Follower Agnostic Methods for Stackelberg Games
arxiv(2023)
摘要
In this paper, we present an efficient algorithm to solve online Stackelberg
games, featuring multiple followers, in a follower-agnostic manner. Unlike
previous works, our approach works even when leader has no knowledge about the
followers' utility functions or strategy space. Our algorithm introduces a
unique gradient estimator, leveraging specially designed strategies to probe
followers. In a departure from traditional assumptions of optimal play, we
model followers' responses using a convergent adaptation rule, allowing for
realistic and dynamic interactions. The leader constructs the gradient
estimator solely based on observations of followers' actions. We provide both
non-asymptotic convergence rates to stationary points of the leader's objective
and demonstrate asymptotic convergence to a local Stackelberg
equilibrium. To validate the effectiveness of our algorithm, we use this
algorithm to solve the problem of incentive design on a large-scale
transportation network, showcasing its robustness even when the leader lacks
access to followers' demand.
更多查看译文
AI 理解论文
溯源树
样例
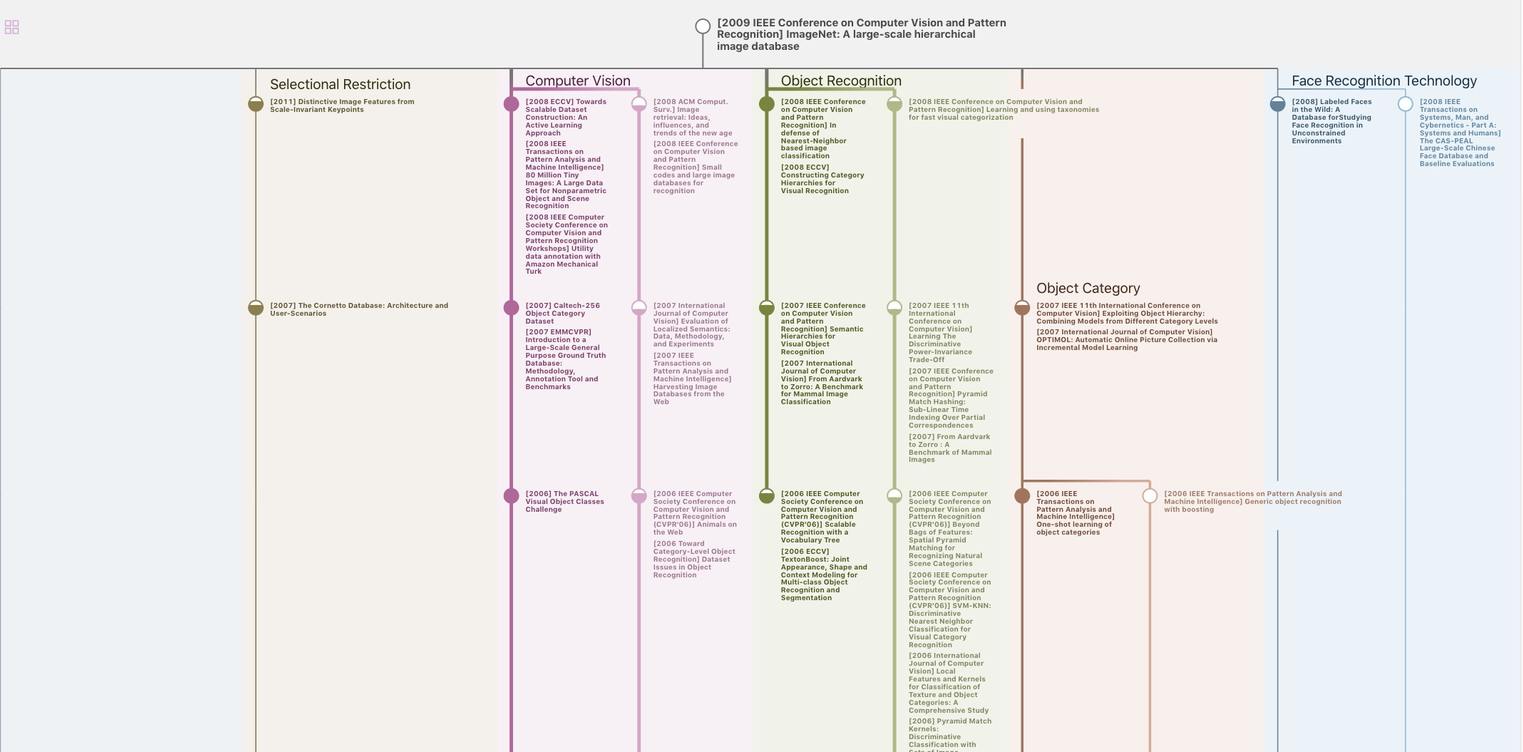
生成溯源树,研究论文发展脉络
Chat Paper
正在生成论文摘要