In Search of Insights, Not Magic Bullets: Towards Demystification of the Model Selection Dilemma in Heterogeneous Treatment Effect Estimation
arxiv(2023)
摘要
Personalized treatment effect estimates are often of interest in high-stakes applications -- thus, before deploying a model estimating such effects in practice, one needs to be sure that the best candidate from the ever-growing machine learning toolbox for this task was chosen. Unfortunately, due to the absence of counterfactual information in practice, it is usually not possible to rely on standard validation metrics for doing so, leading to a well-known model selection dilemma in the treatment effect estimation literature. While some solutions have recently been investigated, systematic understanding of the strengths and weaknesses of different model selection criteria is still lacking. In this paper, instead of attempting to declare a global `winner', we therefore empirically investigate success- and failure modes of different selection criteria. We highlight that there is a complex interplay between selection strategies, candidate estimators and the DGP used for testing, and provide interesting insights into the relative (dis)advantages of different criteria alongside desiderata for the design of further illuminating empirical studies in this context.
更多查看译文
关键词
heterogeneous treatment effect estimation,model selection dilemma
AI 理解论文
溯源树
样例
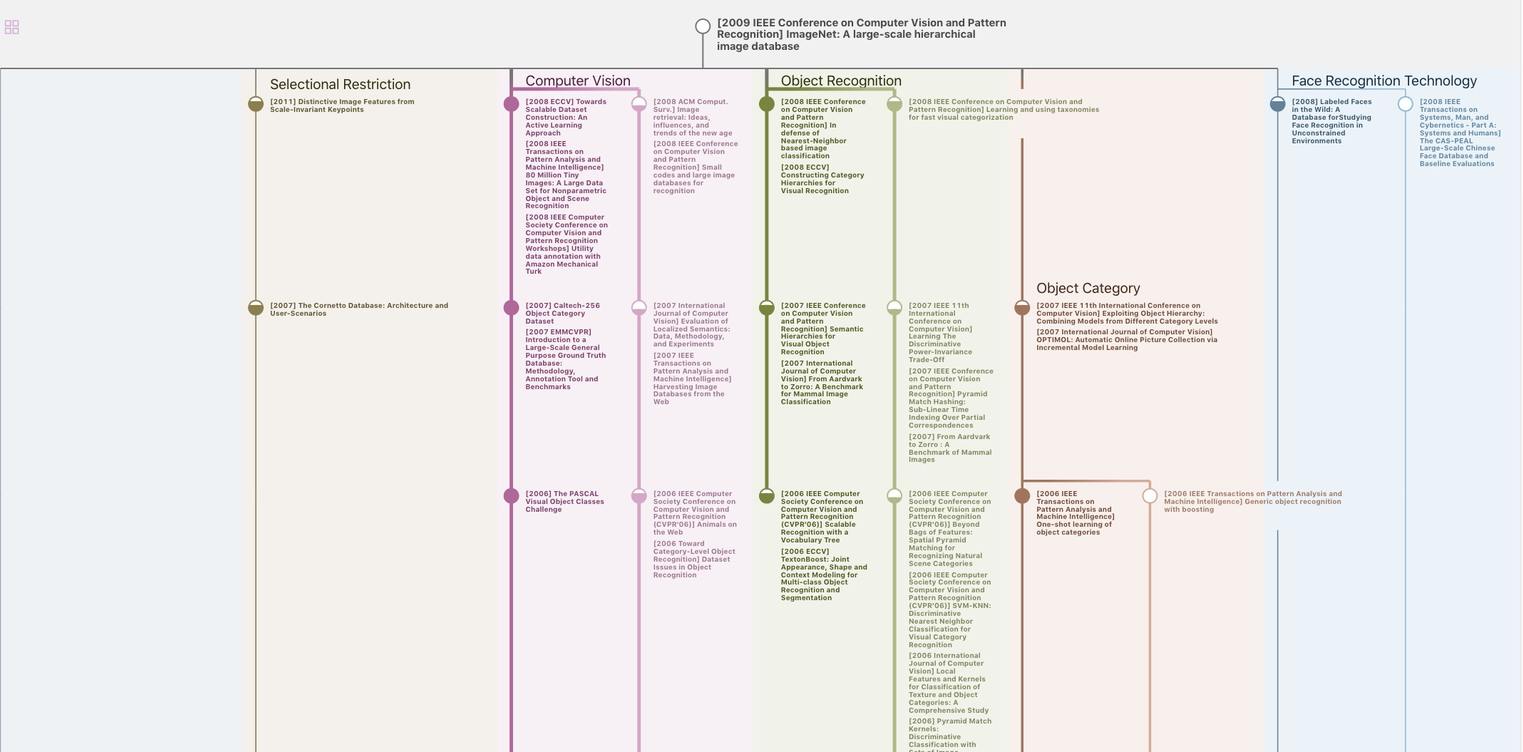
生成溯源树,研究论文发展脉络
Chat Paper
正在生成论文摘要