Evaluating a Learned Admission-Prediction Model as a Replacement for Standardized Tests in College Admissions
PROCEEDINGS OF THE TENTH ACM CONFERENCE ON LEARNING @ SCALE, L@S 2023(2023)
摘要
A growing number of college applications has presented an annual challenge for college admissions in the United States. Admission offices have historically relied on standardized test scores to organize large applicant pools into viable subsets for review. However, this approach may be subject to bias in test scores and selection bias in test-taking with recent trends toward test-optional admission. We explore a machine learning-based approach to replace the role of standardized tests in subset generation while taking into account a wide range of factors extracted from student applications to support a more holistic review. We evaluate the approach on data from an undergraduate admission office at a selective US institution (13,248 applications). We find that a prediction model trained on past admission data outperforms an SAT-based heuristic and matches the demographic composition of the last admitted class. We discuss the risks and opportunities for how such a learned model could be leveraged to support human decision-making in college admissions.
更多查看译文
关键词
higher education,college admissions,machine learning,predictive modeling,standardized testing
AI 理解论文
溯源树
样例
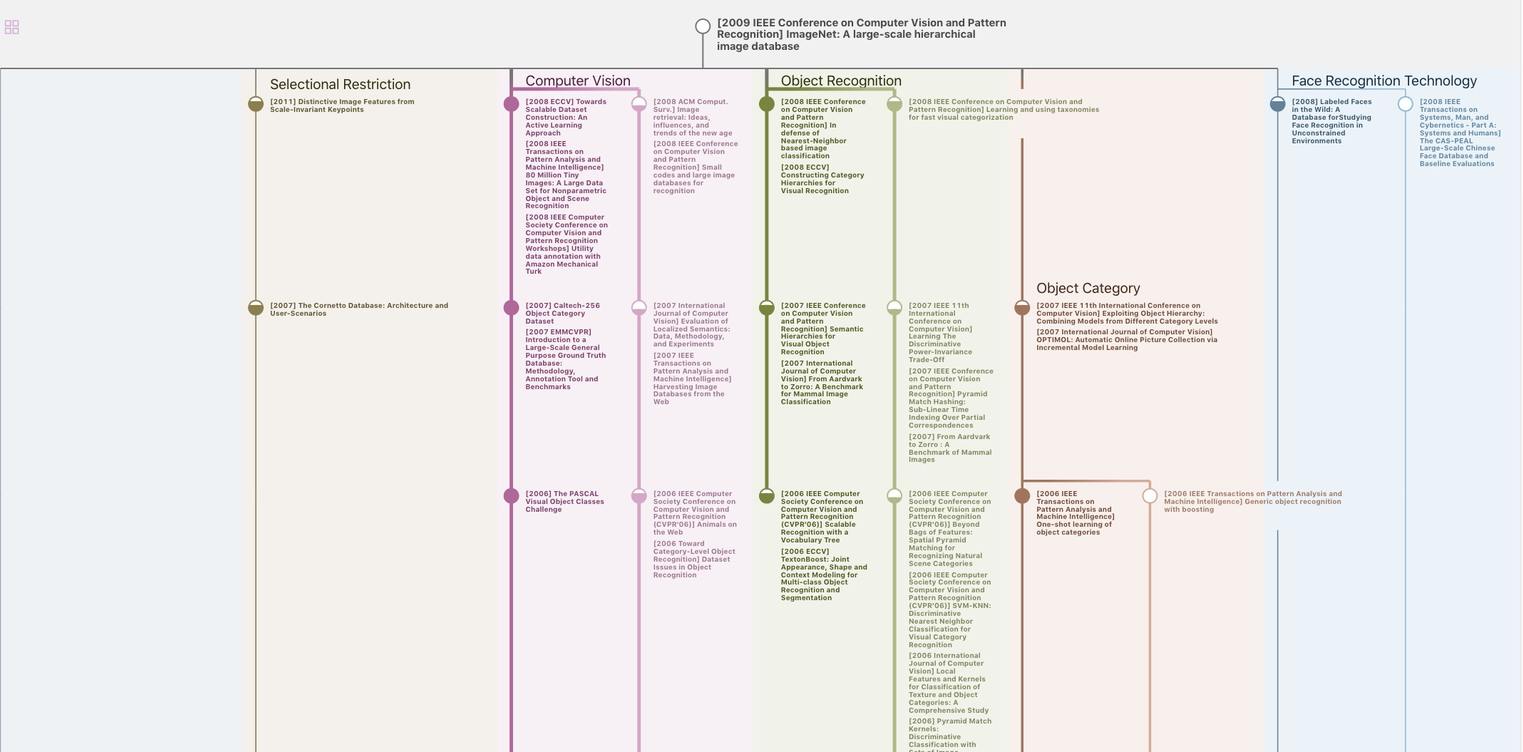
生成溯源树,研究论文发展脉络
Chat Paper
正在生成论文摘要