Network Autoregression for Incomplete Matrix-Valued Time Series
arxiv(2023)
摘要
We study the dynamics of matrix-valued time series with observed network structures by proposing a matrix network autoregression model with row and column networks of the subjects. We incorporate covariate information and a low rank intercept matrix. We allow incomplete observations in the matrices and the missing mechanism can be covariate dependent. To estimate the model, a two-step estimation procedure is proposed. The first step aims to estimate the network autoregression coefficients, and the second step aims to estimate the regression parameters, which are matrices themselves. Theoretically, we first separately establish the asymptotic properties of the autoregression coefficients and the error bounds of the regression parameters. Subsequently, a bias reduction procedure is proposed to reduce the asymptotic bias and the theoretical property of the debiased estimator is studied. Lastly, we illustrate the usefulness of the proposed method through a number of numerical studies and an analysis of a Yelp data set.
更多查看译文
关键词
network,matrix-valued
AI 理解论文
溯源树
样例
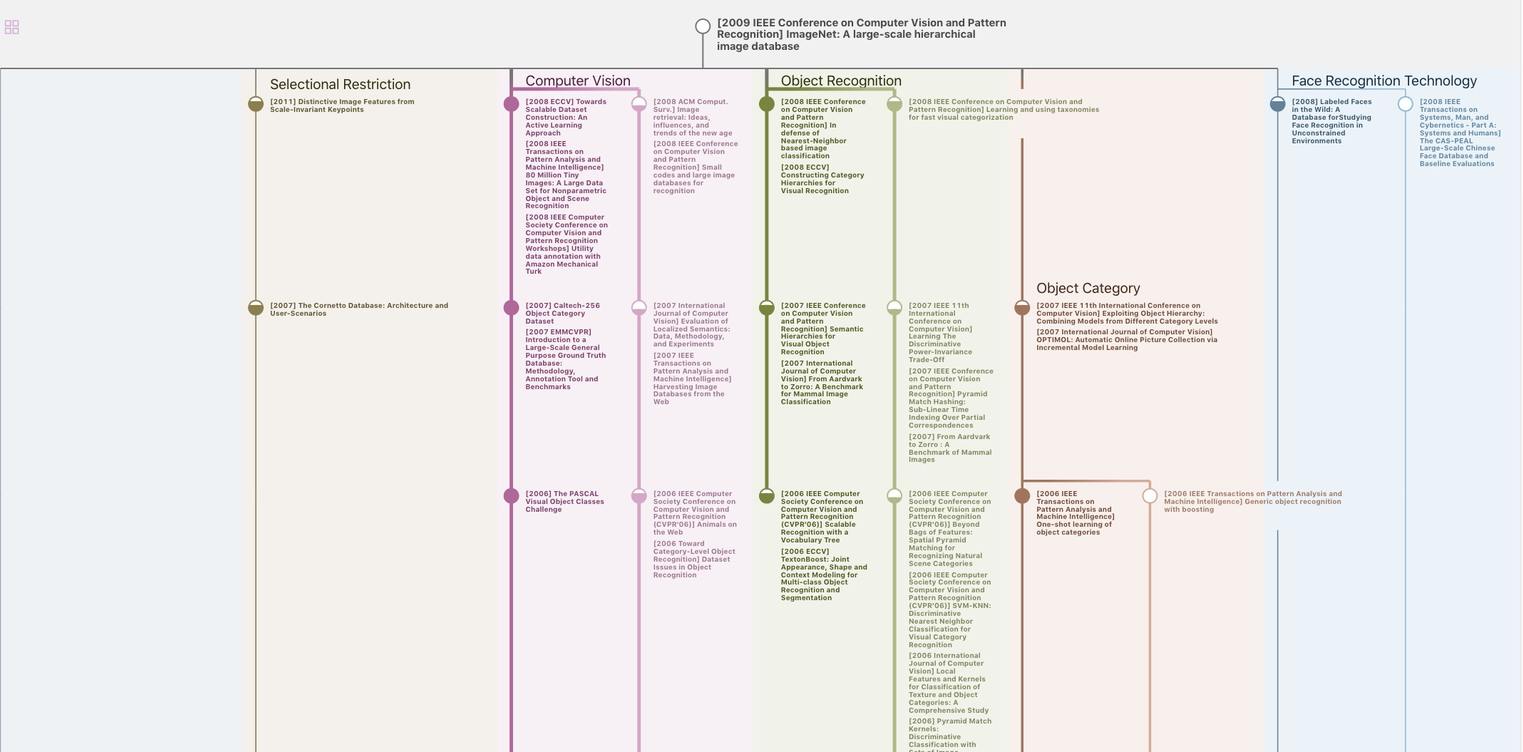
生成溯源树,研究论文发展脉络
Chat Paper
正在生成论文摘要