Augmented Learning of Heterogeneous Treatment Effects via Gradient Boosting Trees
arxiv(2023)
摘要
Heterogeneous treatment effects (HTE) based on patients' genetic or clinical factors are of significant interest to precision medicine. Simultaneously modeling HTE and corresponding main effects for randomized clinical trials with high-dimensional predictive markers is challenging. Motivated by the modified covariates approach, we propose a two-stage statistical learning procedure for estimating HTE with optimal efficiency augmentation, generalizing to arbitrary interaction model and exploiting powerful extreme gradient boosting trees (XGBoost). Target estimands for HTE are defined in the scale of mean difference for quantitative outcomes, or risk ratio for binary outcomes, which are the minimizers of specialized loss functions. The first stage is to estimate the main-effect equivalency of the baseline markers on the outcome, which is then used as an augmentation term in the second stage estimation for HTE. The proposed two-stage procedure is robust to model mis-specification of main effects and improves efficiency for estimating HTE through nonparametric function estimation, e.g., XGBoost. A permutation test is proposed for global assessment of evidence for HTE. An analysis of a genetic study in Prostate Cancer Prevention Trial led by the SWOG Cancer Research Network, is conducted to showcase the properties and the utilities of the two-stage method.
更多查看译文
关键词
heterogeneous treatment effects,boosting trees,augmented learning
AI 理解论文
溯源树
样例
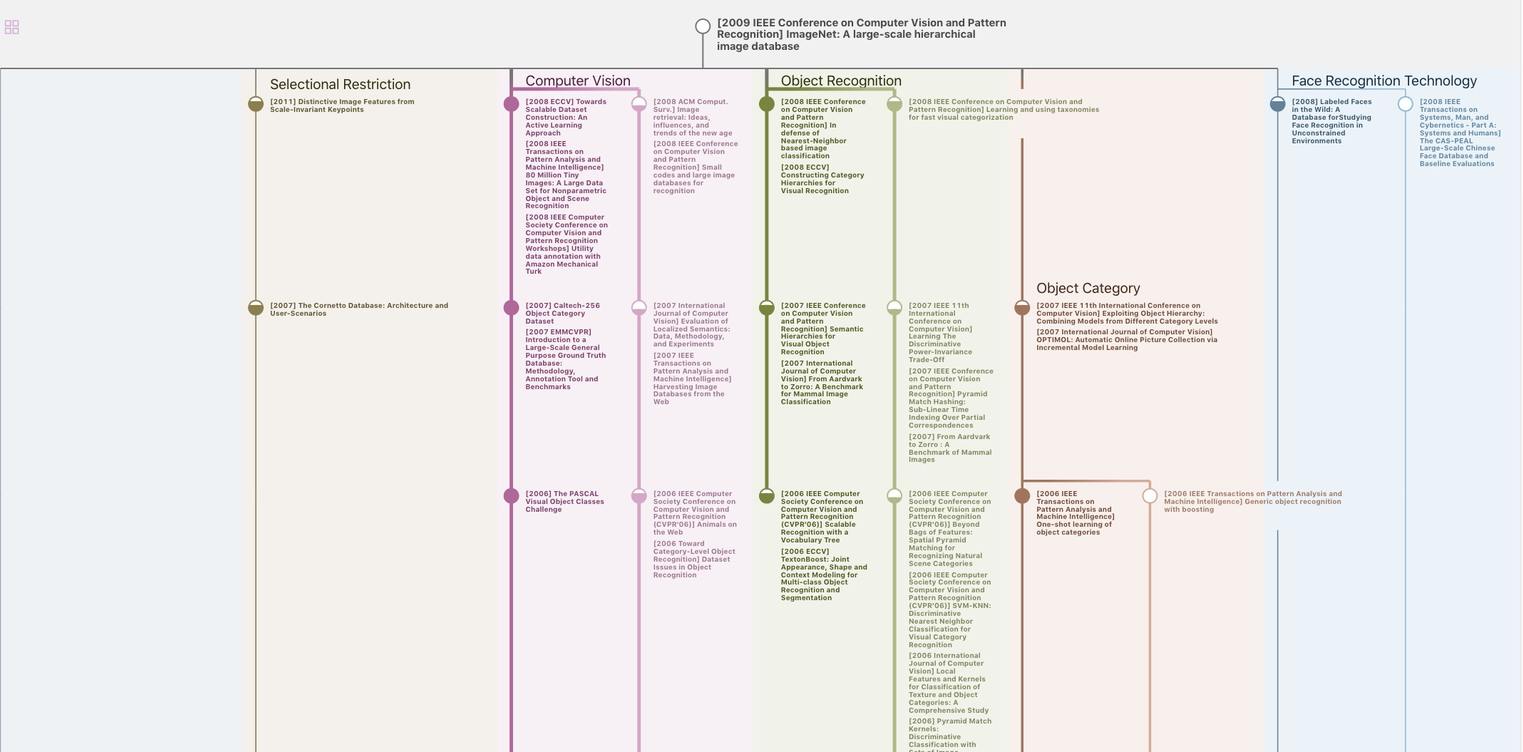
生成溯源树,研究论文发展脉络
Chat Paper
正在生成论文摘要