Statistical Verification of Traffic Systems with Expected Differential Privacy
2023 AMERICAN CONTROL CONFERENCE, ACC(2023)
摘要
Traffic systems are multi-agent cyber-physical systems whose performance is closely related to human welfare. They work in open environments and are subject to uncertainties from various sources, making their performance hard to verify by traditional model-based approaches. Alternatively, statistical model checking (SMC) can verify their performance by sequentially drawing sample data until the correctness of a performance specification can be inferred with desired statistical accuracy. This work aims to verify traffic systems with privacy, motivated by the fact that the data used may include personal information (e.g., daily itinerary) and get leaked unintendedly by observing the execution of the SMC algorithm. To formally capture data privacy in SMC, we introduce the concept of expected differential privacy (EDP), which constrains how much the algorithm execution can change in the expectation sense when data change. Accordingly, we introduce an exponential randomization mechanism for the SMC algorithm to achieve the EDP. Our case study on traffic intersections by Vissim simulation shows the high accuracy of SMC in traffic model verification without significantly sacrificing computing efficiency. The case study also shows EDP successfully bounding the algorithm outputs to guarantee privacy.
更多查看译文
关键词
statistical verification,differential privacy,traffic case study
AI 理解论文
溯源树
样例
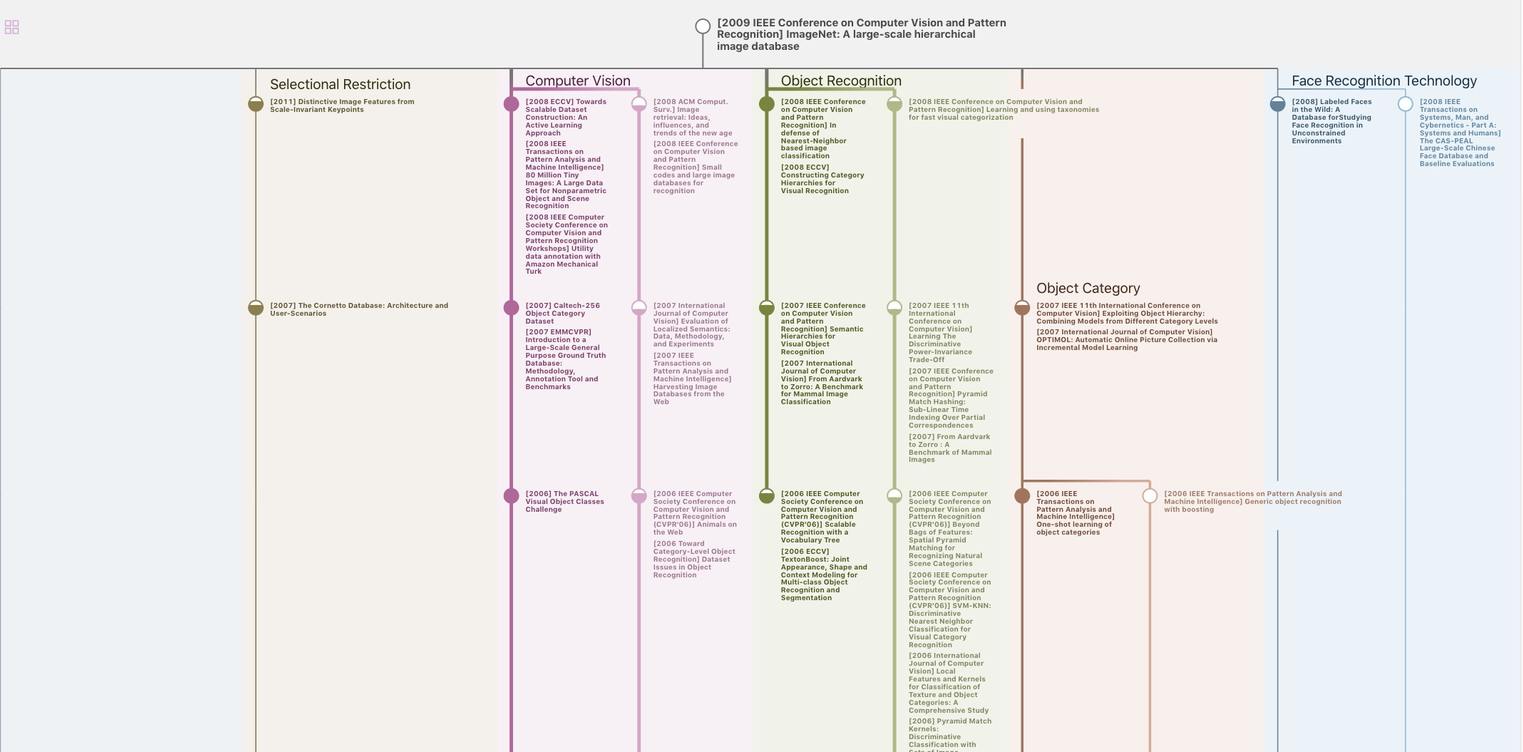
生成溯源树,研究论文发展脉络
Chat Paper
正在生成论文摘要