Online Mechanism Design for Information Acquisition
arxiv(2023)
摘要
We study the problem of designing mechanisms for \emph{information acquisition} scenarios. This setting models strategic interactions between an uniformed \emph{receiver} and a set of informed \emph{senders}. In our model the senders receive information about the underlying state of nature and communicate their observation (either truthfully or not) to the receiver, which, based on this information, selects an action. Our goal is to design mechanisms maximizing the receiver's utility while incentivizing the senders to report truthfully their information. First, we provide an algorithm that efficiently computes an optimal \emph{incentive compatible} (IC) mechanism. Then, we focus on the \emph{online} problem in which the receiver sequentially interacts in an unknown game, with the objective of minimizing the \emph{cumulative regret} w.r.t. the optimal IC mechanism, and the \emph{cumulative violation} of the incentive compatibility constraints. We investigate two different online scenarios, \emph{i.e.,} the \emph{full} and \emph{bandit feedback} settings. For the full feedback problem, we propose an algorithm that guarantees $\tilde{\mathcal O}(\sqrt T)$ regret and violation, while for the bandit feedback setting we present an algorithm that attains $\tilde{\mathcal O}(T^{\alpha})$ regret and $\tilde{\mathcal O}(T^{1-\alpha/2})$ violation for any $\alpha\in[1/2, 1]$. Finally, we complement our results providing a tight lower bound.
更多查看译文
关键词
information acquisition,online mechanism design
AI 理解论文
溯源树
样例
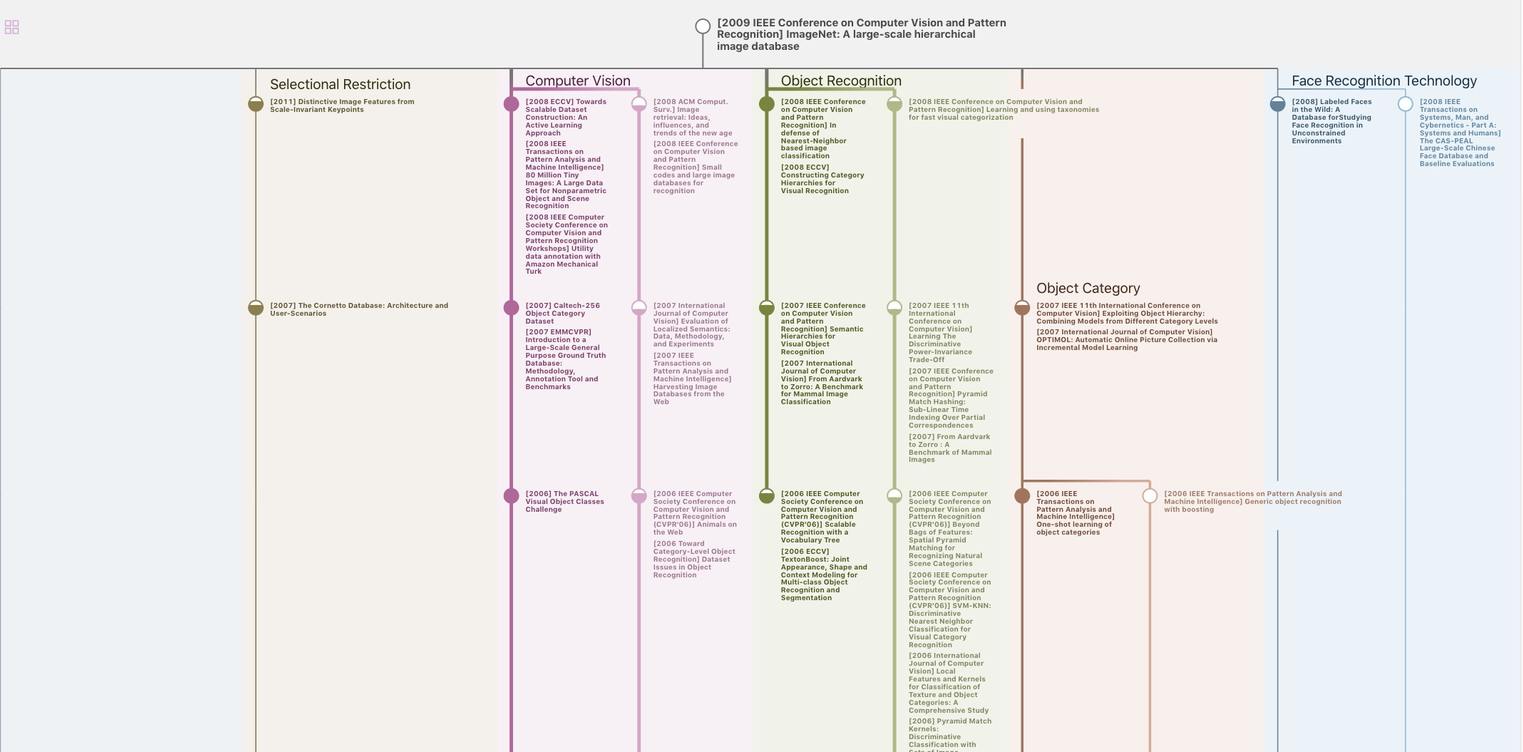
生成溯源树,研究论文发展脉络
Chat Paper
正在生成论文摘要