Spatial Functa: Scaling Functa to ImageNet Classification and Generation
arxiv(2023)
摘要
Neural fields, also known as implicit neural representations, have emerged as a powerful means to represent complex signals of various modalities. Based on this Dupont et al. (2022) introduce a framework that views neural fields as data, termed *functa*, and proposes to do deep learning directly on this dataset of neural fields. In this work, we show that the proposed framework faces limitations when scaling up to even moderately complex datasets such as CIFAR-10. We then propose *spatial functa*, which overcome these limitations by using spatially arranged latent representations of neural fields, thereby allowing us to scale up the approach to ImageNet-1k at 256x256 resolution. We demonstrate competitive performance to Vision Transformers (Steiner et al., 2022) on classification and Latent Diffusion (Rombach et al., 2022) on image generation respectively.
更多查看译文
关键词
spatial functa,imagenet classification,scaling
AI 理解论文
溯源树
样例
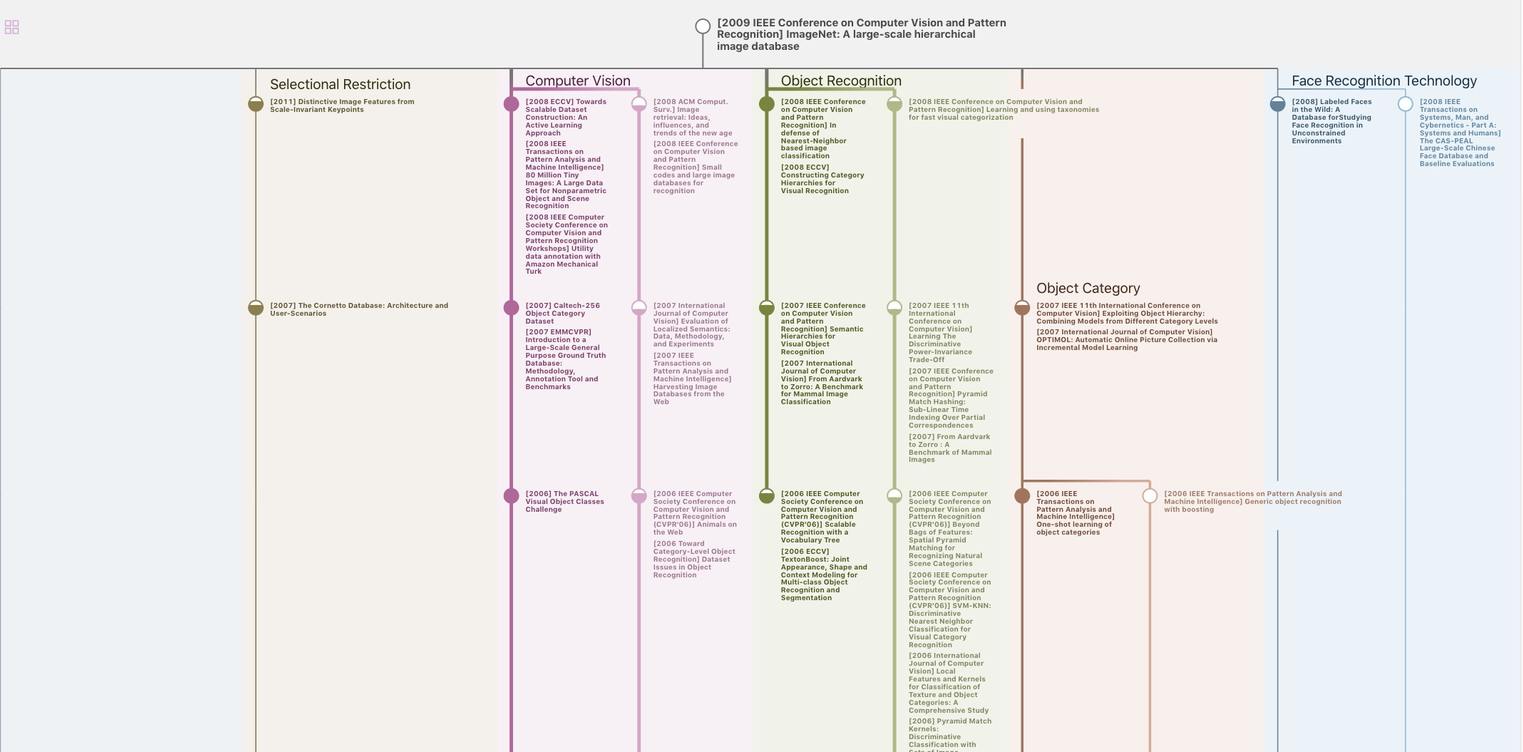
生成溯源树,研究论文发展脉络
Chat Paper
正在生成论文摘要