Revisiting Personalized Federated Learning: Robustness Against Backdoor Attacks
arxiv(2023)
摘要
In this work, besides improving prediction accuracy, we study whether personalization could bring robustness benefits to backdoor attacks. We conduct the first study of backdoor attacks in the pFL framework, testing 4 widely used backdoor attacks against 6 pFL methods on benchmark datasets FEMNIST and CIFAR-10, a total of 600 experiments. The study shows that pFL methods with partial model-sharing can significantly boost robustness against backdoor attacks. In contrast, pFL methods with full model-sharing do not show robustness. To analyze the reasons for varying robustness performances, we provide comprehensive ablation studies on different pFL methods. Based on our findings, we further propose a lightweight defense method, Simple-Tuning, which empirically improves defense performance against backdoor attacks. We believe that our work could provide both guidance for pFL application in terms of its robustness and offer valuable insights to design more robust FL methods in the future.
更多查看译文
关键词
personalized federated learning,backdoor
AI 理解论文
溯源树
样例
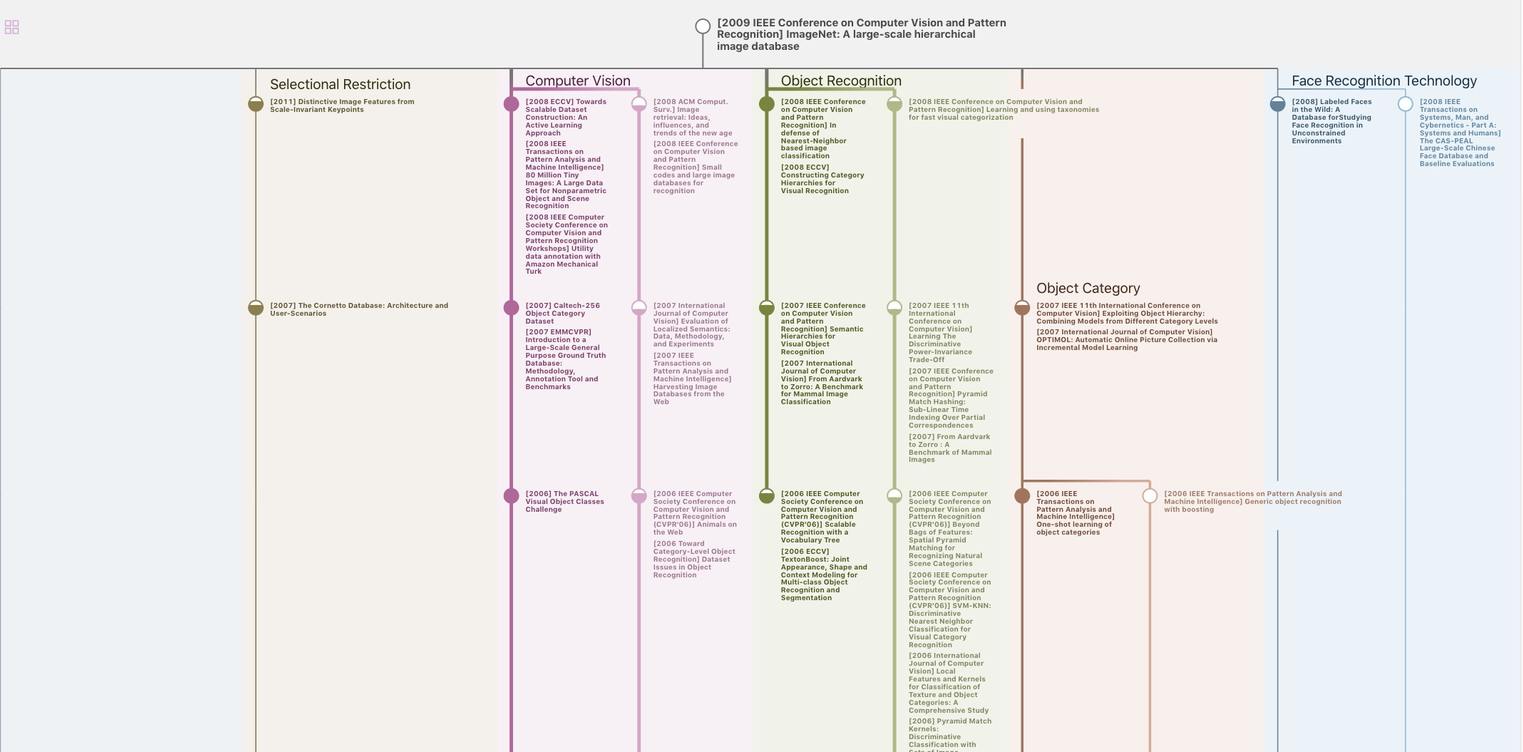
生成溯源树,研究论文发展脉络
Chat Paper
正在生成论文摘要