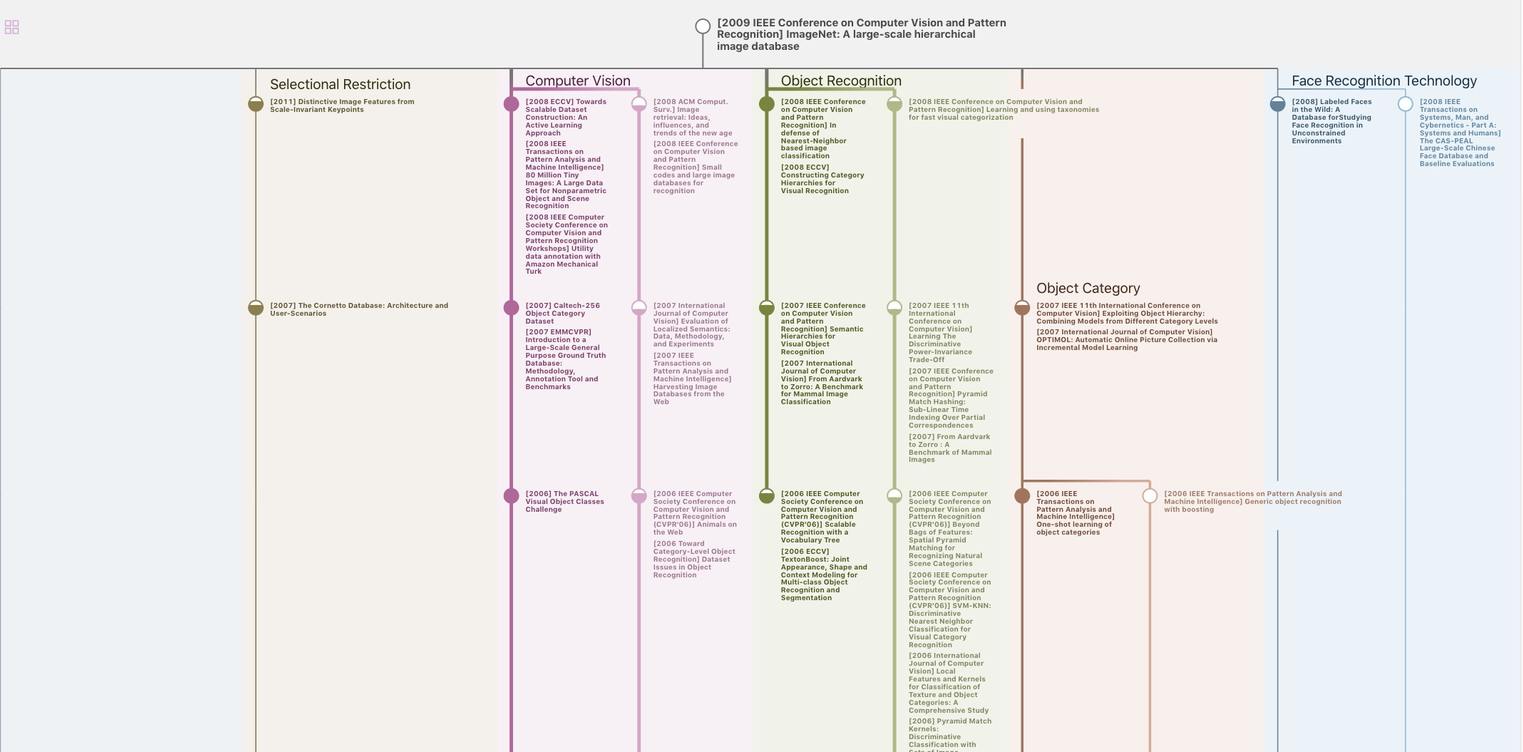
生成溯源树,研究论文发展脉络
Chat Paper
正在生成论文摘要