Model-Based Smoothing with Integrated Wiener Processes and Overlapping Splines
JOURNAL OF COMPUTATIONAL AND GRAPHICAL STATISTICS(2024)
摘要
In many applications that involve the inference of an unknown smooth function, the inference of its derivatives is also important. To make joint inferences of the function and its derivatives, a class of Gaussian processes called pth order Integrated Wiener's Process (IWP), is considered. Methods for constructing a finite element (FEM) approximation of an IWP exist but only focus on the case p = 2 and do not allow appropriate inference for derivatives. In this article, we propose an alternative FEM approximation with overlapping splines (O-spline). The O-spline approximation applies for any order p is an element of Z+, and provides consistent and efficient inference for all derivatives up to order p - 1. It is shown both theoretically and empirically that the O-spline approximation converges to the IWP as the number of knots increases. We further provide a unified and interpretable way to define priors for the smoothing parameter based on the notion of predictive standard deviation, which is invariant to the order p and the knot placement. Finally, we demonstrate the practical use of the O-spline approximation through an analysis of COVID death rates where the inference of derivative has an important interpretation in terms of the course of the pandemic. Supplementary materials for this article are available online.
更多查看译文
关键词
Approximate Bayesian inference,Derivatives inference,Gaussian process,Hierarchical model,Prior Selection,Smoothing
AI 理解论文
溯源树
样例
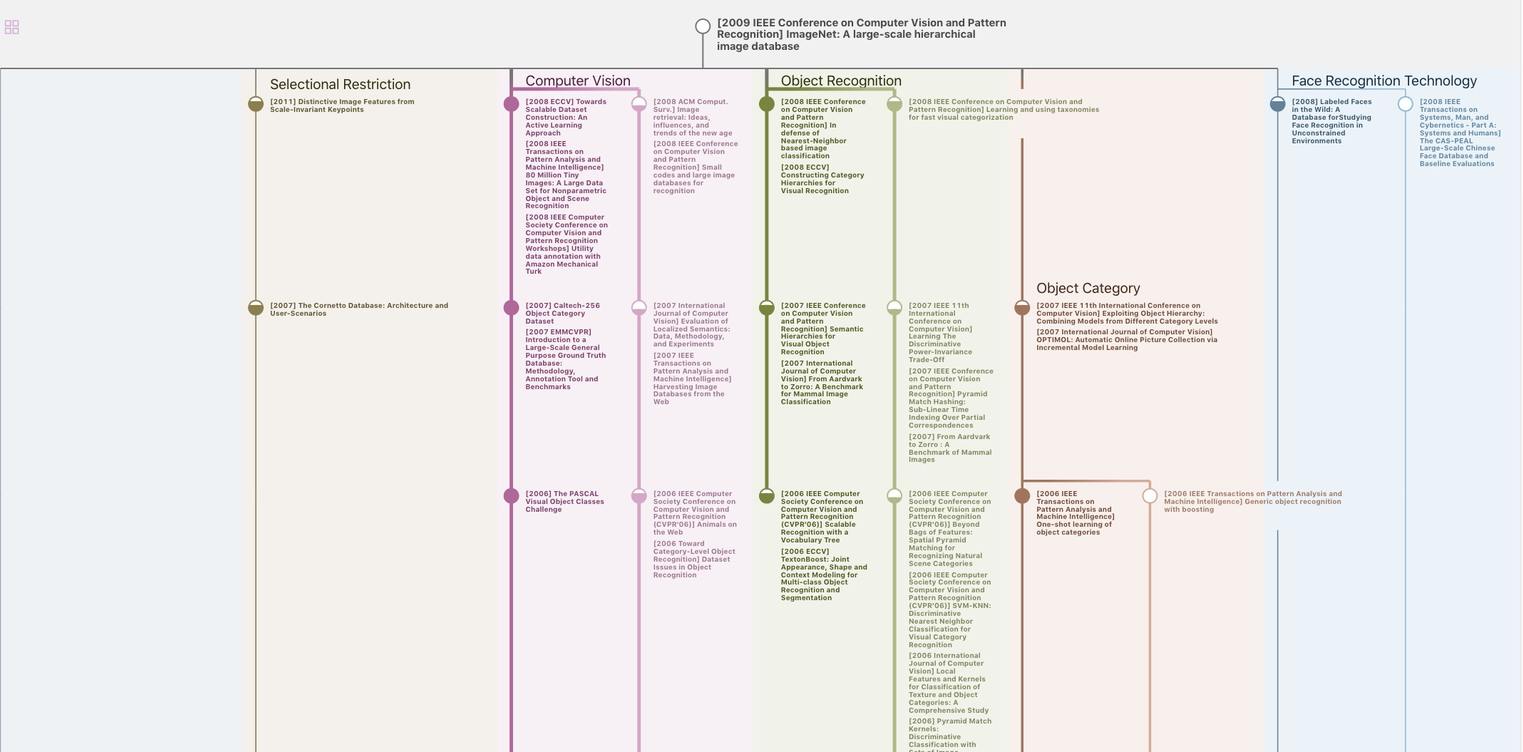
生成溯源树,研究论文发展脉络
Chat Paper
正在生成论文摘要