Clarifying Trust of Materials Property Predictions using Neural Networks with Distribution-Specific Uncertainty Quantification
arxiv(2023)
摘要
It is critical that machine learning (ML) model predictions be trustworthy for high-throughput catalyst discovery approaches. Uncertainty quantification (UQ) methods allow estimation of the trustworthiness of an ML model, but these methods have not been well explored in the field of heterogeneous catalysis. Herein, we investigate different UQ methods applied to a crystal graph convolutional neural network (CGCNN) to predict adsorption energies of molecules on alloys from the Open Catalyst 2020 (OC20) dataset, the largest existing heterogeneous catalyst dataset. We apply three UQ methods to the adsorption energy predictions, namely k-fold ensembling, Monte Carlo dropout, and evidential regression. The effectiveness of each UQ method is assessed based on accuracy, sharpness, dispersion, calibration, and tightness. Evidential regression is demonstrated to be a powerful approach for rapidly obtaining tunable, competitively trustworthy UQ estimates for heterogeneous catalysis applications when using neural networks. Recalibration of model uncertainties is shown to be essential in practical screening applications of catalysts using uncertainties.
更多查看译文
关键词
materials property predictions,neural networks,uncertainty,distribution-specific
AI 理解论文
溯源树
样例
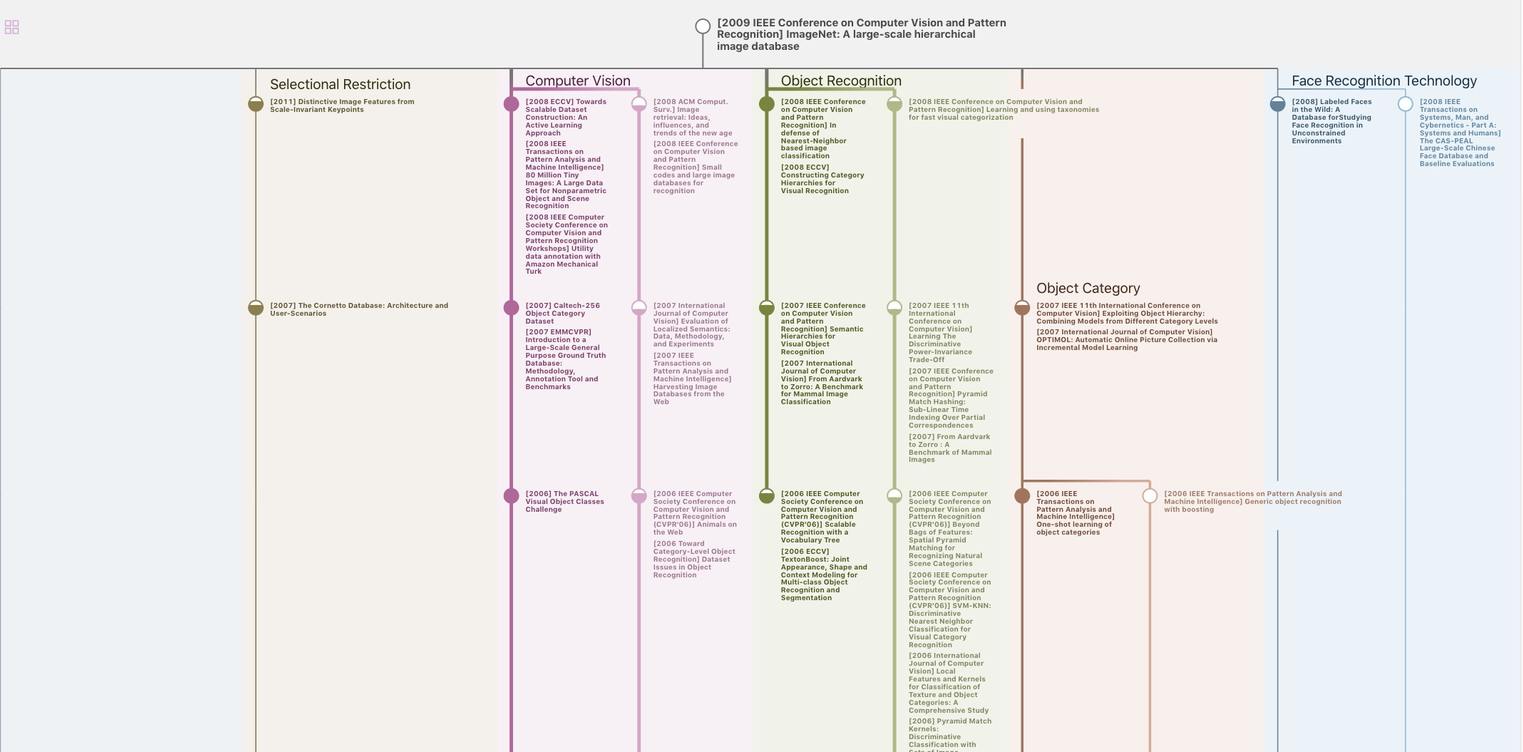
生成溯源树,研究论文发展脉络
Chat Paper
正在生成论文摘要