Cluster-Level Contrastive Learning for Emotion Recognition in Conversations
IEEE TRANSACTIONS ON AFFECTIVE COMPUTING(2023)
摘要
A key challenge for Emotion Recognition in Conversations (ERC) is to distinguish semantically similar emotions. Some works utilise Supervised Contrastive Learning (SCL) which uses categorical emotion labels as supervision signals and contrasts in high-dimensional semantic space. However, categorical labels fail to provide quantitative information between emotions. ERC is also not equally dependent on all embedded features in the semantic space, which makes the high-dimensional SCL inefficient. To address these issues, we propose a novel low-dimensional Supervised Cluster-level Contrastive Learning (SCCL) method, which first reduces the high-dimensional SCL space to a three-dimensional affect representation space Valence-Arousal-Dominance (VAD), then performs cluster-level contrastive learning to incorporate measurable emotion prototypes. To help modelling the dialogue and enriching the context, we leverage the pre-trained knowledge adapters to infuse linguistic and factual knowledge. Experiments show that our method achieves new state-of-the-art results with 69.81% on IEMOCAP, 65.7% on MELD, and 62.51% on DailyDialog datasets. The analysis also proves that the VAD space is not only suitable for ERC but also interpretable, with VAD prototypes enhancing its performance and stabilising the training of SCCL. In addition, the pre-trained knowledge adapters benefit the performance of the utterance encoder and SCCL. Our code is available at: https://github.com/SteveKGYang/SCCLI
更多查看译文
关键词
Emotion recognition,Prototypes,Linguistics,Task analysis,Semantics,Training,Adaptation models,Cluster-level contrastive learning,emotion recognition in conversations,pre-trained knowledge adapters,valence-arousal-dominance
AI 理解论文
溯源树
样例
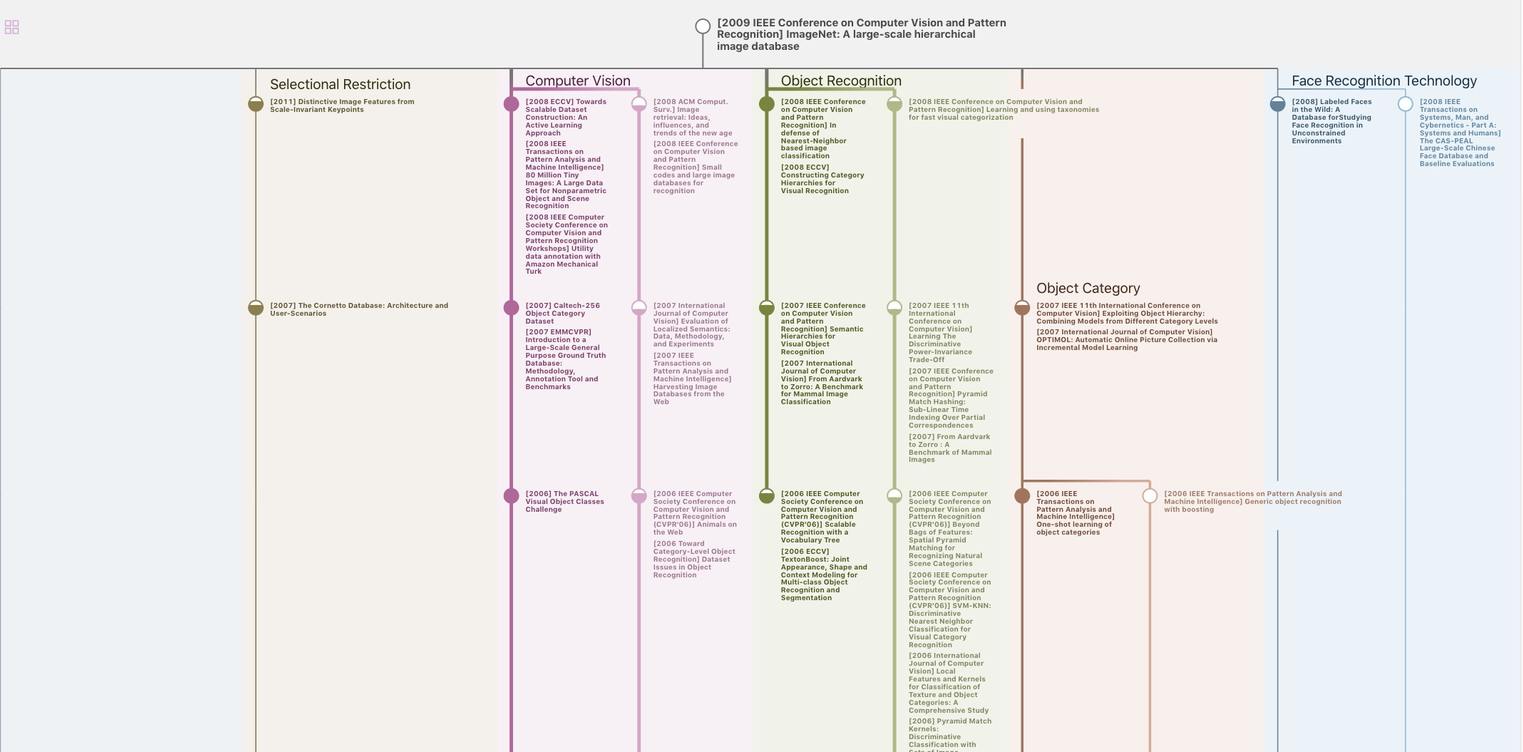
生成溯源树,研究论文发展脉络
Chat Paper
正在生成论文摘要