Look around and learn: self-improving object detection by exploration
arxiv(2023)
摘要
Object detectors often experience a drop in performance when new environmental conditions are insufficiently represented in the training data. This paper studies how to automatically fine-tune a pre-existing object detector while exploring and acquiring images in a new environment without relying on human intervention, i.e., in an utterly self-supervised fashion. In our setting, an agent initially learns to explore the environment using a pre-trained off-the-shelf detector to locate objects and associate pseudo-labels. By assuming that pseudo-labels for the same object must be consistent across different views, we learn an exploration policy mining hard samples and we devise a novel mechanism for producing refined predictions from the consensus among observations. Our approach outperforms the current state-of-the-art, and it closes the performance gap against a fully supervised setting without relying on ground-truth annotations. We also compare various exploration policies for the agent to gather more informative observations. Code and dataset will be made available upon paper acceptance
更多查看译文
关键词
object detection,exploration,look,self-improving
AI 理解论文
溯源树
样例
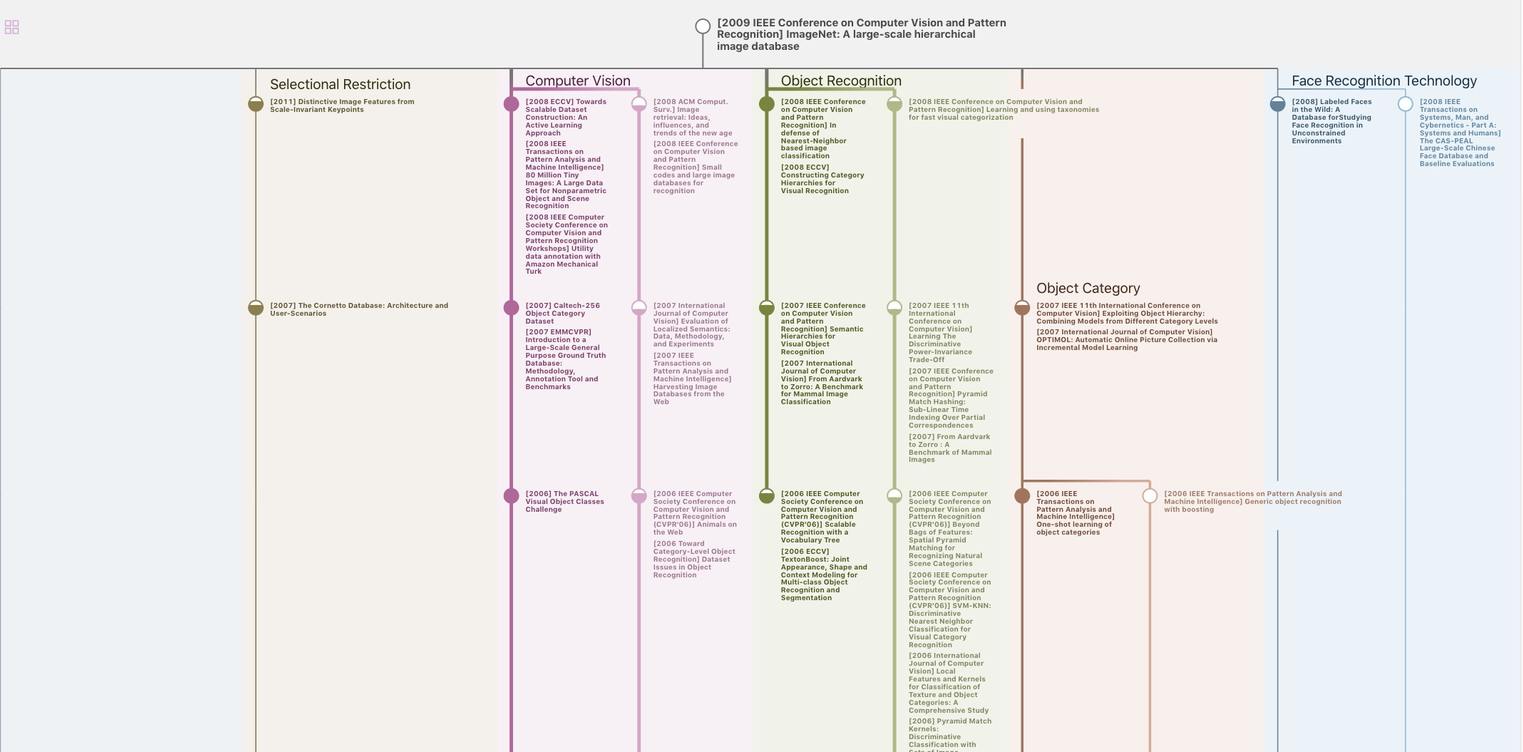
生成溯源树,研究论文发展脉络
Chat Paper
正在生成论文摘要