Exploiting Partial Common Information Microstructure for Multi-modal Brain Tumor Segmentation
MACHINE LEARNING FOR MULTIMODAL HEALTHCARE DATA, ML4MHD 2023(2024)
摘要
Learning with multiple modalities is crucial for automated brain tumor segmentation from magnetic resonance imaging data. Explicitly optimizing the common information shared among all modalities (e.g., by maximizing the total correlation) has been shown to achieve better feature representations and thus enhance the segmentation performance. However, existing approaches are oblivious to partial common information shared by subsets of the modalities. In this paper, we show that identifying such partial common information can significantly boost the discriminative power of image segmentation models. In particular, we introduce a novel concept of partial common information mask (PCI-mask) to provide a fine-grained characterization of what partial common information is shared by which subsets of the modalities. By solving a masked correlation maximization and simultaneously learning an optimal PCI-mask, we identify the latent microstructure of partial common information and leverage it in a self-attention module to selectively weight different feature representations in multi-modal data. We implement our proposed framework on the standard U-Net. Our experimental results on the Multi-modal Brain Tumor Segmentation Challenge (BraTS) datasets outperform those of state-of-the-art segmentation baselines, with validation Dice similarity coefficients of 0.920, 0.897, 0.837 for the whole tumor, tumor core, and enhancing tumor on BraTS-2020.
更多查看译文
关键词
Multi-modal learning,Image segmentation,Maximal correlation optimization,Common information
AI 理解论文
溯源树
样例
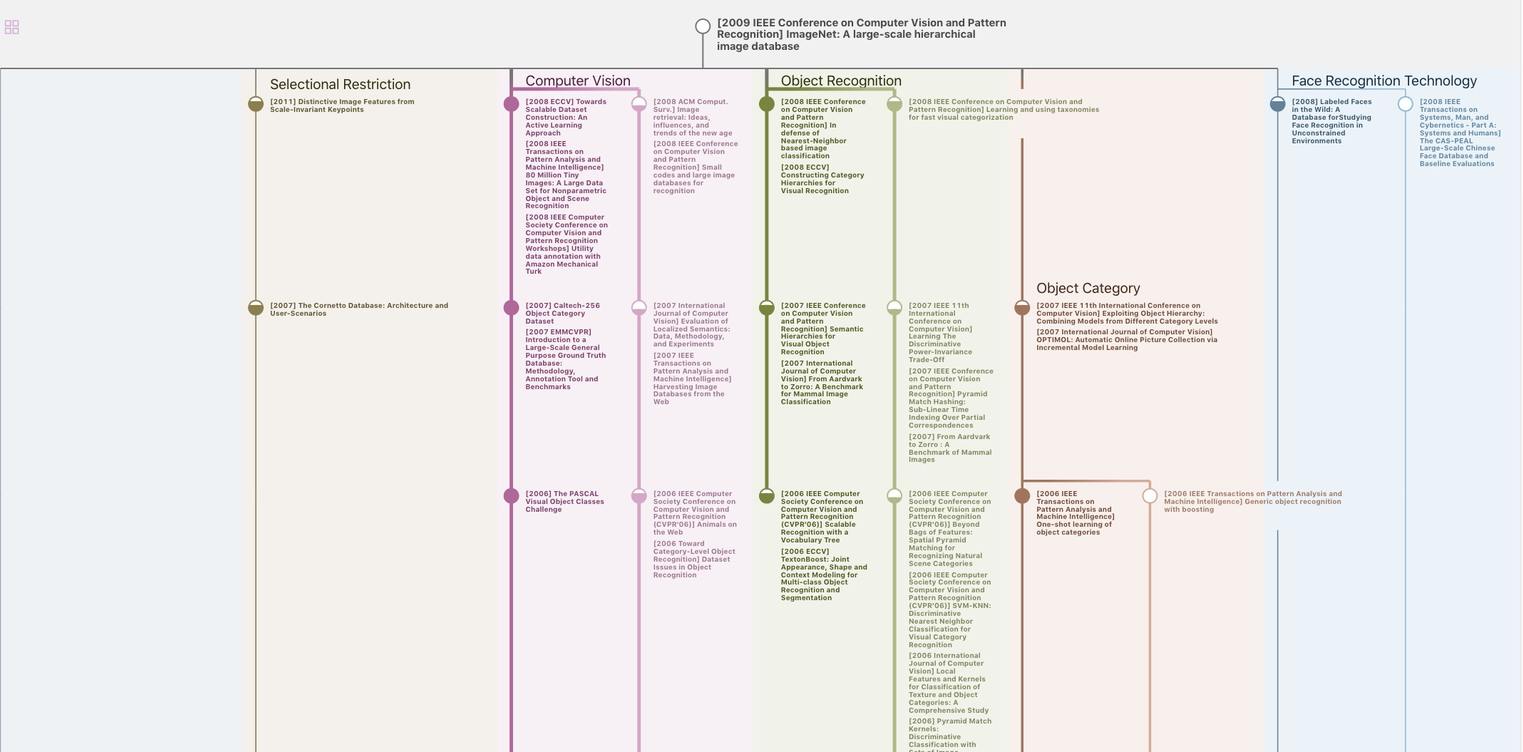
生成溯源树,研究论文发展脉络
Chat Paper
正在生成论文摘要